CBT-Bench: Evaluating Large Language Models on Assisting Cognitive Behavior Therapy
Mian Zhang, Xianjun Yang, Xinlu Zhang, Travis Labrum, Jamie C. Chiu, Shaun M. Eack, Fei Fang, William Yang Wang, Zhiyu Zoey Chen
2024-10-22
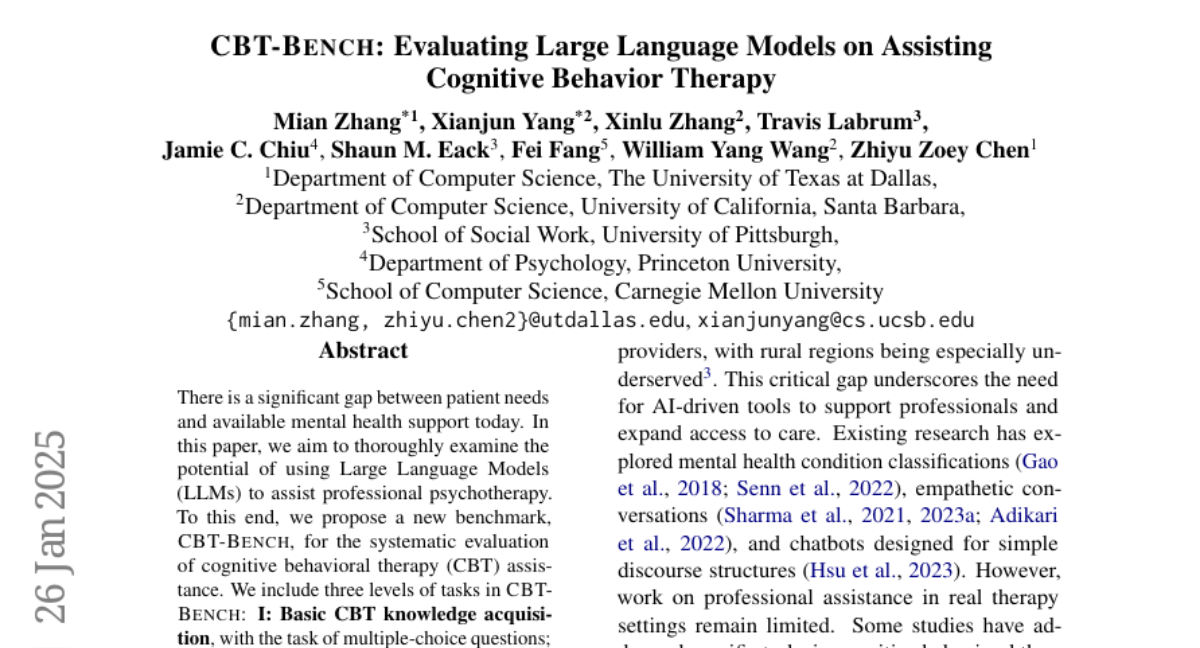
Summary
This paper presents Agent-to-Sim (ATS), a new framework that learns how 3D agents, like humans and animals, behave by analyzing long videos recorded in a single environment.
What's the problem?
Understanding and simulating the behavior of agents in 3D environments is challenging because traditional methods often rely on complex setups with multiple cameras or special tracking markers. This can be invasive and impractical for studying natural behaviors over time. There is a need for a more straightforward way to learn these behaviors from regular video recordings without extra equipment.
What's the solution?
To tackle this issue, the authors developed ATS, which learns from casual videos taken over long periods (like a month). They created a method that tracks both the agent's movements and the camera's position to build a detailed 4D representation of the environment. This allows them to capture how agents behave in different situations. The framework then trains a model that can simulate these behaviors based on the video data, enabling the transfer of real-world actions into a virtual simulation.
Why it matters?
This research is significant because it allows for realistic simulations of agent behavior using easily obtainable video data. By making it possible to study and replicate how pets or people interact in their environments, this framework can be applied in various fields such as robotics, gaming, and virtual reality. It opens up new possibilities for creating interactive experiences that closely mimic real-life behavior.
Abstract
There is a significant gap between patient needs and available mental health support today. In this paper, we aim to thoroughly examine the potential of using Large Language Models (LLMs) to assist professional psychotherapy. To this end, we propose a new benchmark, CBT-BENCH, for the systematic evaluation of cognitive behavioral therapy (CBT) assistance. We include three levels of tasks in CBT-BENCH: I: Basic CBT knowledge acquisition, with the task of multiple-choice questions; II: Cognitive model understanding, with the tasks of cognitive distortion classification, primary core belief classification, and fine-grained core belief classification; III: Therapeutic response generation, with the task of generating responses to patient speech in CBT therapy sessions. These tasks encompass key aspects of CBT that could potentially be enhanced through AI assistance, while also outlining a hierarchy of capability requirements, ranging from basic knowledge recitation to engaging in real therapeutic conversations. We evaluated representative LLMs on our benchmark. Experimental results indicate that while LLMs perform well in reciting CBT knowledge, they fall short in complex real-world scenarios requiring deep analysis of patients' cognitive structures and generating effective responses, suggesting potential future work.