Compositional Video Generation as Flow Equalization
Xingyi Yang, Xinchao Wang
2024-07-09
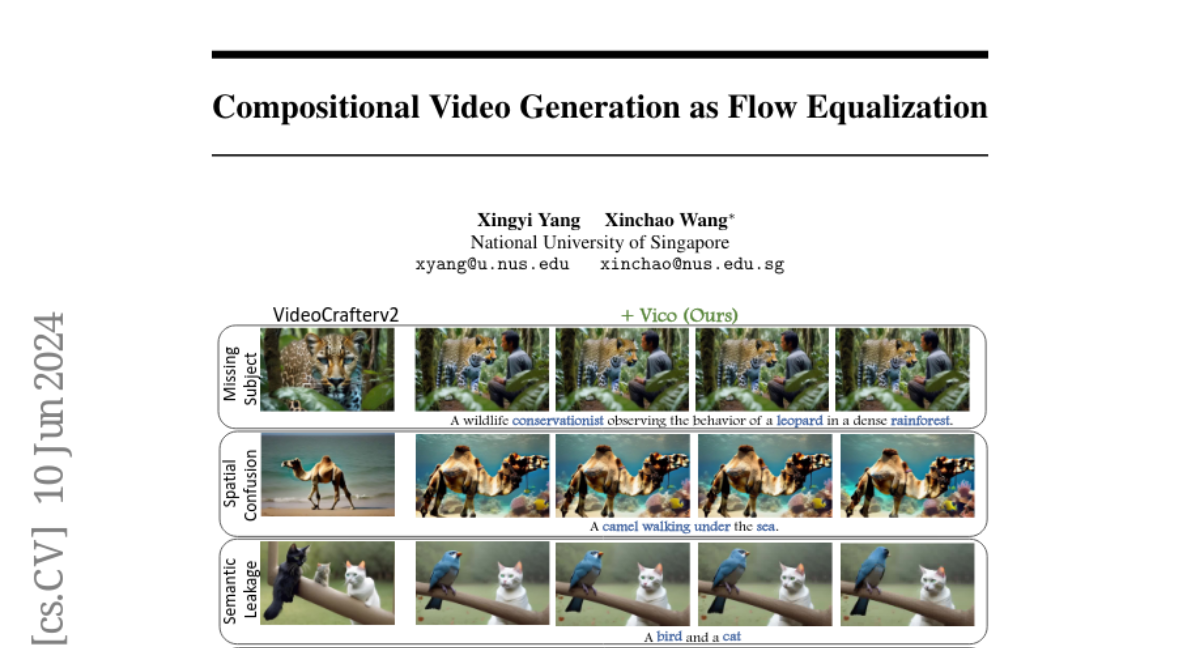
Summary
This paper talks about Vico, a new framework designed to improve the way models generate videos from text descriptions by ensuring that all concepts are represented equally and accurately in the final video.
What's the problem?
The main problem is that existing text-to-video models often struggle with complex scenes that involve multiple concepts or actions. When generating a video, some words can dominate the output, overshadowing other important details. This means that the resulting video may not accurately reflect the full meaning of the text description, leading to videos that are less coherent or realistic.
What's the solution?
To solve this issue, the authors developed Vico, which helps balance how different concepts are represented in the generated videos. Vico analyzes how each word in the input text influences the video by creating a spatial-temporal attention graph that shows how information flows from the text to the video. It uses an efficient method to approximate this flow, allowing the model to adjust and ensure that no single concept overpowers others. By doing this, Vico produces videos that better match the intended meaning of the text descriptions.
Why it matters?
This research is important because it enhances the quality of videos generated from text, making them more accurate and visually appealing. By improving how models understand and represent multiple concepts in a scene, Vico could have significant applications in fields like film production, advertising, and education, where clear and coherent visual storytelling is essential.
Abstract
Large-scale Text-to-Video (T2V) diffusion models have recently demonstrated unprecedented capability to transform natural language descriptions into stunning and photorealistic videos. Despite the promising results, a significant challenge remains: these models struggle to fully grasp complex compositional interactions between multiple concepts and actions. This issue arises when some words dominantly influence the final video, overshadowing other concepts.To tackle this problem, we introduce Vico, a generic framework for compositional video generation that explicitly ensures all concepts are represented properly. At its core, Vico analyzes how input tokens influence the generated video, and adjusts the model to prevent any single concept from dominating. Specifically, Vico extracts attention weights from all layers to build a spatial-temporal attention graph, and then estimates the influence as the max-flow from the source text token to the video target token. Although the direct computation of attention flow in diffusion models is typically infeasible, we devise an efficient approximation based on subgraph flows and employ a fast and vectorized implementation, which in turn makes the flow computation manageable and differentiable. By updating the noisy latent to balance these flows, Vico captures complex interactions and consequently produces videos that closely adhere to textual descriptions. We apply our method to multiple diffusion-based video models for compositional T2V and video editing. Empirical results demonstrate that our framework significantly enhances the compositional richness and accuracy of the generated videos. Visit our website at~https://adamdad.github.io/vico/{https://adamdad.github.io/vico/}.