Commonsense-T2I Challenge: Can Text-to-Image Generation Models Understand Commonsense?
Xingyu Fu, Muyu He, Yujie Lu, William Yang Wang, Dan Roth
2024-06-14
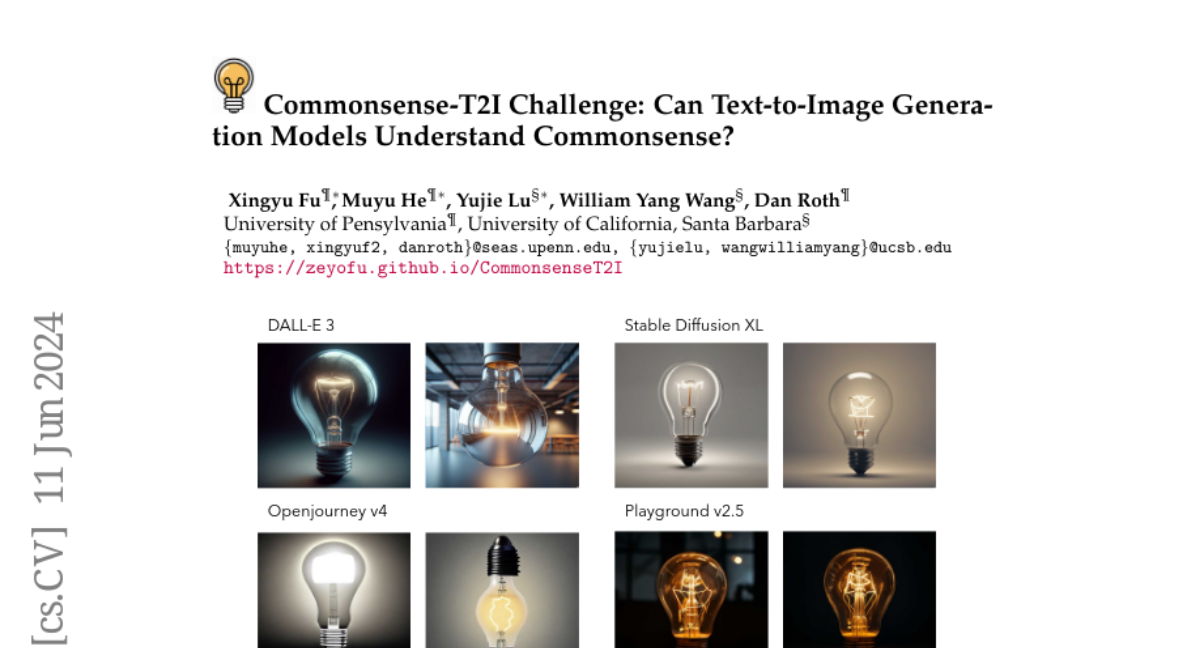
Summary
This paper introduces the Commonsense-T2I Challenge, a new way to test how well text-to-image (T2I) generation models can create images that make sense in real life. The challenge evaluates whether these models can understand and visualize commonsense concepts based on text prompts.
What's the problem?
Many T2I models can generate impressive images from text, but they often struggle with commonsense reasoning. For example, if given the prompts 'a lightbulb without electricity' and 'a lightbulb with electricity,' the models need to produce images that accurately reflect these scenarios. Current models have difficulty making the right visual distinctions based on subtle differences in prompts, which shows a gap between their image generation capabilities and real-world logic.
What's the solution?
The authors created a dataset called Commonsense-T2I, which includes pairs of text prompts that are similar but require different images. Each prompt pair is carefully designed to test the model's understanding of commonsense reasoning. The dataset is annotated with details like the type of commonsense involved and how likely each expected output is. The researchers tested various state-of-the-art T2I models using this dataset and found that even advanced models like DALL-E 3 performed poorly, achieving only about 49% accuracy in generating the correct images.
Why it matters?
This research is important because it highlights the limitations of current T2I models in understanding real-world commonsense. By establishing the Commonsense-T2I Challenge as a benchmark, it encourages further development of AI systems that can generate images more accurately based on human-like reasoning. Improving these models can lead to better applications in fields like art, education, and virtual reality, where understanding context and commonsense is crucial.
Abstract
We present a novel task and benchmark for evaluating the ability of text-to-image(T2I) generation models to produce images that fit commonsense in real life, which we call Commonsense-T2I. Given two adversarial text prompts containing an identical set of action words with minor differences, such as "a lightbulb without electricity" v.s. "a lightbulb with electricity", we evaluate whether T2I models can conduct visual-commonsense reasoning, e.g. produce images that fit "the lightbulb is unlit" vs. "the lightbulb is lit" correspondingly. Commonsense-T2I presents an adversarial challenge, providing pairwise text prompts along with expected outputs. The dataset is carefully hand-curated by experts and annotated with fine-grained labels, such as commonsense type and likelihood of the expected outputs, to assist analyzing model behavior. We benchmark a variety of state-of-the-art (sota) T2I models and surprisingly find that, there is still a large gap between image synthesis and real life photos--even the DALL-E 3 model could only achieve 48.92% on Commonsense-T2I, and the stable diffusion XL model only achieves 24.92% accuracy. Our experiments show that GPT-enriched prompts cannot solve this challenge, and we include a detailed analysis about possible reasons for such deficiency. We aim for Commonsense-T2I to serve as a high-quality evaluation benchmark for T2I commonsense checking, fostering advancements in real life image generation.