Boosting Healthcare LLMs Through Retrieved Context
Jordi Bayarri-Planas, Ashwin Kumar Gururajan, Dario Garcia-Gasulla
2024-09-26
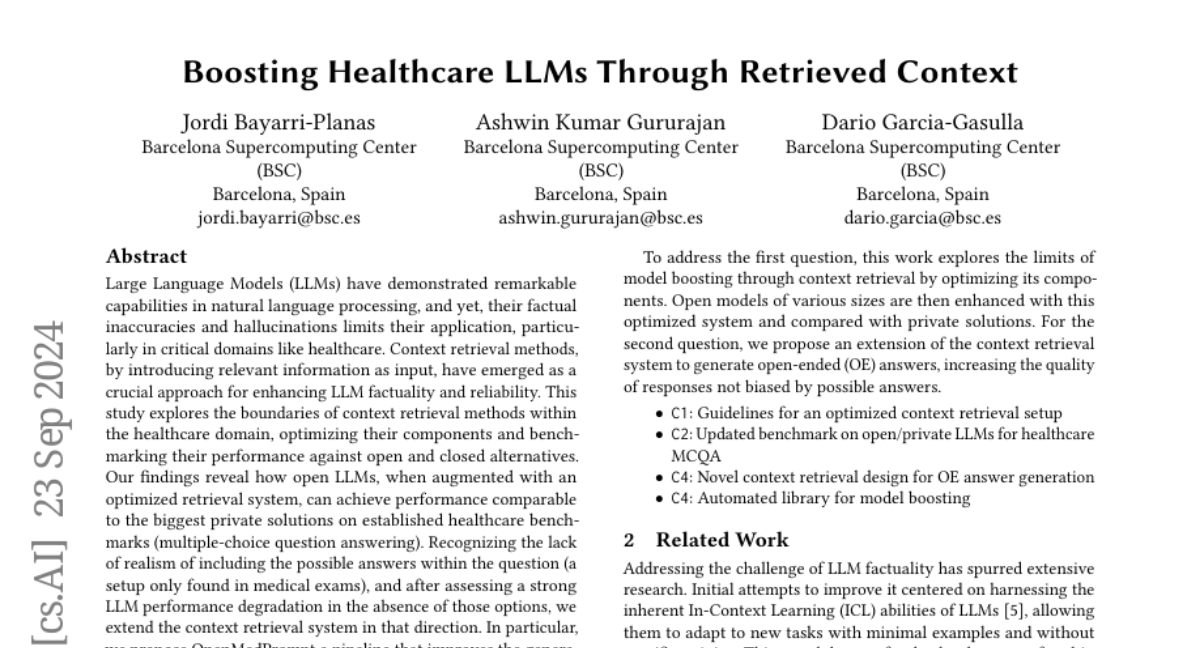
Summary
This paper discusses how to improve large language models (LLMs) for healthcare applications by using context retrieval methods. The researchers aim to enhance the accuracy and reliability of LLMs when answering medical questions.
What's the problem?
Although LLMs are powerful tools for processing language, they often provide incorrect or misleading information, especially in critical areas like healthcare. This is a significant issue because healthcare professionals rely on accurate information to make important decisions. Traditional methods of training LLMs do not always include relevant background knowledge, which can lead to errors in their responses.
What's the solution?
To tackle this problem, the researchers explored ways to enhance LLMs by providing them with additional context from reliable sources. They developed a system that retrieves relevant information and integrates it into the model's input, allowing the LLM to generate more informed and accurate answers. They also introduced a new pipeline called OpenMedPrompt, which helps the model produce better open-ended responses by considering more realistic scenarios, such as those found in medical exams.
Why it matters?
This research is important because it improves the ability of AI systems to assist in healthcare settings by increasing their accuracy and reliability. By enhancing LLMs with context retrieval methods, healthcare professionals can trust these models more when seeking information or making decisions. This advancement could lead to better patient care and more effective use of AI in medical applications.
Abstract
Large Language Models (LLMs) have demonstrated remarkable capabilities in natural language processing, and yet, their factual inaccuracies and hallucinations limits their application, particularly in critical domains like healthcare. Context retrieval methods, by introducing relevant information as input, have emerged as a crucial approach for enhancing LLM factuality and reliability. This study explores the boundaries of context retrieval methods within the healthcare domain, optimizing their components and benchmarking their performance against open and closed alternatives. Our findings reveal how open LLMs, when augmented with an optimized retrieval system, can achieve performance comparable to the biggest private solutions on established healthcare benchmarks (multiple-choice question answering). Recognizing the lack of realism of including the possible answers within the question (a setup only found in medical exams), and after assessing a strong LLM performance degradation in the absence of those options, we extend the context retrieval system in that direction. In particular, we propose OpenMedPrompt a pipeline that improves the generation of more reliable open-ended answers, moving this technology closer to practical application.