Bridging the Data Provenance Gap Across Text, Speech and Video
Shayne Longpre, Nikhil Singh, Manuel Cherep, Kushagra Tiwary, Joanna Materzynska, William Brannon, Robert Mahari, Manan Dey, Mohammed Hamdy, Nayan Saxena, Ahmad Mustafa Anis, Emad A. Alghamdi, Vu Minh Chien, Naana Obeng-Marnu, Da Yin, Kun Qian, Yizhi Li, Minnie Liang, An Dinh, Shrestha Mohanty, Deividas Mataciunas, Tobin South
2024-12-25
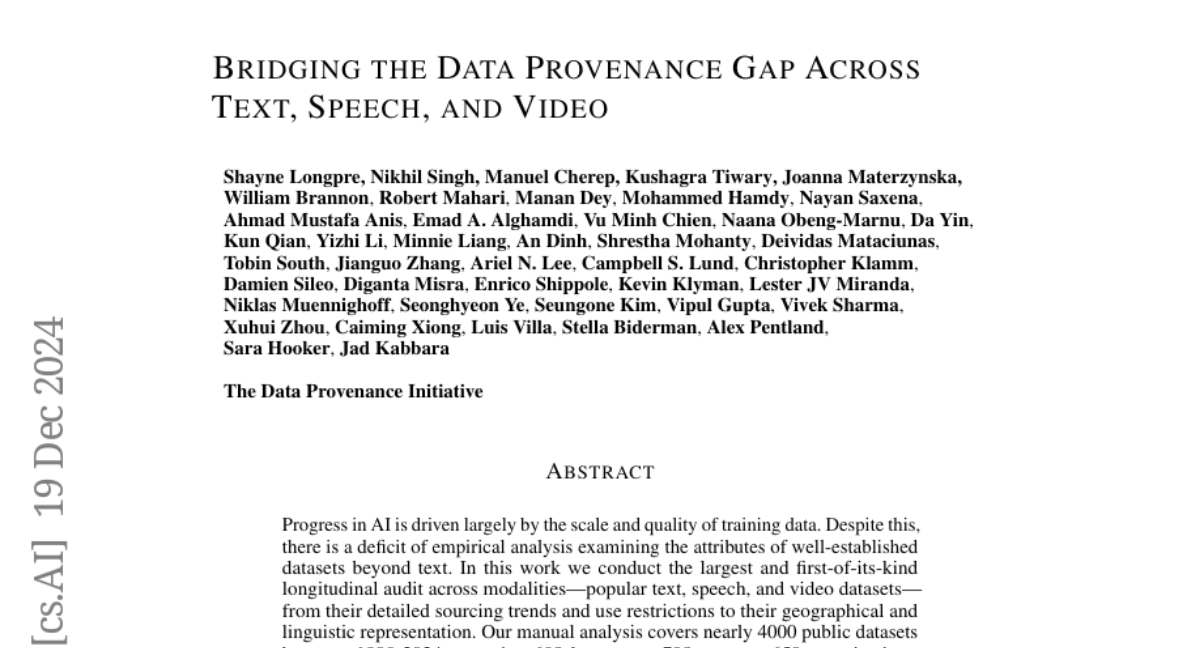
Summary
This paper talks about a comprehensive analysis of various datasets used in AI, focusing on text, speech, and video, to understand their sourcing trends and representation across different languages and regions.
What's the problem?
While AI has advanced due to large amounts of training data, there hasn't been enough detailed examination of datasets beyond just text. This lack of analysis makes it difficult to understand how these datasets are sourced and what limitations they might have, especially regarding geographical and linguistic diversity.
What's the solution?
The authors conducted a large-scale audit of nearly 4,000 public datasets from 1990 to 2024, covering text, speech, and video. They analyzed the sources of these datasets, the restrictions on their use, and their representation across different languages and countries. Their findings show that many datasets rely heavily on web-crawled data from platforms like YouTube, and they also highlight issues with licensing restrictions and a lack of improvement in geographical and multilingual representation since 2013. The authors provide their findings to help improve dataset transparency and responsible use in AI.
Why it matters?
This research is important because it sheds light on the quality and diversity of the data used to train AI systems. By understanding where this data comes from and its limitations, researchers can work towards creating more inclusive and effective AI technologies that better represent different cultures and languages.
Abstract
Progress in AI is driven largely by the scale and quality of training data. Despite this, there is a deficit of empirical analysis examining the attributes of well-established datasets beyond text. In this work we conduct the largest and first-of-its-kind longitudinal audit across modalities--popular text, speech, and video datasets--from their detailed sourcing trends and use restrictions to their geographical and linguistic representation. Our manual analysis covers nearly 4000 public datasets between 1990-2024, spanning 608 languages, 798 sources, 659 organizations, and 67 countries. We find that multimodal machine learning applications have overwhelmingly turned to web-crawled, synthetic, and social media platforms, such as YouTube, for their training sets, eclipsing all other sources since 2019. Secondly, tracing the chain of dataset derivations we find that while less than 33% of datasets are restrictively licensed, over 80% of the source content in widely-used text, speech, and video datasets, carry non-commercial restrictions. Finally, counter to the rising number of languages and geographies represented in public AI training datasets, our audit demonstrates measures of relative geographical and multilingual representation have failed to significantly improve their coverage since 2013. We believe the breadth of our audit enables us to empirically examine trends in data sourcing, restrictions, and Western-centricity at an ecosystem-level, and that visibility into these questions are essential to progress in responsible AI. As a contribution to ongoing improvements in dataset transparency and responsible use, we release our entire multimodal audit, allowing practitioners to trace data provenance across text, speech, and video.