ChatGLM: A Family of Large Language Models from GLM-130B to GLM-4 All Tools
Team GLM, Aohan Zeng, Bin Xu, Bowen Wang, Chenhui Zhang, Da Yin, Diego Rojas, Guanyu Feng, Hanlin Zhao, Hanyu Lai, Hao Yu, Hongning Wang, Jiadai Sun, Jiajie Zhang, Jiale Cheng, Jiayi Gui, Jie Tang, Jing Zhang, Juanzi Li, Lei Zhao, Lindong Wu, Lucen Zhong
2024-06-19
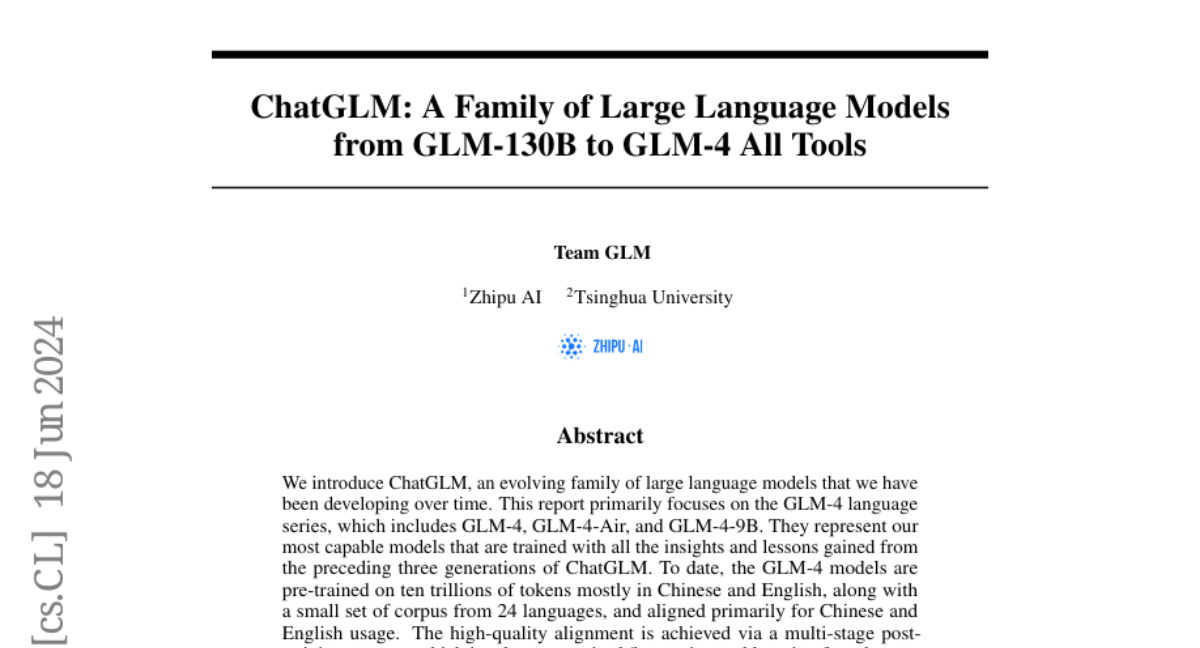
Summary
This paper introduces ChatGLM, a series of advanced large language models, focusing on the latest GLM-4 models. These models are designed to understand and generate text in multiple languages, primarily Chinese and English, and have been developed using lessons learned from previous versions.
What's the problem?
As language models have become more popular, there is a need for models that can perform well across different tasks and languages. However, many existing models are limited in their ability to understand context and user intent, especially in languages other than English. This can make it challenging for users to get accurate and relevant responses from these AI systems.
What's the solution?
The authors developed the GLM-4 series, which includes GLM-4, GLM-4-Air, and GLM-4-9B. These models are trained on a massive dataset of ten trillion tokens from various languages, focusing mainly on Chinese and English. They use a multi-stage training process that involves fine-tuning with human feedback to improve performance. The GLM-4 models are designed to follow instructions effectively and can autonomously decide which tools to use for complex tasks, such as web browsing or solving math problems. This makes them versatile and capable of handling a wide range of applications.
Why it matters?
This research is significant because it pushes the boundaries of what language models can do, making them more accessible and useful for people who speak different languages. By offering open-source versions of these models, the authors aim to encourage innovation and development in the AI community. The success of the GLM-4 models could lead to better AI tools for education, customer service, content creation, and more, ultimately enhancing how we interact with technology.
Abstract
We introduce ChatGLM, an evolving family of large language models that we have been developing over time. This report primarily focuses on the GLM-4 language series, which includes GLM-4, GLM-4-Air, and GLM-4-9B. They represent our most capable models that are trained with all the insights and lessons gained from the preceding three generations of ChatGLM. To date, the GLM-4 models are pre-trained on ten trillions of tokens mostly in Chinese and English, along with a small set of corpus from 24 languages, and aligned primarily for Chinese and English usage. The high-quality alignment is achieved via a multi-stage post-training process, which involves supervised fine-tuning and learning from human feedback. Evaluations show that GLM-4 1) closely rivals or outperforms GPT-4 in terms of general metrics such as MMLU, GSM8K, MATH, BBH, GPQA, and HumanEval, 2) gets close to GPT-4-Turbo in instruction following as measured by IFEval, 3) matches GPT-4 Turbo (128K) and Claude 3 for long context tasks, and 4) outperforms GPT-4 in Chinese alignments as measured by AlignBench. The GLM-4 All Tools model is further aligned to understand user intent and autonomously decide when and which tool(s) touse -- including web browser, Python interpreter, text-to-image model, and user-defined functions -- to effectively complete complex tasks. In practical applications, it matches and even surpasses GPT-4 All Tools in tasks like accessing online information via web browsing and solving math problems using Python interpreter. Over the course, we have open-sourced a series of models, including ChatGLM-6B (three generations), GLM-4-9B (128K, 1M), GLM-4V-9B, WebGLM, and CodeGeeX, attracting over 10 million downloads on Hugging face in the year 2023 alone. The open models can be accessed through https://github.com/THUDM and https://huggingface.co/THUDM.