Can sparse autoencoders be used to decompose and interpret steering vectors?
Harry Mayne, Yushi Yang, Adam Mahdi
2024-11-14
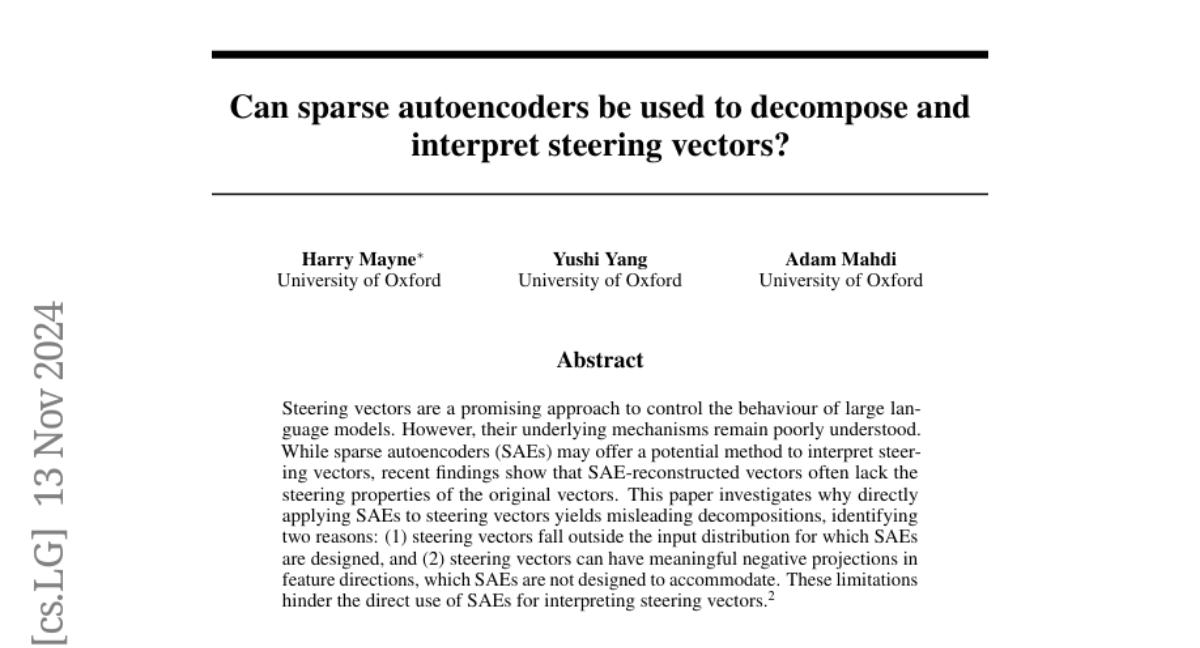
Summary
This paper explores the use of sparse autoencoders (SAEs) to understand and break down steering vectors, which are tools used to control how large language models behave.
What's the problem?
Steering vectors can help guide the outputs of language models, but their inner workings are not well understood. When researchers tried to use SAEs to analyze these vectors, they found that the reconstructed vectors often did not retain the important steering properties of the originals. This raises concerns about the effectiveness of using SAEs for this purpose.
What's the solution?
The authors investigated why applying SAEs directly to steering vectors can lead to misleading results. They identified two main issues: first, steering vectors often don't fit the type of data SAEs are designed to work with; second, steering vectors can include negative values in certain directions that SAEs cannot handle. These problems make it difficult to accurately interpret steering vectors using SAEs.
Why it matters?
Understanding how to effectively use steering vectors is crucial for improving the control we have over language models. By identifying the limitations of using SAEs for this task, this research helps pave the way for developing better methods to analyze and utilize steering vectors, ultimately leading to more reliable AI systems.
Abstract
Steering vectors are a promising approach to control the behaviour of large language models. However, their underlying mechanisms remain poorly understood. While sparse autoencoders (SAEs) may offer a potential method to interpret steering vectors, recent findings show that SAE-reconstructed vectors often lack the steering properties of the original vectors. This paper investigates why directly applying SAEs to steering vectors yields misleading decompositions, identifying two reasons: (1) steering vectors fall outside the input distribution for which SAEs are designed, and (2) steering vectors can have meaningful negative projections in feature directions, which SAEs are not designed to accommodate. These limitations hinder the direct use of SAEs for interpreting steering vectors.