ChartGemma: Visual Instruction-tuning for Chart Reasoning in the Wild
Ahmed Masry, Megh Thakkar, Aayush Bajaj, Aaryaman Kartha, Enamul Hoque, Shafiq Joty
2024-07-08
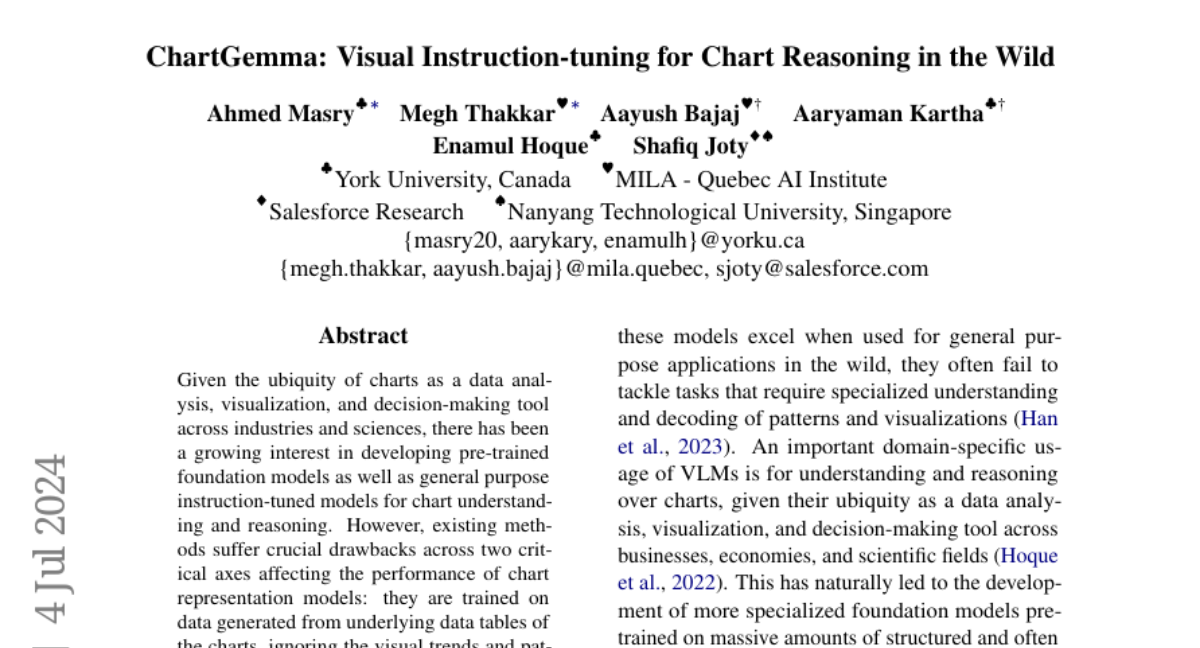
Summary
This paper talks about ChartGemma, a new AI model designed to improve how computers understand and analyze charts. It learns directly from chart images instead of relying on data tables, which helps it recognize visual patterns and trends more effectively.
What's the problem?
The main problem with existing chart understanding models is that they often use data generated from underlying tables, which means they miss important visual details present in the actual charts. Additionally, these models are built using weakly aligned vision-language techniques, making them less effective when faced with real-world charts that vary in style and complexity.
What's the solution?
To solve these issues, the authors developed ChartGemma, which is trained using instruction-tuning data created directly from chart images. This allows the model to learn both high-level trends (like overall patterns) and low-level details (like specific shapes and colors) from a wide range of charts. By doing this, ChartGemma can better understand and reason about charts, leading to improved performance on tasks like summarizing data, answering questions, and fact-checking.
Why it matters?
This research is important because it enhances the ability of AI systems to process and interpret charts, which are widely used in many fields for data analysis and decision-making. By improving how models understand visual information, ChartGemma can help users make better decisions based on complex data presented in charts, ultimately leading to more informed outcomes in various industries.
Abstract
Given the ubiquity of charts as a data analysis, visualization, and decision-making tool across industries and sciences, there has been a growing interest in developing pre-trained foundation models as well as general purpose instruction-tuned models for chart understanding and reasoning. However, existing methods suffer crucial drawbacks across two critical axes affecting the performance of chart representation models: they are trained on data generated from underlying data tables of the charts, ignoring the visual trends and patterns in chart images, and use weakly aligned vision-language backbone models for domain-specific training, limiting their generalizability when encountering charts in the wild. We address these important drawbacks and introduce ChartGemma, a novel chart understanding and reasoning model developed over PaliGemma. Rather than relying on underlying data tables, ChartGemma is trained on instruction-tuning data generated directly from chart images, thus capturing both high-level trends and low-level visual information from a diverse set of charts. Our simple approach achieves state-of-the-art results across 5 benchmarks spanning chart summarization, question answering, and fact-checking, and our elaborate qualitative studies on real-world charts show that ChartGemma generates more realistic and factually correct summaries compared to its contemporaries. We release the code, model checkpoints, dataset, and demos at https://github.com/vis-nlp/ChartGemma.