Colorful Diffuse Intrinsic Image Decomposition in the Wild
Chris Careaga, Yağız Aksoy
2024-09-23
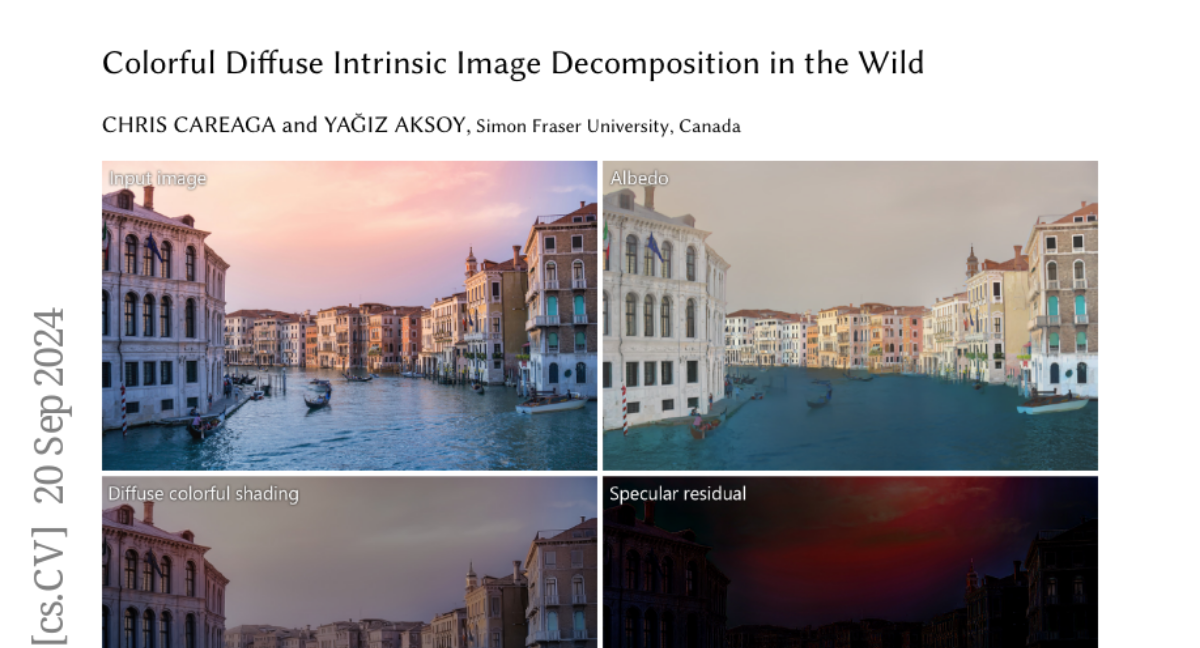
Summary
This paper discusses a new method for breaking down images into their basic components, specifically focusing on separating colors and lighting effects in photographs. The method, called intrinsic image decomposition, allows for better editing and understanding of images taken in real-world settings.
What's the problem?
Traditional methods for separating an image's colors and lighting often assume that the lighting is a single color and that surfaces reflect light uniformly. This makes it difficult to accurately analyze and edit images with complex lighting conditions, which limits their use in applications like photo editing where understanding the true nature of colors and shadows is essential.
What's the solution?
The researchers developed a new approach that gradually removes these simplifying assumptions. They break the problem into smaller, more manageable parts, allowing them to separate an image into three key components: diffuse albedo (the inherent color of surfaces), colorful diffuse shading (how light interacts with those surfaces), and specular residuals (shiny highlights). This method enables better analysis of photographs by handling complex lighting situations more effectively, even with limited data available for training.
Why it matters?
This research is significant because it enhances our ability to edit and analyze photographs in a way that reflects real-world conditions. By improving how we understand and manipulate images, this method can lead to better tools for photographers, artists, and anyone working with visual media, ultimately allowing for more realistic representations of scenes.
Abstract
Intrinsic image decomposition aims to separate the surface reflectance and the effects from the illumination given a single photograph. Due to the complexity of the problem, most prior works assume a single-color illumination and a Lambertian world, which limits their use in illumination-aware image editing applications. In this work, we separate an input image into its diffuse albedo, colorful diffuse shading, and specular residual components. We arrive at our result by gradually removing first the single-color illumination and then the Lambertian-world assumptions. We show that by dividing the problem into easier sub-problems, in-the-wild colorful diffuse shading estimation can be achieved despite the limited ground-truth datasets. Our extended intrinsic model enables illumination-aware analysis of photographs and can be used for image editing applications such as specularity removal and per-pixel white balancing.