Click-Gaussian: Interactive Segmentation to Any 3D Gaussians
Seokhun Choi, Hyeonseop Song, Jaechul Kim, Taehyeong Kim, Hoseok Do
2024-07-17
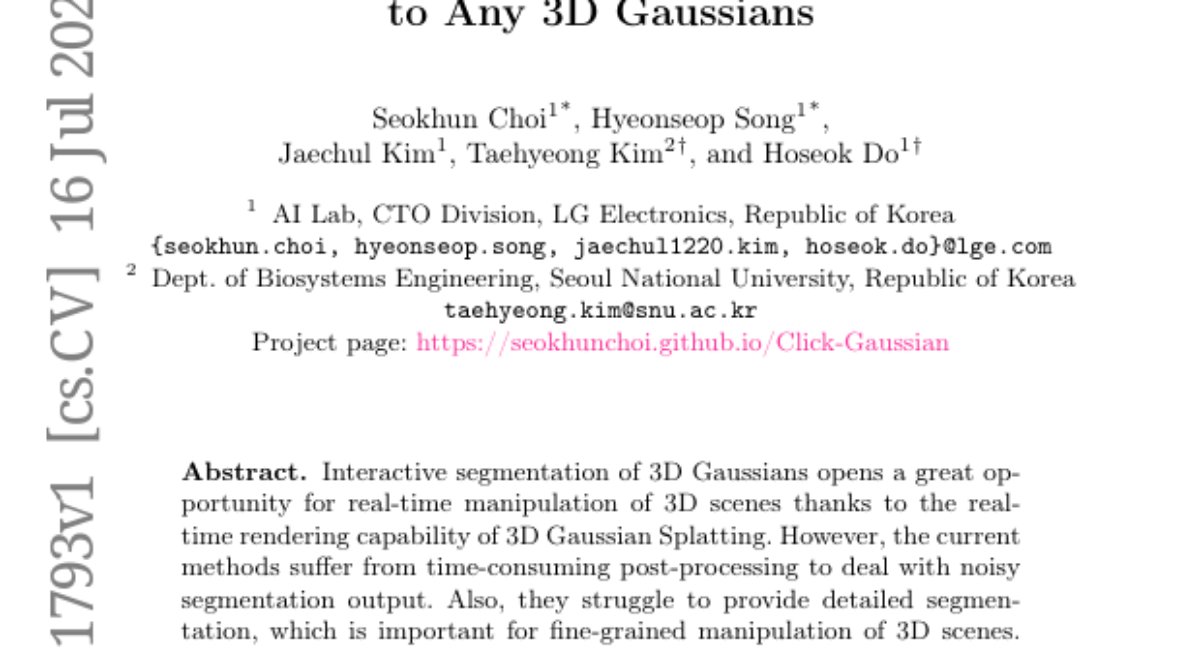
Summary
This paper introduces Click-Gaussian, a new method for interactively segmenting 3D Gaussian shapes in real-time, allowing for better manipulation of 3D scenes.
What's the problem?
Current methods for segmenting 3D objects often require a lot of time to clean up messy outputs after the initial segmentation, and they typically struggle to provide detailed and accurate results. This makes it hard to manipulate 3D scenes effectively, especially when precise adjustments are needed.
What's the solution?
Click-Gaussian solves these problems by using a two-level granularity approach to learn features from 2D segmentations without needing lengthy post-processing. It introduces a technique called Global Feature-guided Learning (GFL), which helps improve the accuracy of the segmentation by smoothing out noise from the initial 2D data. This method allows users to segment objects in just 10 milliseconds per click, making it significantly faster—up to 130 times quicker than previous methods—while also improving accuracy.
Why it matters?
This research is important because it enhances how we can interact with and manipulate 3D scenes in real-time. By making segmentation faster and more accurate, Click-Gaussian can be used in various applications like video games, virtual reality, and animation, where precise control over 3D objects is crucial.
Abstract
Interactive segmentation of 3D Gaussians opens a great opportunity for real-time manipulation of 3D scenes thanks to the real-time rendering capability of 3D Gaussian Splatting. However, the current methods suffer from time-consuming post-processing to deal with noisy segmentation output. Also, they struggle to provide detailed segmentation, which is important for fine-grained manipulation of 3D scenes. In this study, we propose Click-Gaussian, which learns distinguishable feature fields of two-level granularity, facilitating segmentation without time-consuming post-processing. We delve into challenges stemming from inconsistently learned feature fields resulting from 2D segmentation obtained independently from a 3D scene. 3D segmentation accuracy deteriorates when 2D segmentation results across the views, primary cues for 3D segmentation, are in conflict. To overcome these issues, we propose Global Feature-guided Learning (GFL). GFL constructs the clusters of global feature candidates from noisy 2D segments across the views, which smooths out noises when training the features of 3D Gaussians. Our method runs in 10 ms per click, 15 to 130 times as fast as the previous methods, while also significantly improving segmentation accuracy. Our project page is available at https://seokhunchoi.github.io/Click-Gaussian