CLEAR: Character Unlearning in Textual and Visual Modalities
Alexey Dontsov, Dmitrii Korzh, Alexey Zhavoronkin, Boris Mikheev, Denis Bobkov, Aibek Alanov, Oleg Y. Rogov, Ivan Oseledets, Elena Tutubalina
2024-10-30
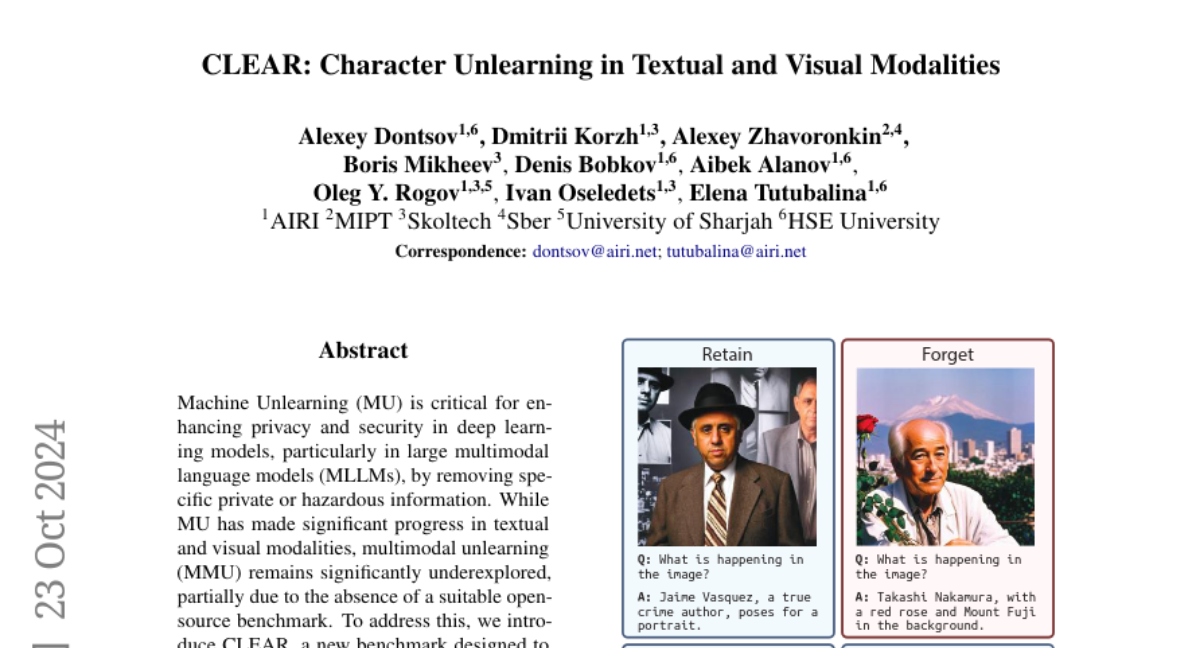
Summary
This paper introduces CLEAR, a new benchmark for testing how well machine learning models can forget specific information in both text and images to enhance privacy and security.
What's the problem?
As machine learning models become more advanced, they often store sensitive or private information that may need to be removed for privacy reasons. While there has been progress in making models forget specific data in text and images, there hasn't been much focus on how to do this effectively when combining both types of data, which is known as multimodal unlearning.
What's the solution?
To tackle this issue, the authors created CLEAR, a benchmark that includes 200 fictional individuals and 3,700 images with related question-answer pairs. This dataset allows researchers to evaluate different methods for multimodal unlearning. The study tested ten existing methods for removing information from models and introduced a new technique that helps maintain model performance while ensuring that unwanted information is forgotten. They found that using a specific regularization method significantly reduces the loss of important data during the unlearning process.
Why it matters?
This research is important because it addresses the growing need for privacy in AI systems. By developing CLEAR and improving how models can forget sensitive information, the study helps ensure that machine learning applications can be used safely without compromising user privacy. This is particularly relevant in fields like healthcare and finance, where protecting personal data is crucial.
Abstract
Machine Unlearning (MU) is critical for enhancing privacy and security in deep learning models, particularly in large multimodal language models (MLLMs), by removing specific private or hazardous information. While MU has made significant progress in textual and visual modalities, multimodal unlearning (MMU) remains significantly underexplored, partially due to the absence of a suitable open-source benchmark. To address this, we introduce CLEAR, a new benchmark designed to evaluate MMU methods. CLEAR contains 200 fictitious individuals and 3,700 images linked with corresponding question-answer pairs, enabling a thorough evaluation across modalities. We assess 10 MU methods, adapting them for MMU, and highlight new challenges specific to multimodal forgetting. We also demonstrate that simple ell_1 regularization on LoRA weights significantly mitigates catastrophic forgetting, preserving model performance on retained data. The dataset is available at https://huggingface.co/datasets/therem/CLEAR