BRIGHT: A Realistic and Challenging Benchmark for Reasoning-Intensive Retrieval
Hongjin Su, Howard Yen, Mengzhou Xia, Weijia Shi, Niklas Muennighoff, Han-yu Wang, Haisu Liu, Quan Shi, Zachary S. Siegel, Michael Tang, Ruoxi Sun, Jinsung Yoon, Sercan O. Arik, Danqi Chen, Tao Yu
2024-07-19
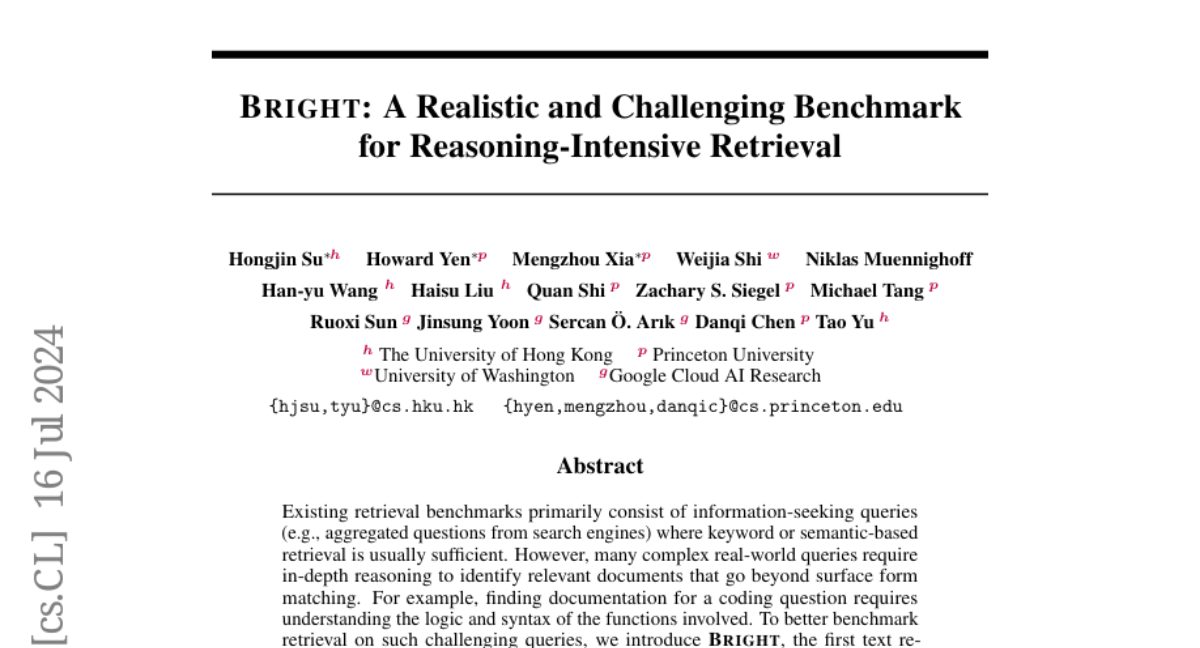
Summary
This paper introduces BRIGHT, a new benchmark designed to evaluate how well retrieval systems can handle complex queries that require deep reasoning. Unlike previous benchmarks that focus on simpler keyword searches, BRIGHT challenges models to think critically and provide relevant information from a variety of real-world topics.
What's the problem?
Most existing retrieval benchmarks are based on straightforward questions where keyword matching is usually enough. However, many real-life queries need more in-depth reasoning to find the right answers, such as understanding coding logic or complex concepts in fields like economics or psychology. This gap means that current models may not perform well when faced with these challenging queries.
What's the solution?
The authors created BRIGHT using 1,398 real-world queries from various fields, ensuring the questions require more than just surface-level matching. They tested state-of-the-art retrieval models and found that they struggled with BRIGHT, scoring much lower than expected. To improve performance, they showed that adding reasoning techniques, like Chain-of-Thought reasoning from large language models (LLMs), can help models perform better on these complex queries.
Why it matters?
This research is significant because it highlights the need for better evaluation methods for retrieval systems in realistic settings. By developing BRIGHT, the authors provide a framework for future research to improve how AI can understand and respond to complicated questions, which is crucial for applications in education, customer support, and any field where accurate information retrieval is essential.
Abstract
Existing retrieval benchmarks primarily consist of information-seeking queries (e.g., aggregated questions from search engines) where keyword or semantic-based retrieval is usually sufficient. However, many complex real-world queries require in-depth reasoning to identify relevant documents that go beyond surface form matching. For example, finding documentation for a coding question requires understanding the logic and syntax of the functions involved. To better benchmark retrieval on such challenging queries, we introduce BRIGHT, the first text retrieval benchmark that requires intensive reasoning to retrieve relevant documents. BRIGHT is constructed from the 1,398 real-world queries collected from diverse domains (such as economics, psychology, robotics, software engineering, earth sciences, etc.), sourced from naturally occurring or carefully curated human data. Extensive evaluation reveals that even state-of-the-art retrieval models perform poorly on BRIGHT. The leading model on the MTEB leaderboard [38 ], which achieves a score of 59.0 nDCG@10,2 produces a score of nDCG@10 of 18.0 on BRIGHT. We further demonstrate that augmenting queries with Chain-of-Thought reasoning generated by large language models (LLMs) improves performance by up to 12.2 points. Moreover, BRIGHT is robust against data leakage during pretraining of the benchmarked models as we validate by showing similar performance even when documents from the benchmark are included in the training data. We believe that BRIGHT paves the way for future research on retrieval systems in more realistic and challenging settings. Our code and data are available at https://brightbenchmark.github.io.