ChartMimic: Evaluating LMM's Cross-Modal Reasoning Capability via Chart-to-Code Generation
Chufan Shi, Cheng Yang, Yaxin Liu, Bo Shui, Junjie Wang, Mohan Jing, Linran Xu, Xinyu Zhu, Siheng Li, Yuxiang Zhang, Gongye Liu, Xiaomei Nie, Deng Cai, Yujiu Yang
2024-06-17
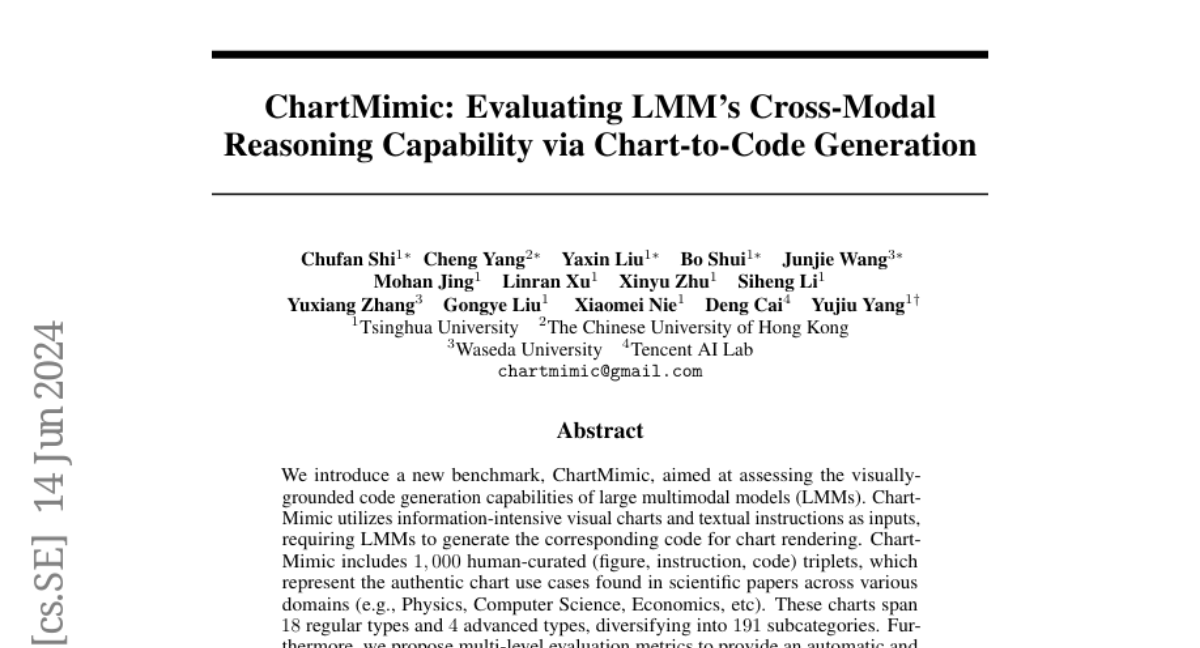
Summary
This paper introduces ChartMimic, a new benchmark designed to evaluate how well large multimodal models (LMMs) can generate code from visual charts and text instructions. It focuses on testing the models' ability to understand and create accurate representations of data.
What's the problem?
Many existing benchmarks for code generation do not effectively assess how well AI models can handle complex tasks that involve both visual information (like charts) and textual instructions. This is important because generating code that accurately reflects the information in charts is crucial for applications in fields such as science and economics. Current models often struggle with this task, leading to inaccuracies in the generated code.
What's the solution?
To address this issue, the authors created ChartMimic, which consists of 1,000 carefully curated triplets of figures, instructions, and corresponding code. These triplets represent real-world examples found in scientific papers across various domains. The benchmark includes a wide variety of chart types and uses multi-level evaluation metrics to thoroughly assess how well the models perform in generating code from these charts. This approach emphasizes the models' abilities in visual understanding, code generation, and cross-modal reasoning.
Why it matters?
This research is significant because it provides a more comprehensive way to evaluate AI models that need to work with both visual and textual data. By highlighting the challenges faced by current models and offering a structured way to assess their performance, ChartMimic aims to inspire improvements in AI development. This could lead to advancements in artificial intelligence that better understand and generate complex data representations, ultimately contributing to the goal of achieving artificial general intelligence.
Abstract
We introduce a new benchmark, ChartMimic, aimed at assessing the visually-grounded code generation capabilities of large multimodal models (LMMs). ChartMimic utilizes information-intensive visual charts and textual instructions as inputs, requiring LMMs to generate the corresponding code for chart rendering. ChartMimic includes 1,000 human-curated (figure, instruction, code) triplets, which represent the authentic chart use cases found in scientific papers across various domains(e.g., Physics, Computer Science, Economics, etc). These charts span 18 regular types and 4 advanced types, diversifying into 191 subcategories. Furthermore, we propose multi-level evaluation metrics to provide an automatic and thorough assessment of the output code and the rendered charts. Unlike existing code generation benchmarks, ChartMimic places emphasis on evaluating LMMs' capacity to harmonize a blend of cognitive capabilities, encompassing visual understanding, code generation, and cross-modal reasoning. The evaluation of 3 proprietary models and 11 open-weight models highlights the substantial challenges posed by ChartMimic. Even the advanced GPT-4V, Claude-3-opus only achieve an average score of 73.2 and 53.7, respectively, indicating significant room for improvement. We anticipate that ChartMimic will inspire the development of LMMs, advancing the pursuit of artificial general intelligence.