COAT: Compressing Optimizer states and Activation for Memory-Efficient FP8 Training
Haocheng Xi, Han Cai, Ligeng Zhu, Yao Lu, Kurt Keutzer, Jianfei Chen, Song Han
2024-10-29
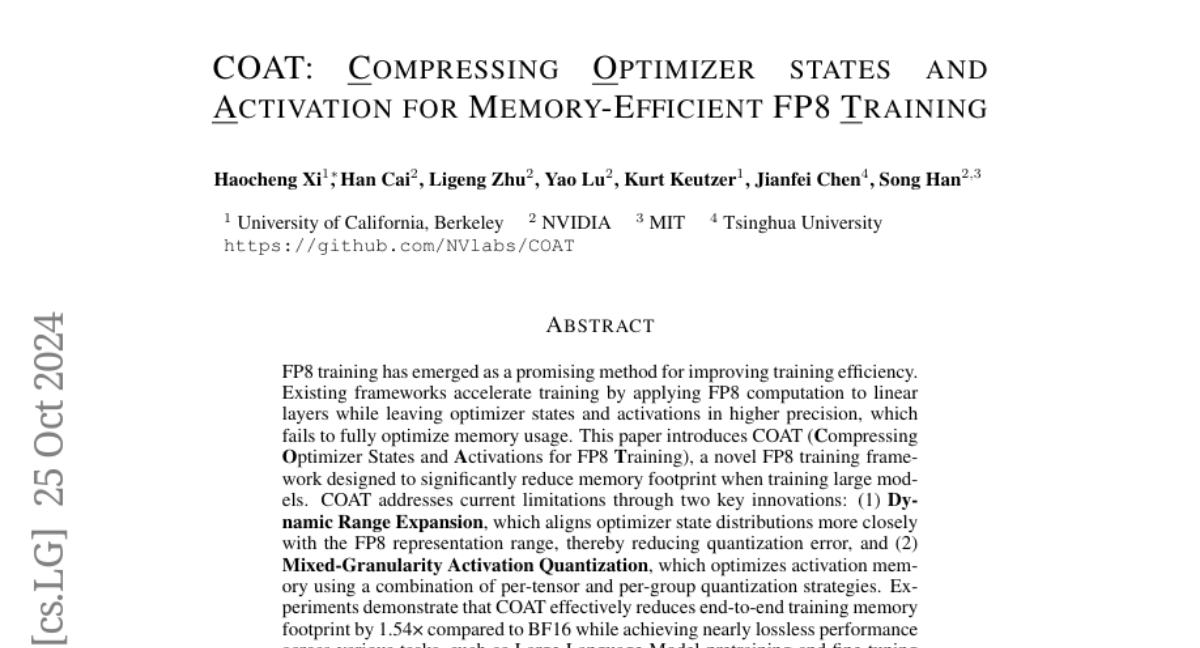
Summary
This paper discusses COAT, a new framework designed to make training large AI models more memory-efficient by compressing optimizer states and activations during FP8 training.
What's the problem?
Training large AI models can require a lot of memory, especially when using high-precision data formats. While FP8 (8-bit floating point) training improves efficiency, existing methods still use higher precision for optimizer states and activations, which does not fully optimize memory usage. This can slow down training and limit the size of models that can be trained effectively.
What's the solution?
COAT introduces two main innovations to improve memory efficiency: Dynamic Range Expansion, which helps align optimizer states with the FP8 format to reduce errors, and Mixed-Granularity Activation Quantization, which optimizes how activations are stored by using different strategies for different types of data. The results show that COAT reduces the memory needed for training by 1.54 times compared to BF16 (another precision format) while maintaining high performance across various tasks. It also speeds up training by 1.43 times compared to BF16, allowing for more efficient use of GPUs and enabling larger batch sizes.
Why it matters?
This research is important because it allows for more efficient training of large AI models, making it possible to use fewer resources while achieving better performance. This can lead to advancements in AI technology, making it easier and cheaper to develop complex models that can perform a wide range of tasks.
Abstract
FP8 training has emerged as a promising method for improving training efficiency. Existing frameworks accelerate training by applying FP8 computation to linear layers while leaving optimizer states and activations in higher precision, which fails to fully optimize memory usage. This paper introduces COAT (Compressing Optimizer States and Activations for FP8 Training), a novel FP8 training framework designed to significantly reduce memory footprint when training large models. COAT addresses current limitations through two key innovations: (1) Dynamic Range Expansion, which aligns optimizer state distributions more closely with the FP8 representation range, thereby reducing quantization error, and (2) Mixed-Granularity Activation Quantization, which optimizes activation memory using a combination of per-tensor and per-group quantization strategies. Experiments demonstrate that COAT effectively reduces end-to-end training memory footprint by 1.54x compared to BF16 while achieving nearly lossless performance across various tasks, such as Large Language Model pretraining and fine-tuning and Vision Language Model training. COAT also achieves a 1.43x end-to-end training speedup compared to BF16, performing on par with or surpassing TransformerEngine's speedup. COAT enables efficient full-parameter training of large models on fewer GPUs, and facilitates doubling the batch size in distributed training settings, providing a practical solution for scaling large-scale model training. The code is available at https://github.com/NVlabs/COAT.