Can Knowledge Editing Really Correct Hallucinations?
Baixiang Huang, Canyu Chen, Xiongxiao Xu, Ali Payani, Kai Shu
2024-10-25
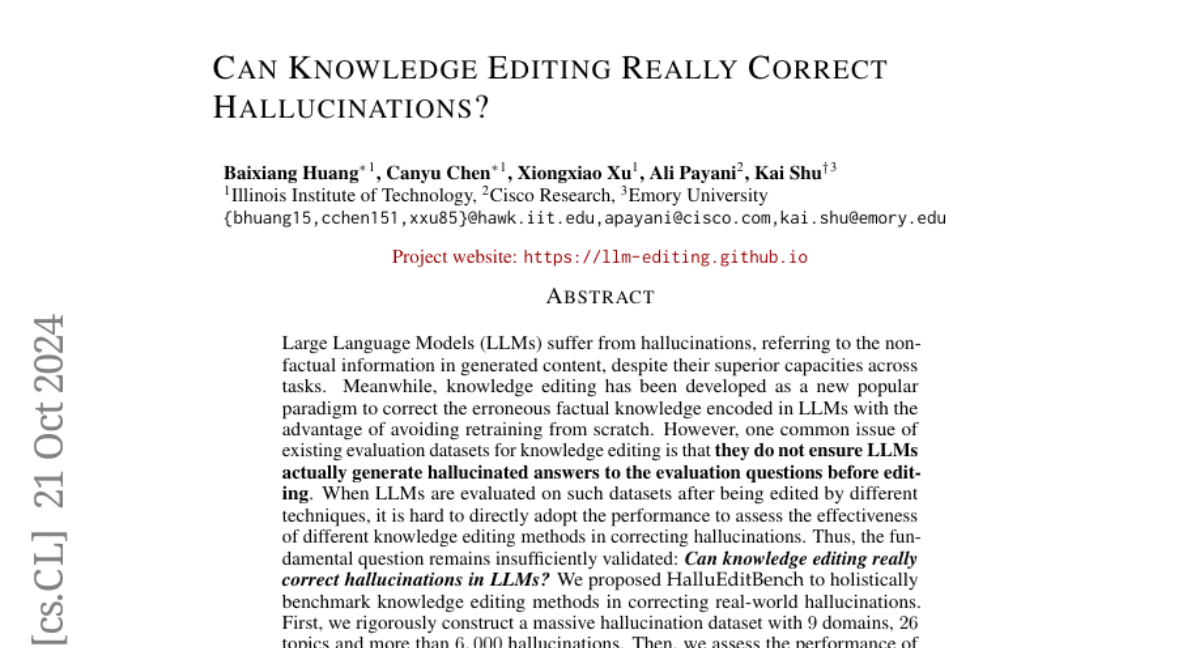
Summary
This paper explores how knowledge editing can be used to fix inaccuracies, or 'hallucinations', in large language models (LLMs) without needing to retrain them from scratch.
What's the problem?
Large language models often generate incorrect or misleading information, known as hallucinations. These errors can occur because the models are trained on a mix of high-quality and low-quality data, making it hard to ensure they always produce accurate answers. Additionally, existing methods for evaluating how well knowledge editing works often don't confirm whether the models actually produced hallucinated answers before being edited.
What's the solution?
The authors introduce HalluEditBench, a new framework designed to thoroughly test knowledge editing methods specifically for correcting real-world hallucinations. They created a large dataset containing over 6,000 examples of hallucinations across different topics. This allows for a comprehensive evaluation of various knowledge editing techniques based on multiple criteria, such as how effective and robust they are in fixing these inaccuracies.
Why it matters?
This research is significant because it provides a clearer way to assess whether knowledge editing can effectively correct errors in language models. By improving our understanding of how to fix these issues, we can enhance the reliability of AI systems that rely on LLMs, making them more trustworthy for users in fields like education, healthcare, and customer service.
Abstract
Large Language Models (LLMs) suffer from hallucinations, referring to the non-factual information in generated content, despite their superior capacities across tasks. Meanwhile, knowledge editing has been developed as a new popular paradigm to correct the erroneous factual knowledge encoded in LLMs with the advantage of avoiding retraining from scratch. However, one common issue of existing evaluation datasets for knowledge editing is that they do not ensure LLMs actually generate hallucinated answers to the evaluation questions before editing. When LLMs are evaluated on such datasets after being edited by different techniques, it is hard to directly adopt the performance to assess the effectiveness of different knowledge editing methods in correcting hallucinations. Thus, the fundamental question remains insufficiently validated: Can knowledge editing really correct hallucinations in LLMs? We proposed HalluEditBench to holistically benchmark knowledge editing methods in correcting real-world hallucinations. First, we rigorously construct a massive hallucination dataset with 9 domains, 26 topics and more than 6,000 hallucinations. Then, we assess the performance of knowledge editing methods in a holistic way on five dimensions including Efficacy, Generalization, Portability, Locality, and Robustness. Through HalluEditBench, we have provided new insights into the potentials and limitations of different knowledge editing methods in correcting hallucinations, which could inspire future improvements and facilitate the progress in the field of knowledge editing.