CapArena: Benchmarking and Analyzing Detailed Image Captioning in the LLM Era
Kanzhi Cheng, Wenpo Song, Jiaxin Fan, Zheng Ma, Qiushi Sun, Fangzhi Xu, Chenyang Yan, Nuo Chen, Jianbing Zhang, Jiajun Chen
2025-03-19
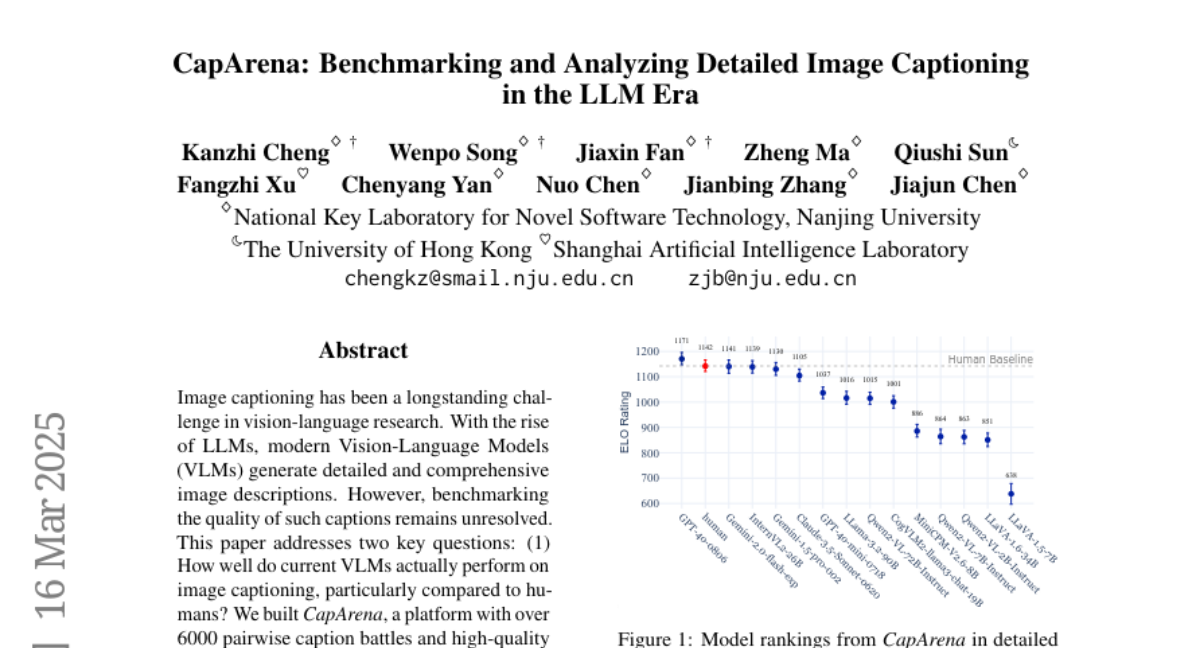
Summary
This paper introduces CapArena, a tool to test how well AI models can describe images in detail, similar to how humans do.
What's the problem?
It's hard to know if AI-generated image descriptions are actually good because there wasn't a reliable way to compare them, especially against human-written descriptions.
What's the solution?
The researchers built CapArena, a platform where AI-generated captions are compared against each other and human-written captions. This helps figure out which models are best at detailed image descriptions. They also created a way to automatically score the captions.
Why it matters?
This work matters because it helps improve AI's ability to understand and describe images, which is important for many applications like helping visually impaired people or making search engines better.
Abstract
Image captioning has been a longstanding challenge in vision-language research. With the rise of LLMs, modern Vision-Language Models (VLMs) generate detailed and comprehensive image descriptions. However, benchmarking the quality of such captions remains unresolved. This paper addresses two key questions: (1) How well do current VLMs actually perform on image captioning, particularly compared to humans? We built CapArena, a platform with over 6000 pairwise caption battles and high-quality human preference votes. Our arena-style evaluation marks a milestone, showing that leading models like GPT-4o achieve or even surpass human performance, while most open-source models lag behind. (2) Can automated metrics reliably assess detailed caption quality? Using human annotations from CapArena, we evaluate traditional and recent captioning metrics, as well as VLM-as-a-Judge. Our analysis reveals that while some metrics (e.g., METEOR) show decent caption-level agreement with humans, their systematic biases lead to inconsistencies in model ranking. In contrast, VLM-as-a-Judge demonstrates robust discernment at both the caption and model levels. Building on these insights, we release CapArena-Auto, an accurate and efficient automated benchmark for detailed captioning, achieving 94.3% correlation with human rankings at just $4 per test. Data and resources will be open-sourced at https://caparena.github.io.