CityDreamer4D: Compositional Generative Model of Unbounded 4D Cities
Haozhe Xie, Zhaoxi Chen, Fangzhou Hong, Ziwei Liu
2025-01-16
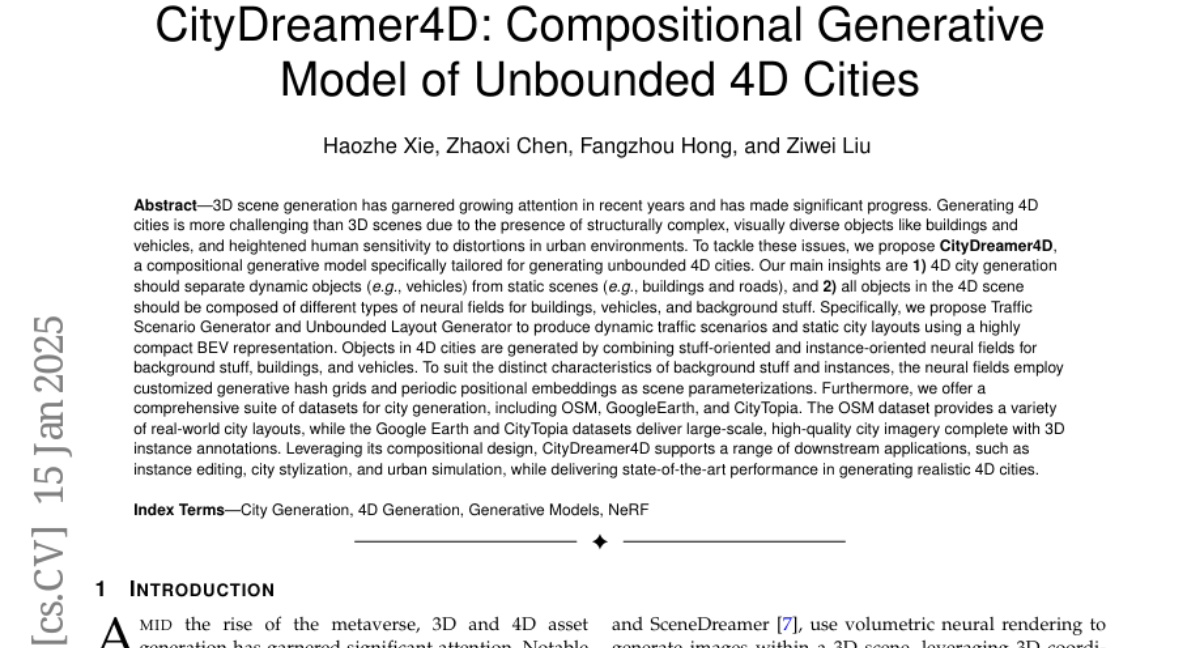
Summary
This paper talks about CityDreamer4D, a new AI system that can create realistic, moving 3D cities that can be as big as you want. It's like having a super-advanced version of SimCity that can generate entire urban landscapes with moving cars and changing scenery.
What's the problem?
Creating realistic 3D cities that move and change over time (4D) is really hard for computers. Cities have lots of complex parts like buildings and cars that all look different and move in unique ways. Plus, people are really good at spotting when something looks off in a city scene, so the AI has to be extra careful to make everything look right.
What's the solution?
The researchers made CityDreamer4D, which breaks down the city-making process into smaller, more manageable parts. It separates the things that don't move (like buildings and roads) from things that do (like cars). It uses special AI techniques to create each part of the city, like one for making buildings and another for creating traffic patterns. They also made huge datasets of real city layouts and images to help train their AI. CityDreamer4D can use all these parts to create cities that can be as big as you want and change over time.
Why it matters?
This matters because it could change how we design and plan cities in the future. Architects and urban planners could use this to visualize how new buildings or roads might affect a city before anything is built. It could help with making video games or movies with more realistic city backgrounds. Scientists could use it to study how cities might grow or change over time. Plus, it shows how breaking big, complex problems into smaller parts can help AI tackle really challenging tasks.
Abstract
3D scene generation has garnered growing attention in recent years and has made significant progress. Generating 4D cities is more challenging than 3D scenes due to the presence of structurally complex, visually diverse objects like buildings and vehicles, and heightened human sensitivity to distortions in urban environments. To tackle these issues, we propose CityDreamer4D, a compositional generative model specifically tailored for generating unbounded 4D cities. Our main insights are 1) 4D city generation should separate dynamic objects (e.g., vehicles) from static scenes (e.g., buildings and roads), and 2) all objects in the 4D scene should be composed of different types of neural fields for buildings, vehicles, and background stuff. Specifically, we propose Traffic Scenario Generator and Unbounded Layout Generator to produce dynamic traffic scenarios and static city layouts using a highly compact BEV representation. Objects in 4D cities are generated by combining stuff-oriented and instance-oriented neural fields for background stuff, buildings, and vehicles. To suit the distinct characteristics of background stuff and instances, the neural fields employ customized generative hash grids and periodic positional embeddings as scene parameterizations. Furthermore, we offer a comprehensive suite of datasets for city generation, including OSM, GoogleEarth, and CityTopia. The OSM dataset provides a variety of real-world city layouts, while the Google Earth and CityTopia datasets deliver large-scale, high-quality city imagery complete with 3D instance annotations. Leveraging its compositional design, CityDreamer4D supports a range of downstream applications, such as instance editing, city stylization, and urban simulation, while delivering state-of-the-art performance in generating realistic 4D cities.