C3PO: Critical-Layer, Core-Expert, Collaborative Pathway Optimization for Test-Time Expert Re-Mixing
Zhongyang Li, Ziyue Li, Tianyi Zhou
2025-04-11
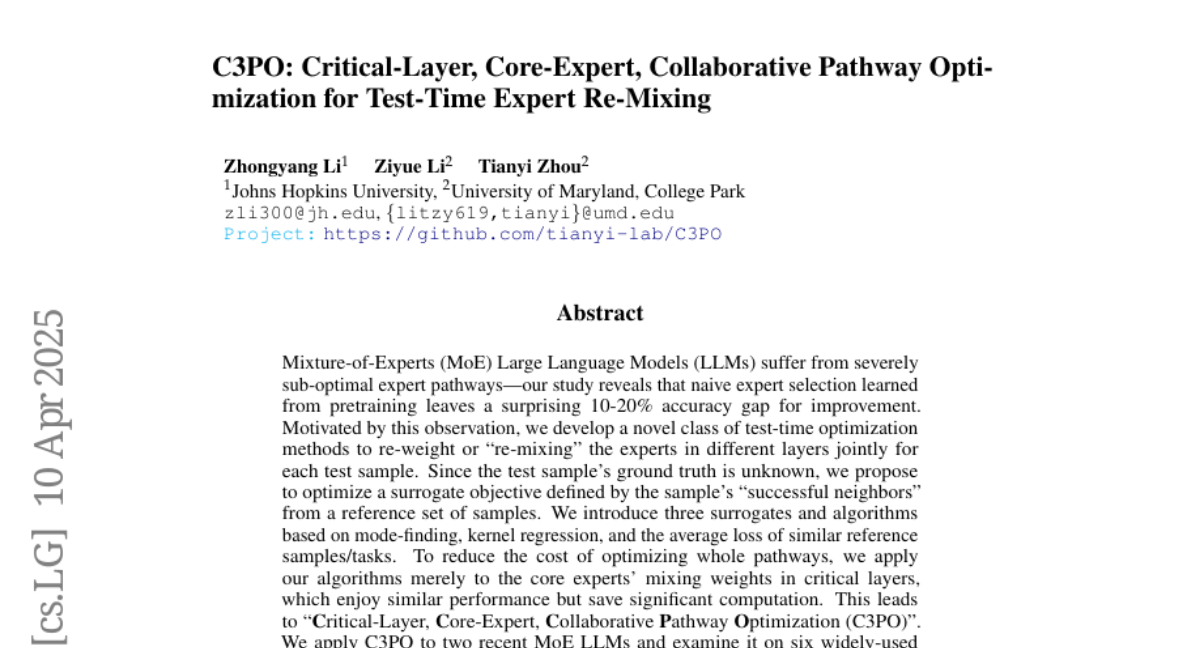
Summary
This paper introduces C3PO, a new method to make large language models that use Mixture-of-Experts (MoE) smarter and more accurate by improving how they pick which expert parts of the model to use when answering questions. Instead of just using the expert selection learned during training, C3PO changes how experts are chosen for each new question, even after the model is already trained.
What's the problem?
The main problem is that MoE language models often don't pick the best combination of their expert parts when solving new problems, which means they don't perform as well as they could. The way they usually select which experts to use is set during training and isn't flexible enough, leaving a noticeable gap in how accurate these models can be.
What's the solution?
C3PO solves this by introducing a way to adjust and re-mix the experts in the most important layers of the model every time a new question is asked. Since the correct answer isn't known ahead of time, C3PO looks at similar problems it has seen before and uses their results to guide the selection. The method uses smart algorithms to find the best mix without needing to change the whole model, making it both effective and efficient.
Why it matters?
This matters because C3PO makes language models much more accurate and efficient, allowing smaller models to beat much larger ones on many tests. It also shows a new way to keep improving AI models even after they are trained, helping them adapt better to new types of questions and making advanced AI more accessible and useful.
Abstract
Mixture-of-Experts (MoE) Large Language Models (LLMs) suffer from severely sub-optimal expert pathways-our study reveals that naive expert selection learned from pretraining leaves a surprising 10-20% accuracy gap for improvement. Motivated by this observation, we develop a novel class of test-time optimization methods to re-weight or "re-mixing" the experts in different layers jointly for each test sample. Since the test sample's ground truth is unknown, we propose to optimize a surrogate objective defined by the sample's "successful neighbors" from a reference set of samples. We introduce three surrogates and algorithms based on mode-finding, kernel regression, and the average loss of similar reference samples/tasks. To reduce the cost of optimizing whole pathways, we apply our algorithms merely to the core experts' mixing weights in critical layers, which enjoy similar performance but save significant computation. This leads to "Critical-Layer, Core-Expert, Collaborative Pathway Optimization (C3PO)". We apply C3PO to two recent MoE LLMs and examine it on six widely-used benchmarks. It consistently improves the base model by 7-15% in accuracy and outperforms widely used test-time learning baselines, e.g., in-context learning and prompt/prefix tuning, by a large margin. Moreover, C3PO enables MoE LLMs with 1-3B active parameters to outperform LLMs of 7-9B parameters, hence improving MoE's advantages on efficiency. Our thorough ablation study further sheds novel insights on achieving test-time improvement on MoE.