Communication-Efficient Language Model Training Scales Reliably and Robustly: Scaling Laws for DiLoCo
Zachary Charles, Gabriel Teston, Lucio Dery, Keith Rush, Nova Fallen, Zachary Garrett, Arthur Szlam, Arthur Douillard
2025-03-14
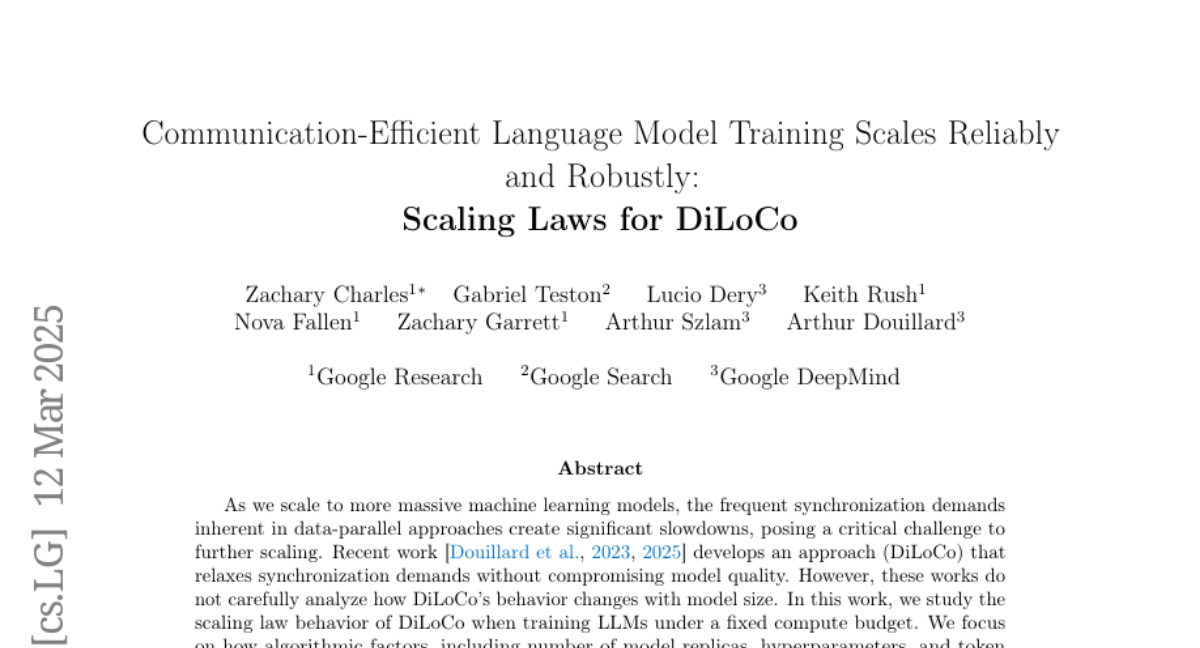
Summary
This paper explores a method called DiLoCo that makes training large language models more efficient by reducing the need for constant synchronization, which usually slows things down.
What's the problem?
Training massive machine learning models is difficult because data-parallel approaches require frequent synchronization, leading to slowdowns and hindering further scaling.
What's the solution?
The researchers studied how DiLoCo behaves as model size increases. They found that DiLoCo scales predictably and robustly, often outperforming data-parallel training, especially when well-tuned. This approach also increases optimal batch sizes and improves downstream generalization.
Why it matters?
This work matters because it provides a way to train larger models more efficiently, which leads to better performance and allows for advancements in various AI applications.
Abstract
As we scale to more massive machine learning models, the frequent synchronization demands inherent in data-parallel approaches create significant slowdowns, posing a critical challenge to further scaling. Recent work develops an approach (DiLoCo) that relaxes synchronization demands without compromising model quality. However, these works do not carefully analyze how DiLoCo's behavior changes with model size. In this work, we study the scaling law behavior of DiLoCo when training LLMs under a fixed compute budget. We focus on how algorithmic factors, including number of model replicas, hyperparameters, and token budget affect training in ways that can be accurately predicted via scaling laws. We find that DiLoCo scales both predictably and robustly with model size. When well-tuned, DiLoCo scales better than data-parallel training with model size, and can outperform data-parallel training even at small model sizes. Our results showcase a more general set of benefits of DiLoCo than previously documented, including increased optimal batch sizes, improved downstream generalization with scale, and improved evaluation loss for a fixed token budget.