Cognitive Map for Language Models: Optimal Planning via Verbally Representing the World Model
Doyoung Kim, Jongwon Lee, Jinho Park, Minjoon Seo
2024-06-24
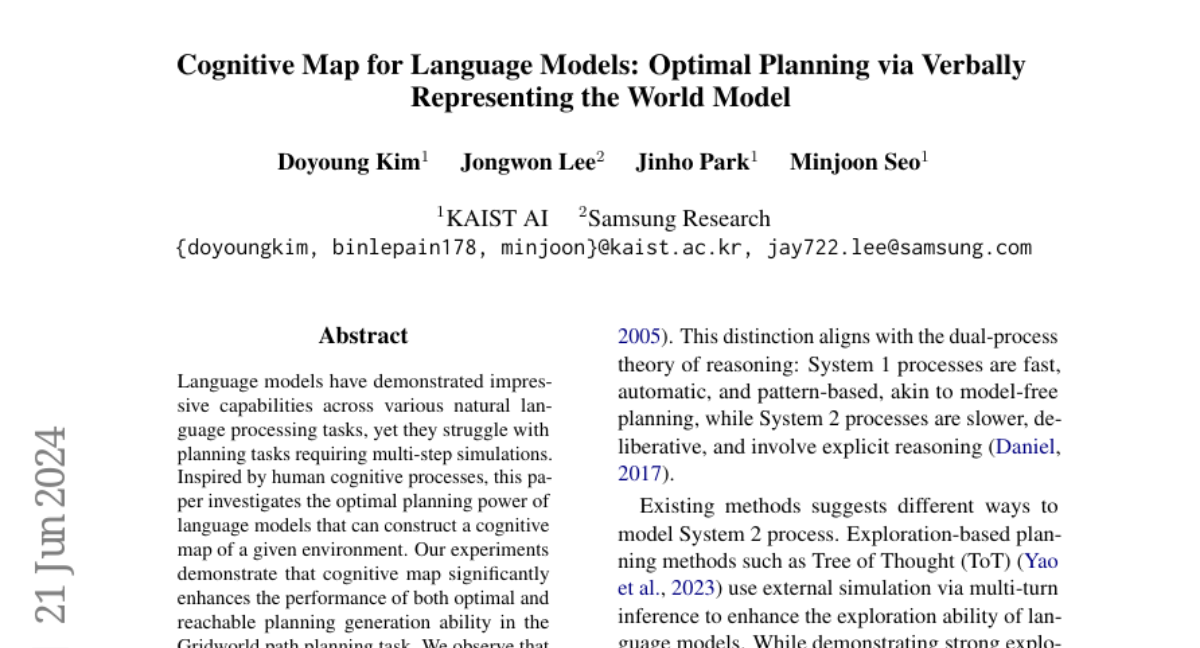
Summary
This paper explores how language models can improve their ability to plan actions in complex tasks by creating a 'cognitive map' of their environment, similar to how humans think and organize information.
What's the problem?
Language models, while good at understanding and generating text, often struggle with planning tasks that require multiple steps and simulations. This limitation makes it difficult for them to perform effectively in scenarios where sequential decision-making is needed.
What's the solution?
The researchers introduced the concept of a cognitive map, which allows language models to better visualize and understand their environment. Through experiments in a Gridworld path planning task, they found that using this cognitive map significantly improved the models' ability to plan both optimal paths and feasible routes. The models demonstrated two important traits: they could generalize their planning skills to new environments and quickly adapt even with little training data.
Why it matters?
These findings are important because they suggest that incorporating cognitive processes into language models can enhance their performance in planning tasks. By mimicking human-like thinking, these models could become more advanced and capable of tackling complex real-world problems, leading to more robust AI systems.
Abstract
Language models have demonstrated impressive capabilities across various natural language processing tasks, yet they struggle with planning tasks requiring multi-step simulations. Inspired by human cognitive processes, this paper investigates the optimal planning power of language models that can construct a cognitive map of a given environment. Our experiments demonstrate that cognitive map significantly enhances the performance of both optimal and reachable planning generation ability in the Gridworld path planning task. We observe that our method showcases two key characteristics similar to human cognition: generalization of its planning ability to extrapolated environments and rapid adaptation with limited training data. We hope our findings in the Gridworld task provide insights into modeling human cognitive processes in language models, potentially leading to the development of more advanced and robust systems that better resemble human cognition.