Cambrian-1: A Fully Open, Vision-Centric Exploration of Multimodal LLMs
Shengbang Tong, Ellis Brown, Penghao Wu, Sanghyun Woo, Manoj Middepogu, Sai Charitha Akula, Jihan Yang, Shusheng Yang, Adithya Iyer, Xichen Pan, Austin Wang, Rob Fergus, Yann LeCun, Saining Xie
2024-06-25
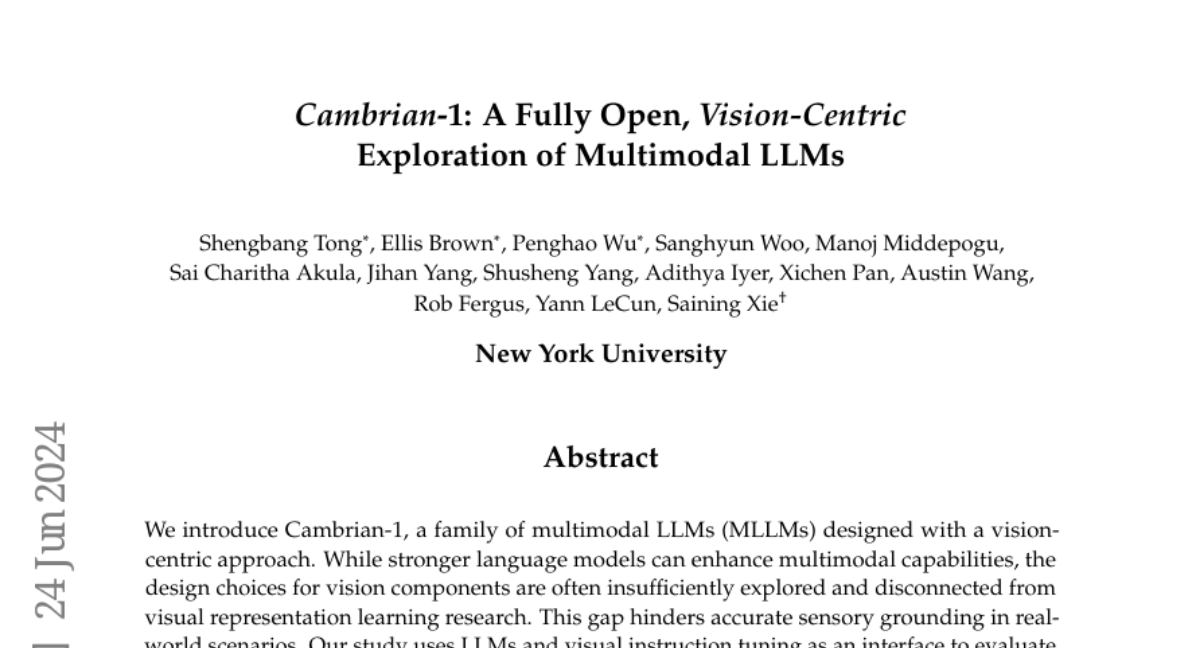
Summary
This paper introduces Cambrian-1, a new family of multimodal large language models (MLLMs) that focuses on improving how these models understand and use visual information. It aims to bridge the gap between language processing and visual representation learning.
What's the problem?
Current multimodal models often do not effectively integrate visual components with language processing. This lack of connection makes it difficult for these models to accurately understand and interpret real-world scenarios, which limits their effectiveness in tasks that require both visual and textual understanding.
What's the solution?
The authors developed Cambrian-1 to address these issues by using a vision-centric approach. They experimented with over 20 different vision encoders and introduced a new benchmark called CV-Bench to evaluate model performance. They also created the Spatial Vision Aggregator (SVA), which helps combine visual features with language models more efficiently. Additionally, they focused on gathering high-quality training data, ensuring a balanced representation of different sources.
Why it matters?
This research is significant because it enhances the capabilities of multimodal models, making them better at tasks that involve both images and text. By providing open access to their methods, tools, and datasets, the authors hope to inspire further developments in the field of AI, leading to more advanced systems that can understand and interact with the world in a more human-like way.
Abstract
We introduce Cambrian-1, a family of multimodal LLMs (MLLMs) designed with a vision-centric approach. While stronger language models can enhance multimodal capabilities, the design choices for vision components are often insufficiently explored and disconnected from visual representation learning research. This gap hinders accurate sensory grounding in real-world scenarios. Our study uses LLMs and visual instruction tuning as an interface to evaluate various visual representations, offering new insights into different models and architectures -- self-supervised, strongly supervised, or combinations thereof -- based on experiments with over 20 vision encoders. We critically examine existing MLLM benchmarks, addressing the difficulties involved in consolidating and interpreting results from various tasks, and introduce a new vision-centric benchmark, CV-Bench. To further improve visual grounding, we propose the Spatial Vision Aggregator (SVA), a dynamic and spatially-aware connector that integrates high-resolution vision features with LLMs while reducing the number of tokens. Additionally, we discuss the curation of high-quality visual instruction-tuning data from publicly available sources, emphasizing the importance of data source balancing and distribution ratio. Collectively, Cambrian-1 not only achieves state-of-the-art performance but also serves as a comprehensive, open cookbook for instruction-tuned MLLMs. We provide model weights, code, supporting tools, datasets, and detailed instruction-tuning and evaluation recipes. We hope our release will inspire and accelerate advancements in multimodal systems and visual representation learning.