CodeV: Empowering LLMs for Verilog Generation through Multi-Level Summarization
Yang Zhao, Di Huang, Chongxiao Li, Pengwei Jin, Ziyuan Nan, Tianyun Ma, Lei Qi, Yansong Pan, Zhenxing Zhang, Rui Zhang, Xishan Zhang, Zidong Du, Qi Guo, Xing Hu, Yunji Chen
2024-07-19
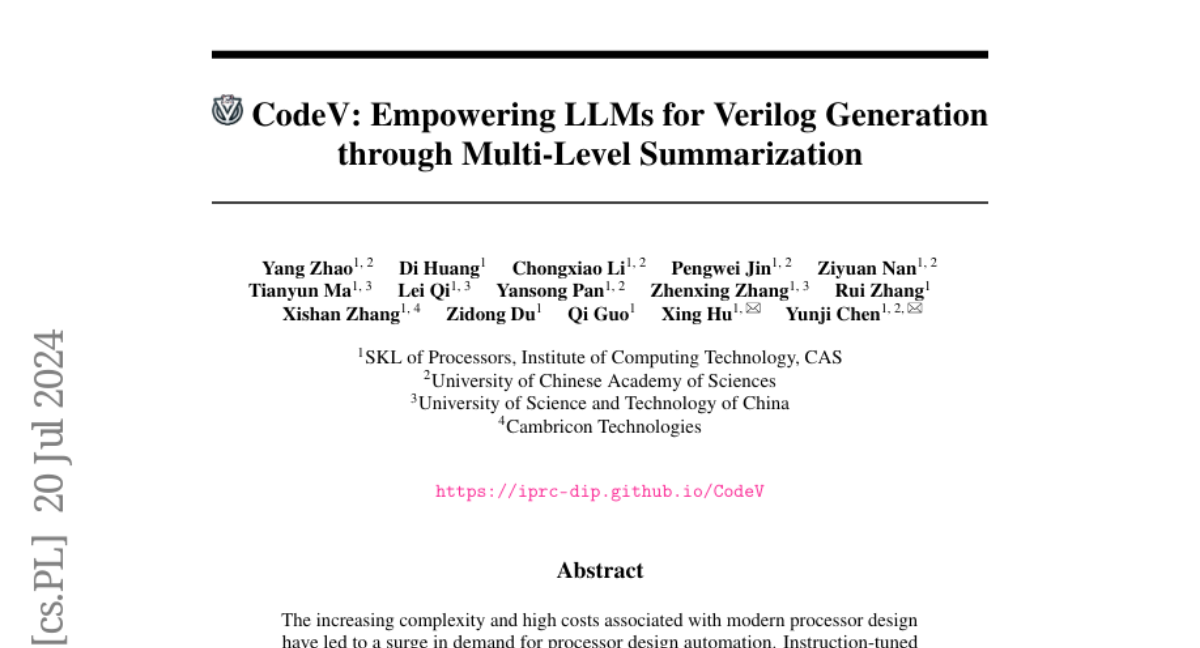
Summary
This paper introduces CodeV, a new tool designed to help large language models (LLMs) generate Verilog code more effectively. It uses a unique method of summarization to improve the quality of the generated code.
What's the problem?
As technology advances, designing processors has become more complex and expensive, leading to a need for automation in processor design. However, existing LLMs struggle with hardware description languages like Verilog due to a lack of good training data. Even advanced models like GPT-3.5 have difficulty generating accurate Verilog code, which limits their usefulness in this field.
What's the solution?
The authors developed CodeV by first collecting high-quality Verilog code from real-world sources. Instead of generating descriptions and then code, they prompt the LLM with existing Verilog code and ask it to create natural language descriptions through multi-level summarization. This innovative approach allows CodeV to produce better results than previous models, including commercial ones like GPT-4, achieving significant improvements in performance on various benchmarks.
Why it matters?
This research is important because it enhances the ability of AI models to assist in processor design, making it easier and more efficient for engineers to create complex systems. By improving how LLMs generate Verilog code, CodeV can help streamline the design process in electronics and computer engineering, ultimately contributing to advancements in technology.
Abstract
The increasing complexity and high costs associated with modern processor design have led to a surge in demand for processor design automation. Instruction-tuned large language models (LLMs) have demonstrated remarkable performance in automatically generating code for general-purpose programming languages like Python. However, these methods fail on hardware description languages (HDLs) like Verilog due to the scarcity of high-quality instruction tuning data, as even advanced LLMs like GPT-3.5 exhibit limited performance on Verilog generation. Regarding this issue, we observe that (1) Verilog code collected from the real world has higher quality than those generated by LLMs. (2) LLMs like GPT-3.5 excel in summarizing Verilog code rather than generating it. Based on these observations, this paper introduces CodeV, a series of open-source instruction-tuned Verilog generation LLMs. Instead of generating descriptions first and then getting the corresponding code from advanced LLMs, we prompt the LLM with Verilog code and let the LLM generate the corresponding natural language description by multi-level summarization. Experimental results show that CodeV relatively surpasses the previous open-source SOTA by 14.4% (BetterV in VerilogEval) and 11.3% (RTLCoder in RTLLM) respectively, and also relatively outperforms previous commercial SOTA GPT-4 by 22.1% in VerilogEval.