CLAY: A Controllable Large-scale Generative Model for Creating High-quality 3D Assets
Longwen Zhang, Ziyu Wang, Qixuan Zhang, Qiwei Qiu, Anqi Pang, Haoran Jiang, Wei Yang, Lan Xu, Jingyi Yu
2024-07-19
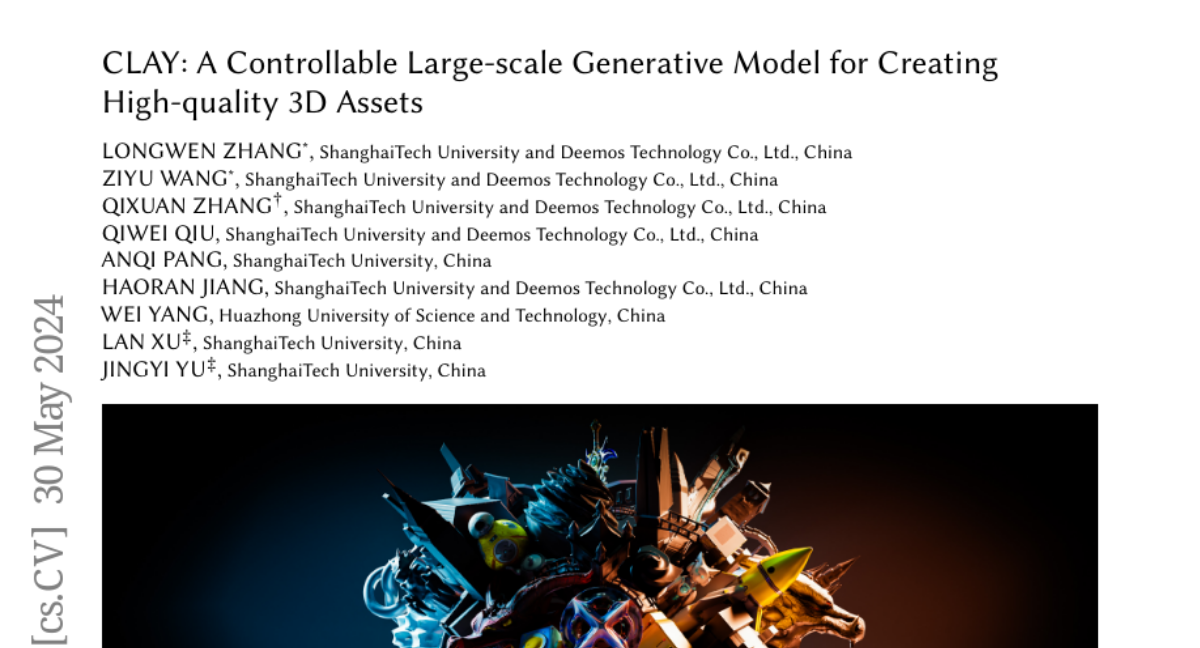
Summary
This paper introduces CLAY, a new tool that makes it easy to create high-quality 3D models and textures from simple inputs like text or images. It aims to help people turn their ideas into detailed 3D designs without needing advanced skills.
What's the problem?
Creating complex 3D models usually requires a lot of technical knowledge and experience with digital tools, which can be a barrier for many people. Existing tools can be complicated and often don't produce the desired results easily, making it hard for beginners to express their creativity in 3D.
What's the solution?
CLAY simplifies the process of generating 3D assets by allowing users to input basic information, such as text descriptions or images. It uses advanced techniques like a multi-resolution Variational Autoencoder (VAE) and a Diffusion Transformer to understand and create detailed 3D shapes and textures. The model has been trained on a massive dataset of 3D models, enabling it to produce high-quality results quickly. Even users with no prior experience can use CLAY to create everything from rough sketches to polished models ready for production.
Why it matters?
This research is important because it democratizes access to 3D modeling tools, allowing more people to engage in digital creativity. By making it easier to create detailed 3D assets, CLAY opens up opportunities in fields like game design, animation, and virtual reality, where high-quality visuals are essential.
Abstract
In the realm of digital creativity, our potential to craft intricate 3D worlds from imagination is often hampered by the limitations of existing digital tools, which demand extensive expertise and efforts. To narrow this disparity, we introduce CLAY, a 3D geometry and material generator designed to effortlessly transform human imagination into intricate 3D digital structures. CLAY supports classic text or image inputs as well as 3D-aware controls from diverse primitives (multi-view images, voxels, bounding boxes, point clouds, implicit representations, etc). At its core is a large-scale generative model composed of a multi-resolution Variational Autoencoder (VAE) and a minimalistic latent Diffusion Transformer (DiT), to extract rich 3D priors directly from a diverse range of 3D geometries. Specifically, it adopts neural fields to represent continuous and complete surfaces and uses a geometry generative module with pure transformer blocks in latent space. We present a progressive training scheme to train CLAY on an ultra large 3D model dataset obtained through a carefully designed processing pipeline, resulting in a 3D native geometry generator with 1.5 billion parameters. For appearance generation, CLAY sets out to produce physically-based rendering (PBR) textures by employing a multi-view material diffusion model that can generate 2K resolution textures with diffuse, roughness, and metallic modalities. We demonstrate using CLAY for a range of controllable 3D asset creations, from sketchy conceptual designs to production ready assets with intricate details. Even first time users can easily use CLAY to bring their vivid 3D imaginations to life, unleashing unlimited creativity.