CogVideoX: Text-to-Video Diffusion Models with An Expert Transformer
Zhuoyi Yang, Jiayan Teng, Wendi Zheng, Ming Ding, Shiyu Huang, Jiazheng Xu, Yuanming Yang, Wenyi Hong, Xiaohan Zhang, Guanyu Feng, Da Yin, Xiaotao Gu, Yuxuan Zhang, Weihan Wang, Yean Cheng, Ting Liu, Bin Xu, Yuxiao Dong, Jie Tang
2024-08-13
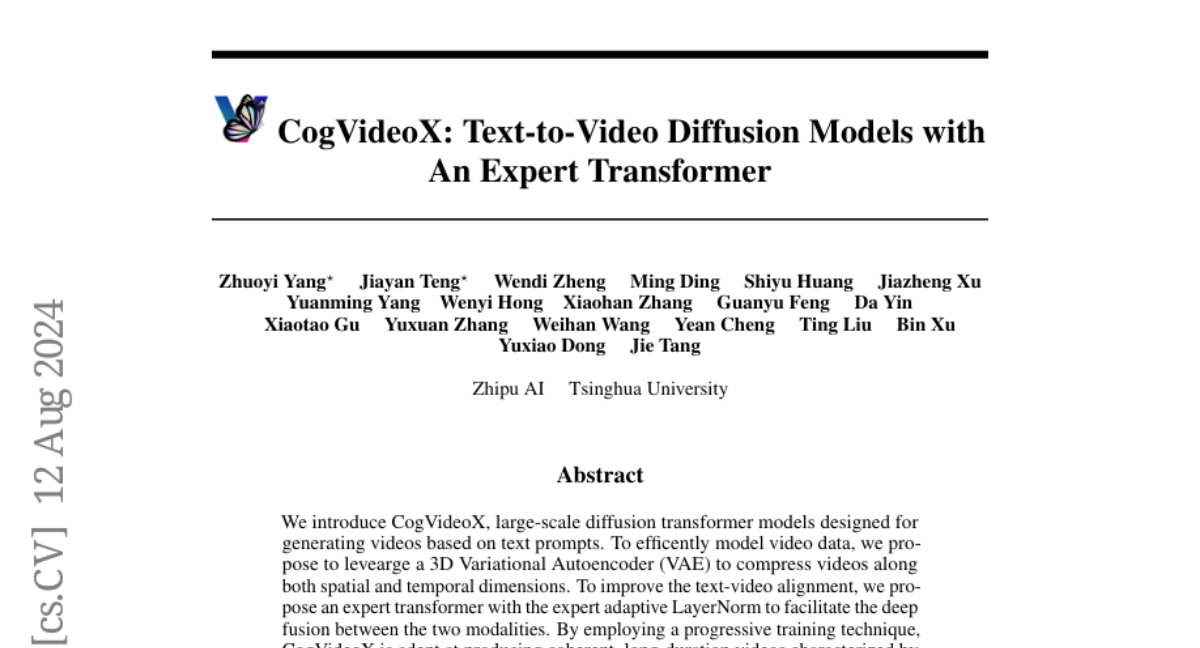
Summary
This paper introduces CogVideoX, a new model that generates videos from text descriptions using advanced AI techniques, making it easier to create high-quality videos.
What's the problem?
Generating videos from text prompts is challenging because it requires understanding both the visual and temporal aspects of video. Existing models often struggle with this task, leading to poor-quality or incoherent videos, especially when significant movements are involved.
What's the solution?
CogVideoX uses a large-scale diffusion transformer model that combines a 3D Variational Autoencoder (VAE) to compress video data effectively. It also features an expert transformer that enhances the connection between text and video, allowing for better alignment and understanding of prompts. By using progressive training techniques, CogVideoX can produce coherent and longer videos with dynamic movements. Additionally, it includes a specialized data processing pipeline to improve the quality and relevance of the generated videos.
Why it matters?
This research is important because it advances the field of AI-generated content, particularly in creating videos. With CogVideoX achieving state-of-the-art performance in generating videos from text, it opens up new possibilities for filmmakers, educators, and content creators by making video production more accessible and efficient.
Abstract
We introduce CogVideoX, a large-scale diffusion transformer model designed for generating videos based on text prompts. To efficently model video data, we propose to levearge a 3D Variational Autoencoder (VAE) to compress videos along both spatial and temporal dimensions. To improve the text-video alignment, we propose an expert transformer with the expert adaptive LayerNorm to facilitate the deep fusion between the two modalities. By employing a progressive training technique, CogVideoX is adept at producing coherent, long-duration videos characterized by significant motions. In addition, we develop an effective text-video data processing pipeline that includes various data preprocessing strategies and a video captioning method. It significantly helps enhance the performance of CogVideoX, improving both generation quality and semantic alignment. Results show that CogVideoX demonstrates state-of-the-art performance across both multiple machine metrics and human evaluations. The model weights of both the 3D Causal VAE and CogVideoX are publicly available at https://github.com/THUDM/CogVideo.