ChroKnowledge: Unveiling Chronological Knowledge of Language Models in Multiple Domains
Yein Park, Chanwoong Yoon, Jungwoo Park, Donghyeon Lee, Minbyul Jeong, Jaewoo Kang
2024-10-17
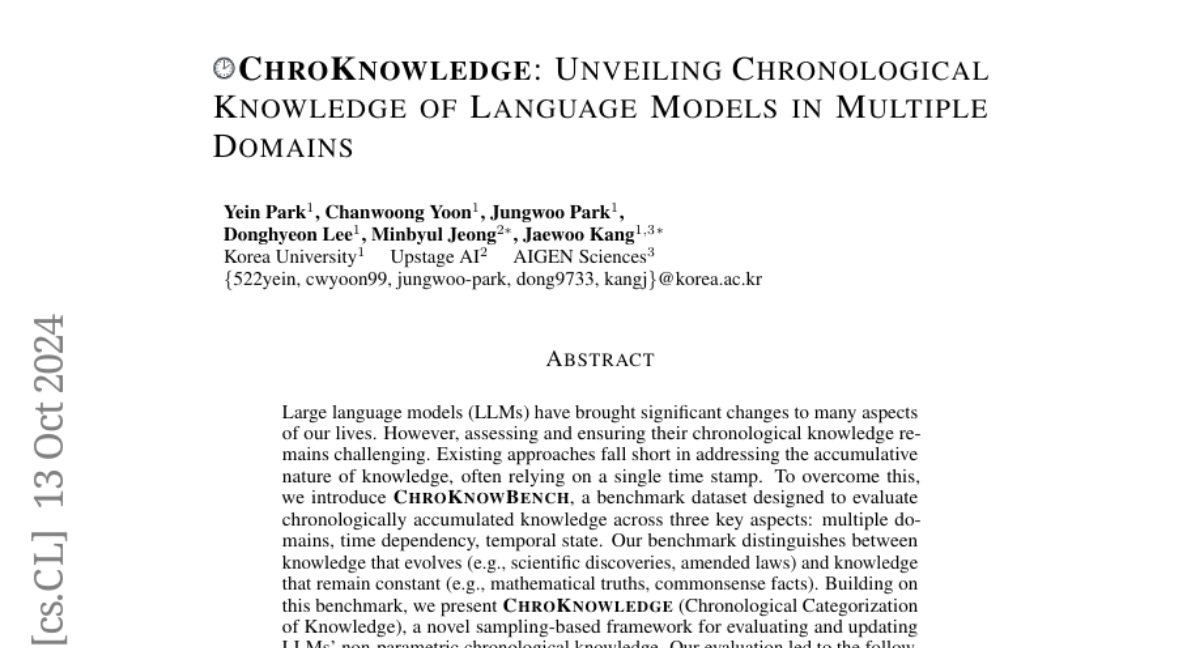
Summary
This paper introduces ChroKnowledge, a framework designed to evaluate and improve the chronological knowledge of large language models (LLMs) across different subjects and time periods.
What's the problem?
Large language models have a significant impact on many areas, but it can be challenging to assess how well they understand information over time. Current methods often use a single timestamp for evaluation, which doesn’t capture how knowledge accumulates or changes. This makes it difficult to ensure that these models accurately reflect updated information, especially in fields where facts can evolve.
What's the solution?
To tackle this issue, the authors created ChroKnowBench, a benchmark dataset that evaluates LLMs based on their ability to handle knowledge that changes over time. They categorize knowledge into two types: evolving knowledge (like scientific discoveries) and constant knowledge (like basic math facts). The framework includes a method called ChroKnowPrompt, which helps elicit and update the model's chronological knowledge by guiding it through relevant time periods step-by-step. Their experiments show that this approach significantly improves the model's ability to recall and update information in both biomedical and general domains.
Why it matters?
This research is important because it enhances our understanding of how LLMs can maintain accurate and up-to-date knowledge over time. By developing methods to evaluate and refine the chronological knowledge of these models, ChroKnowledge can improve the reliability of AI applications in various fields, ensuring they provide accurate information that reflects the latest developments.
Abstract
Large language models (LLMs) have significantly impacted many aspects of our lives. However, assessing and ensuring their chronological knowledge remains challenging. Existing approaches fall short in addressing the accumulative nature of knowledge, often relying on a single time stamp. To overcome this, we introduce ChroKnowBench, a benchmark dataset designed to evaluate chronologically accumulated knowledge across three key aspects: multiple domains, time dependency, temporal state. Our benchmark distinguishes between knowledge that evolves (e.g., scientific discoveries, amended laws) and knowledge that remain constant (e.g., mathematical truths, commonsense facts). Building on this benchmark, we present ChroKnowledge (Chronological Categorization of Knowledge), a novel sampling-based framework for evaluating and updating LLMs' non-parametric chronological knowledge. Our evaluation shows: (1) The ability of eliciting temporal knowledge varies depending on the data format that model was trained on. (2) LLMs partially recall knowledge or show a cut-off at temporal boundaries rather than recalling all aspects of knowledge correctly. Thus, we apply our ChroKnowPrompt, an in-depth prompting to elicit chronological knowledge by traversing step-by-step through the surrounding time spans. We observe that our framework successfully updates the overall knowledge across the entire timeline in both the biomedical domain (+11.9%) and the general domain (+2.8%), demonstrating its effectiveness in refining temporal knowledge. This non-parametric approach also enables knowledge updates not only in open-source models but also in proprietary LLMs, ensuring comprehensive applicability across model types. We perform a comprehensive analysis based on temporal characteristics of ChroKnowPrompt and validate the potential of various models to elicit intrinsic temporal knowledge through our method.