Complexity of Symbolic Representation in Working Memory of Transformer Correlates with the Complexity of a Task
Alsu Sagirova, Mikhail Burtsev
2024-06-24
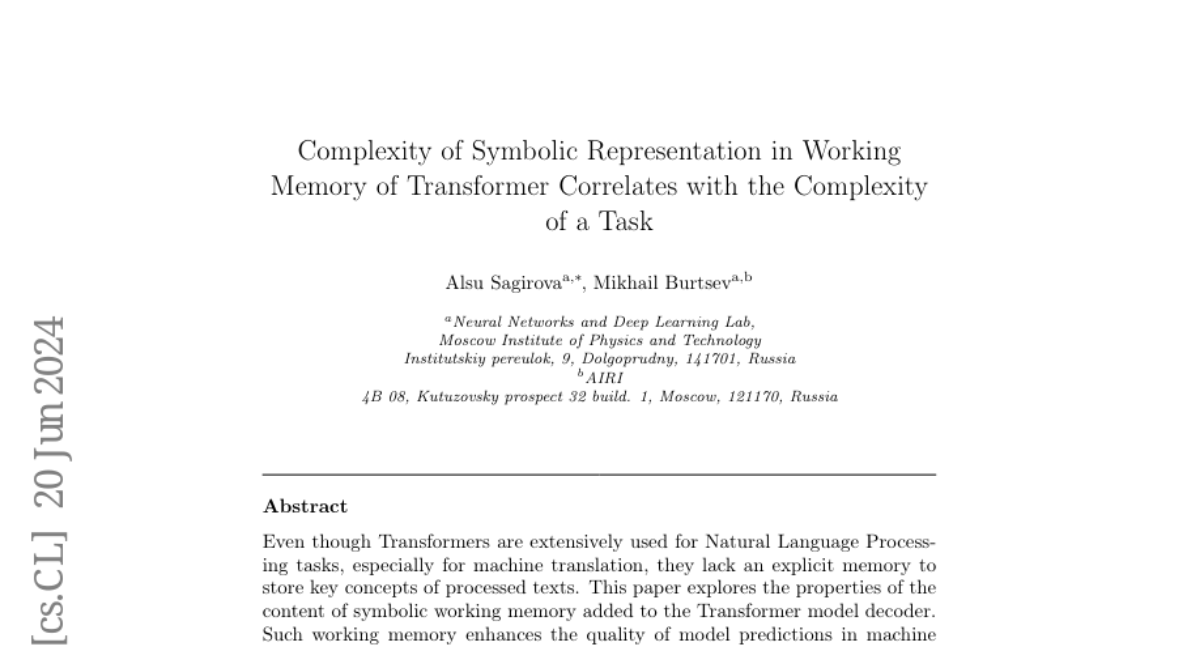
Summary
This paper investigates how adding a working memory to Transformer models can improve their ability to translate text by allowing them to store and use important information during the translation process.
What's the problem?
Transformers are widely used in natural language processing, especially for tasks like translating languages. However, they do not have a built-in memory system to remember key concepts from the texts they process. This limitation can lead to less accurate translations because the model might forget important details while generating the output.
What's the solution?
The researchers added a symbolic working memory to the Transformer model's decoder, which allows the model to store important keywords and information from the text it is translating. This working memory helps the model keep track of relevant information, improving its predictions and overall translation quality. The study found that the keywords from the translated text are stored in this memory, and the variety of words and parts of speech stored correlates with how complex the text being translated is.
Why it matters?
This research is important because it shows that enhancing Transformer models with a working memory can significantly improve their performance in tasks like machine translation. By allowing these models to remember and use key information, we can create more accurate and reliable AI systems for translating languages, which is crucial for communication in our increasingly globalized world.
Abstract
Even though Transformers are extensively used for Natural Language Processing tasks, especially for machine translation, they lack an explicit memory to store key concepts of processed texts. This paper explores the properties of the content of symbolic working memory added to the Transformer model decoder. Such working memory enhances the quality of model predictions in machine translation task and works as a neural-symbolic representation of information that is important for the model to make correct translations. The study of memory content revealed that translated text keywords are stored in the working memory, pointing to the relevance of memory content to the processed text. Also, the diversity of tokens and parts of speech stored in memory correlates with the complexity of the corpora for machine translation task.