Can OOD Object Detectors Learn from Foundation Models?
Jiahui Liu, Xin Wen, Shizhen Zhao, Yingxian Chen, Xiaojuan Qi
2024-09-13
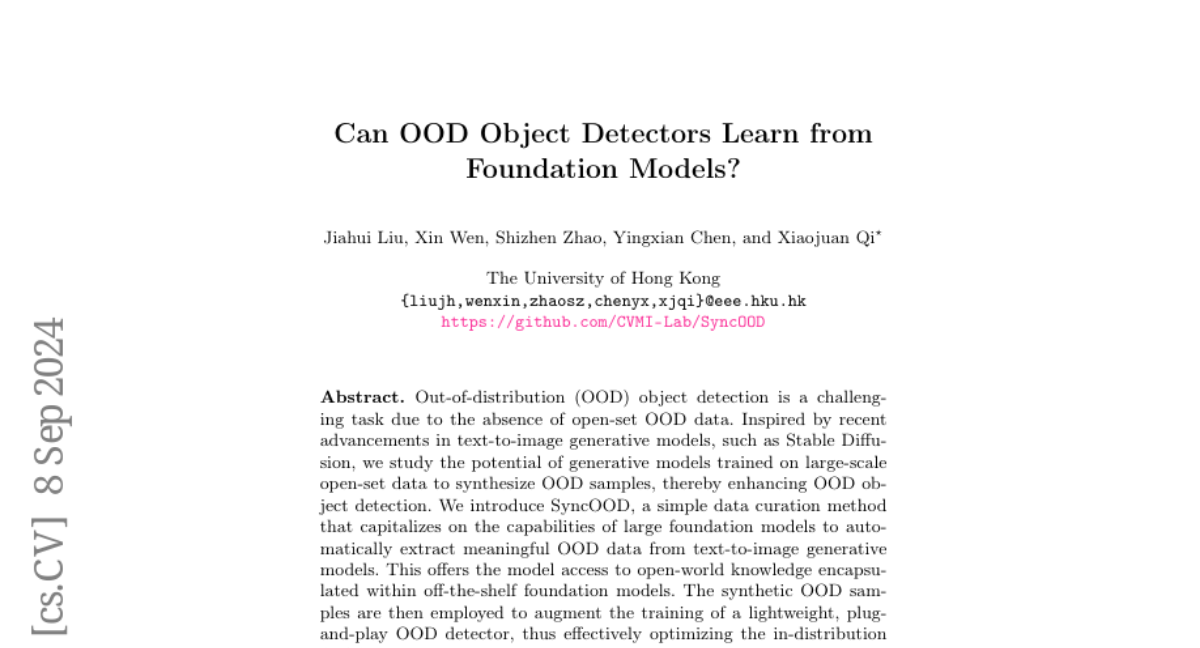
Summary
This paper discusses SyncOOD, a new method for improving out-of-distribution (OOD) object detection by using generative models to create synthetic data from text descriptions.
What's the problem?
Detecting objects that are not part of the training data (OOD) is difficult because there isn't enough available data to train models effectively. Traditional methods often struggle with this task due to the lack of diverse examples and the complexity of the objects involved.
What's the solution?
SyncOOD leverages large generative models, like those used in text-to-image generation, to automatically create synthetic OOD samples. This method extracts meaningful data from these models, allowing for the creation of new images that contain novel objects. The synthetic data is then used to train a lightweight OOD detector, improving its ability to distinguish between known and unknown objects. The approach has been shown to significantly outperform existing methods while using minimal synthetic data.
Why it matters?
This research is important because it enhances the capability of AI systems to recognize and respond to unfamiliar objects in various applications, such as surveillance, robotics, and autonomous vehicles. By improving OOD detection, it can lead to safer and more reliable AI technologies.
Abstract
Out-of-distribution (OOD) object detection is a challenging task due to the absence of open-set OOD data. Inspired by recent advancements in text-to-image generative models, such as Stable Diffusion, we study the potential of generative models trained on large-scale open-set data to synthesize OOD samples, thereby enhancing OOD object detection. We introduce SyncOOD, a simple data curation method that capitalizes on the capabilities of large foundation models to automatically extract meaningful OOD data from text-to-image generative models. This offers the model access to open-world knowledge encapsulated within off-the-shelf foundation models. The synthetic OOD samples are then employed to augment the training of a lightweight, plug-and-play OOD detector, thus effectively optimizing the in-distribution (ID)/OOD decision boundaries. Extensive experiments across multiple benchmarks demonstrate that SyncOOD significantly outperforms existing methods, establishing new state-of-the-art performance with minimal synthetic data usage.