CFG-Zero*: Improved Classifier-Free Guidance for Flow Matching Models
Weichen Fan, Amber Yijia Zheng, Raymond A. Yeh, Ziwei Liu
2025-03-25
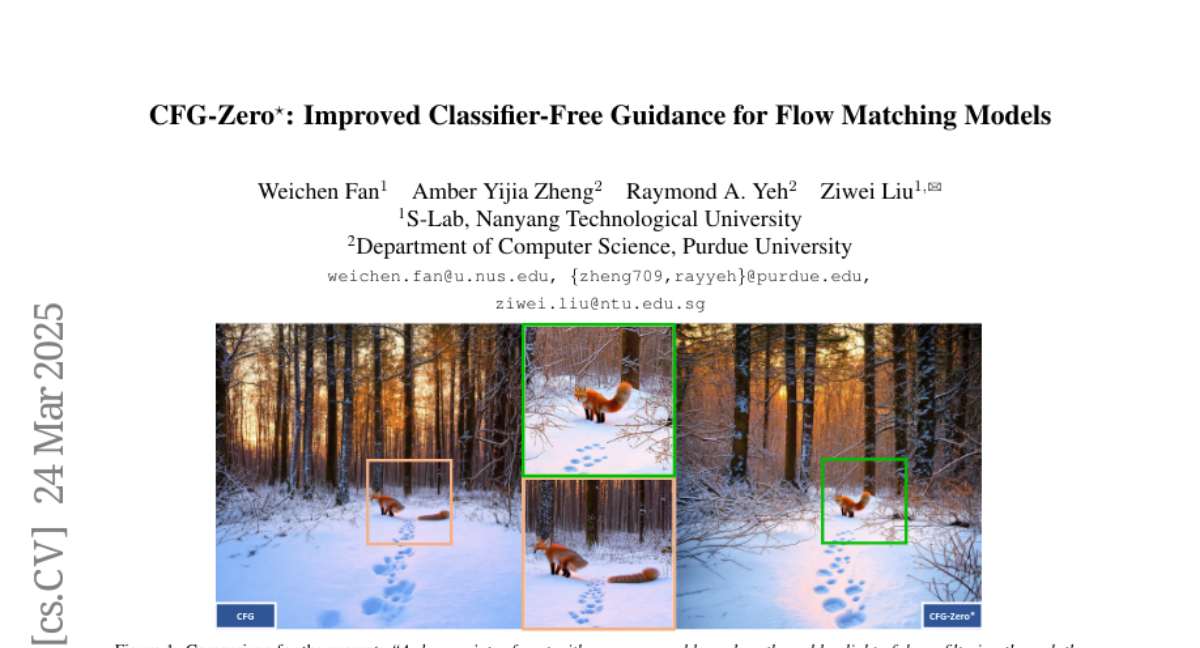
Summary
This paper is about improving how AI creates images using a technique called Classifier-Free Guidance (CFG).
What's the problem?
CFG, which helps AI generate better images, can sometimes lead the AI in the wrong direction, especially early in the training process when the AI is still learning.
What's the solution?
The researchers developed a new version of CFG called CFG-Zero* that optimizes the scaling factor and zeros out the first few steps of the process, which helps the AI stay on the right track.
Why it matters?
This work matters because it can lead to AI image generators that produce higher-quality and more controllable images.
Abstract
Classifier-Free Guidance (CFG) is a widely adopted technique in diffusion/flow models to improve image fidelity and controllability. In this work, we first analytically study the effect of CFG on flow matching models trained on Gaussian mixtures where the ground-truth flow can be derived. We observe that in the early stages of training, when the flow estimation is inaccurate, CFG directs samples toward incorrect trajectories. Building on this observation, we propose CFG-Zero*, an improved CFG with two contributions: (a) optimized scale, where a scalar is optimized to correct for the inaccuracies in the estimated velocity, hence the * in the name; and (b) zero-init, which involves zeroing out the first few steps of the ODE solver. Experiments on both text-to-image (Lumina-Next, Stable Diffusion 3, and Flux) and text-to-video (Wan-2.1) generation demonstrate that CFG-Zero* consistently outperforms CFG, highlighting its effectiveness in guiding Flow Matching models. (Code is available at github.com/WeichenFan/CFG-Zero-star)