CANVAS: Commonsense-Aware Navigation System for Intuitive Human-Robot Interaction
Suhwan Choi, Yongjun Cho, Minchan Kim, Jaeyoon Jung, Myunchul Joe, Yubeen Park, Minseo Kim, Sungwoong Kim, Sungjae Lee, Hwiseong Park, Jiwan Chung, Youngjae Yu
2024-10-07
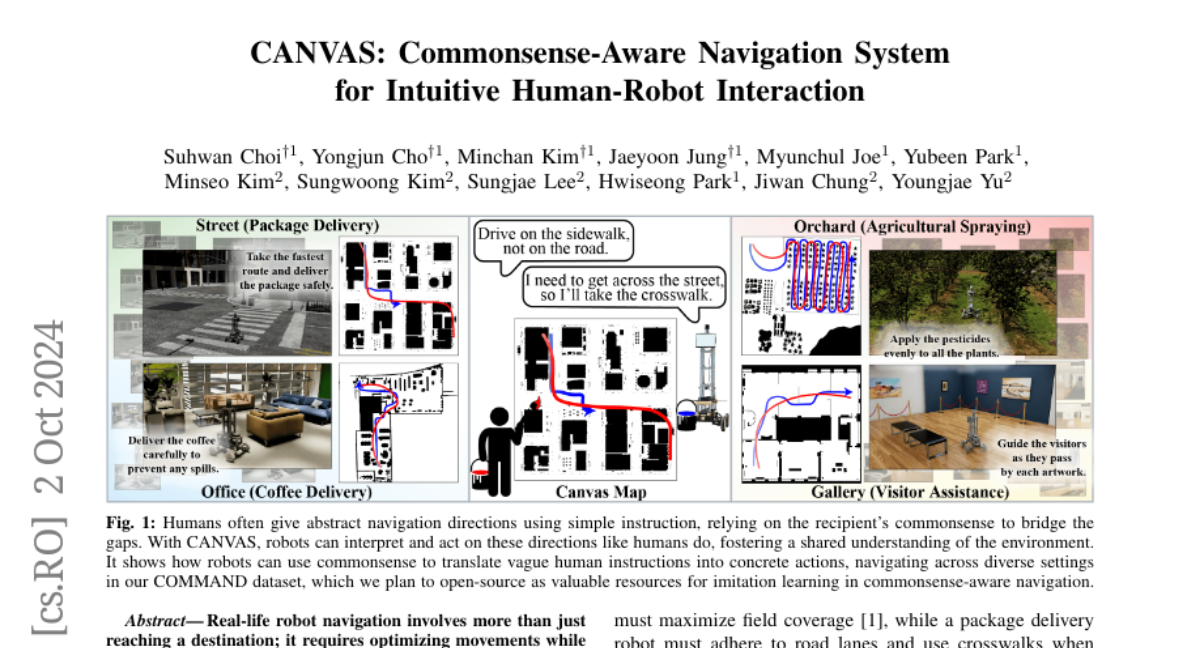
Summary
This paper introduces CANVAS, a new navigation system that helps robots understand and follow human commands more intuitively by combining visual and verbal instructions.
What's the problem?
Robots often struggle to navigate effectively based on vague human instructions, such as simple commands or rough sketches. These instructions can be unclear or incomplete, making it hard for robots to understand exactly what is expected of them. Without a shared understanding of navigation concepts, robots may not perform as intended.
What's the solution?
To solve this problem, the authors developed CANVAS, which allows robots to learn from human behavior through imitation. It uses a dataset called COMMAND, which contains over 48 hours of human navigation data to train the robots. CANVAS combines visual cues and verbal commands to create a commonsense-aware navigation system that can interpret abstract instructions better. The system has been tested in various environments and has shown significant improvements in navigating with noisy or unclear instructions compared to traditional systems.
Why it matters?
This research is important because it enhances how robots interact with humans, making them more effective in real-world scenarios. By improving a robot's ability to understand and follow human commands intuitively, CANVAS could lead to better applications in areas like personal assistance, delivery services, and even in hazardous environments where precise navigation is crucial.
Abstract
Real-life robot navigation involves more than just reaching a destination; it requires optimizing movements while addressing scenario-specific goals. An intuitive way for humans to express these goals is through abstract cues like verbal commands or rough sketches. Such human guidance may lack details or be noisy. Nonetheless, we expect robots to navigate as intended. For robots to interpret and execute these abstract instructions in line with human expectations, they must share a common understanding of basic navigation concepts with humans. To this end, we introduce CANVAS, a novel framework that combines visual and linguistic instructions for commonsense-aware navigation. Its success is driven by imitation learning, enabling the robot to learn from human navigation behavior. We present COMMAND, a comprehensive dataset with human-annotated navigation results, spanning over 48 hours and 219 km, designed to train commonsense-aware navigation systems in simulated environments. Our experiments show that CANVAS outperforms the strong rule-based system ROS NavStack across all environments, demonstrating superior performance with noisy instructions. Notably, in the orchard environment, where ROS NavStack records a 0% total success rate, CANVAS achieves a total success rate of 67%. CANVAS also closely aligns with human demonstrations and commonsense constraints, even in unseen environments. Furthermore, real-world deployment of CANVAS showcases impressive Sim2Real transfer with a total success rate of 69%, highlighting the potential of learning from human demonstrations in simulated environments for real-world applications.