Compact 3D Gaussian Splatting for Static and Dynamic Radiance Fields
Joo Chan Lee, Daniel Rho, Xiangyu Sun, Jong Hwan Ko, Eunbyung Park
2024-08-08
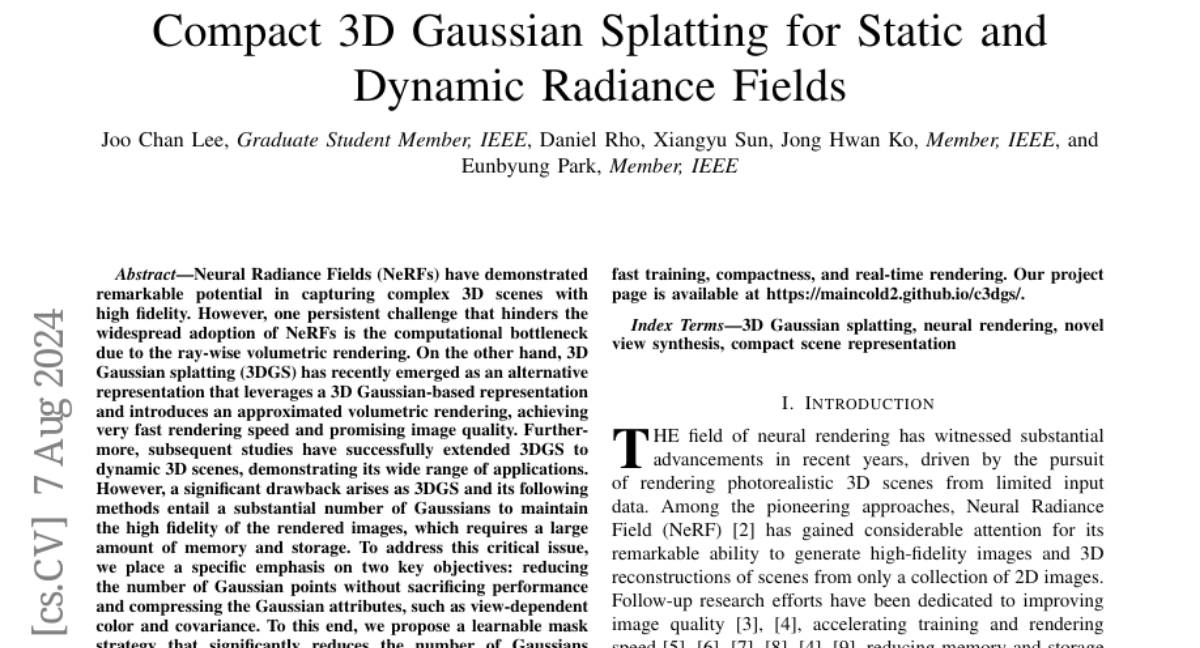
Summary
This paper discusses Compact 3D Gaussian Splatting, a new method for efficiently rendering both static and dynamic 3D scenes using fewer resources while maintaining high image quality.
What's the problem?
3D Gaussian splatting (3DGS) is a technique that helps create realistic 3D images quickly, but it often requires a lot of memory and storage because it uses many Gaussian points to maintain image quality. This can make it difficult to use in real-world applications where resources are limited.
What's the solution?
To solve this problem, the authors developed a method that reduces the number of Gaussian points needed without losing image quality. They introduced a learnable mask strategy that helps keep performance high while using fewer resources. Additionally, they created a new way to represent color information using a grid-based system instead of traditional methods, which makes it more efficient. They also used advanced techniques to compress the data, achieving over 25 times less storage for static scenes and more than 12 times for dynamic scenes while still producing high-quality images.
Why it matters?
This research is important because it allows for faster and more efficient rendering of 3D scenes, making it easier to create realistic graphics in video games, movies, and virtual reality applications. By improving how we represent and render complex scenes, this work can lead to better visual experiences with less computational power.
Abstract
3D Gaussian splatting (3DGS) has recently emerged as an alternative representation that leverages a 3D Gaussian-based representation and introduces an approximated volumetric rendering, achieving very fast rendering speed and promising image quality. Furthermore, subsequent studies have successfully extended 3DGS to dynamic 3D scenes, demonstrating its wide range of applications. However, a significant drawback arises as 3DGS and its following methods entail a substantial number of Gaussians to maintain the high fidelity of the rendered images, which requires a large amount of memory and storage. To address this critical issue, we place a specific emphasis on two key objectives: reducing the number of Gaussian points without sacrificing performance and compressing the Gaussian attributes, such as view-dependent color and covariance. To this end, we propose a learnable mask strategy that significantly reduces the number of Gaussians while preserving high performance. In addition, we propose a compact but effective representation of view-dependent color by employing a grid-based neural field rather than relying on spherical harmonics. Finally, we learn codebooks to compactly represent the geometric and temporal attributes by residual vector quantization. With model compression techniques such as quantization and entropy coding, we consistently show over 25x reduced storage and enhanced rendering speed compared to 3DGS for static scenes, while maintaining the quality of the scene representation. For dynamic scenes, our approach achieves more than 12x storage efficiency and retains a high-quality reconstruction compared to the existing state-of-the-art methods. Our work provides a comprehensive framework for 3D scene representation, achieving high performance, fast training, compactness, and real-time rendering. Our project page is available at https://maincold2.github.io/c3dgs/.