Compositional 3D-aware Video Generation with LLM Director
Hanxin Zhu, Tianyu He, Anni Tang, Junliang Guo, Zhibo Chen, Jiang Bian
2024-09-04
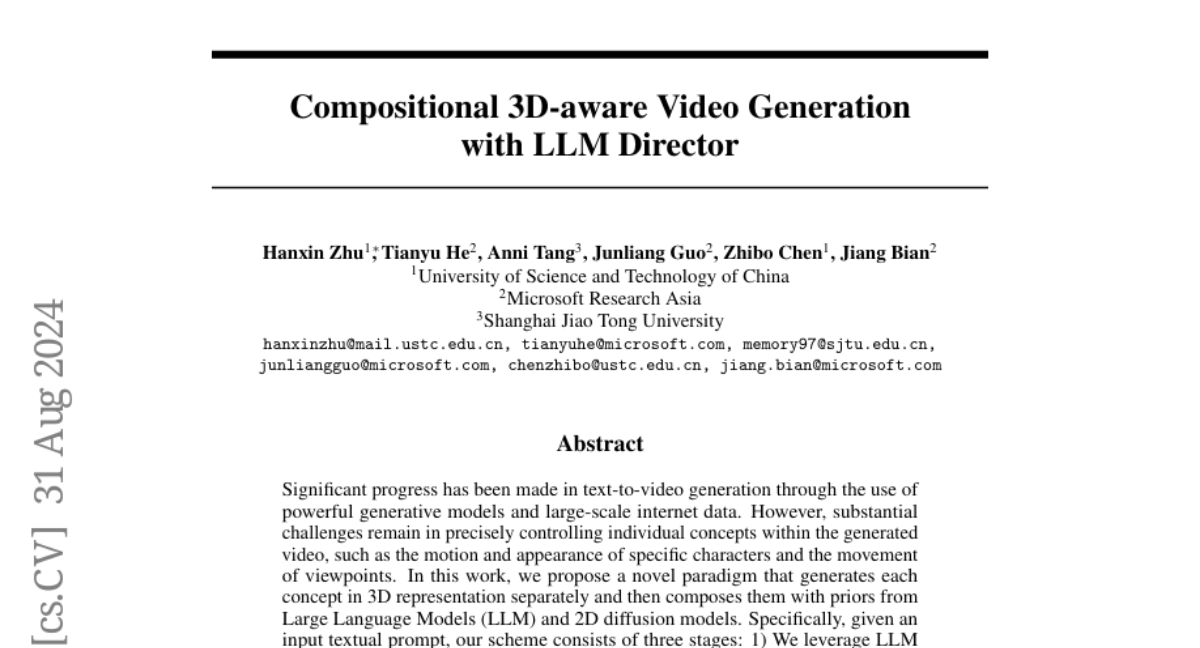
Summary
This paper talks about a new method called Diffusion Policy Policy Optimization (DPPO) that helps improve how robots learn to perform tasks by using a specific type of AI known as diffusion-based policies.
What's the problem?
Many existing methods for training robots to perform tasks can be inefficient, especially when using diffusion-based policies. These methods often struggle to effectively fine-tune the robot's learning process, making it harder for robots to adapt and perform well in various environments.
What's the solution?
DPPO introduces a framework that combines best practices for fine-tuning diffusion-based policies with reinforcement learning techniques. It optimizes the training process by leveraging the strengths of diffusion models, allowing robots to learn more efficiently and effectively. The researchers conducted experiments that showed DPPO outperforms other methods in training robots for continuous control tasks, leading to better performance in real-world scenarios.
Why it matters?
This research is important because it enhances the ability of robots to learn and adapt to new tasks more efficiently. By improving the training methods used for robotic learning, DPPO can lead to better performance in practical applications such as manufacturing, healthcare, and autonomous vehicles, ultimately making robots more useful in everyday life.
Abstract
Significant progress has been made in text-to-video generation through the use of powerful generative models and large-scale internet data. However, substantial challenges remain in precisely controlling individual concepts within the generated video, such as the motion and appearance of specific characters and the movement of viewpoints. In this work, we propose a novel paradigm that generates each concept in 3D representation separately and then composes them with priors from Large Language Models (LLM) and 2D diffusion models. Specifically, given an input textual prompt, our scheme consists of three stages: 1) We leverage LLM as the director to first decompose the complex query into several sub-prompts that indicate individual concepts within the video~(e.g., scene, objects, motions), then we let LLM to invoke pre-trained expert models to obtain corresponding 3D representations of concepts. 2) To compose these representations, we prompt multi-modal LLM to produce coarse guidance on the scales and coordinates of trajectories for the objects. 3) To make the generated frames adhere to natural image distribution, we further leverage 2D diffusion priors and use Score Distillation Sampling to refine the composition. Extensive experiments demonstrate that our method can generate high-fidelity videos from text with diverse motion and flexible control over each concept. Project page: https://aka.ms/c3v.