Constant Acceleration Flow
Dogyun Park, Sojin Lee, Sihyeon Kim, Taehoon Lee, Youngjoon Hong, Hyunwoo J. Kim
2024-11-04
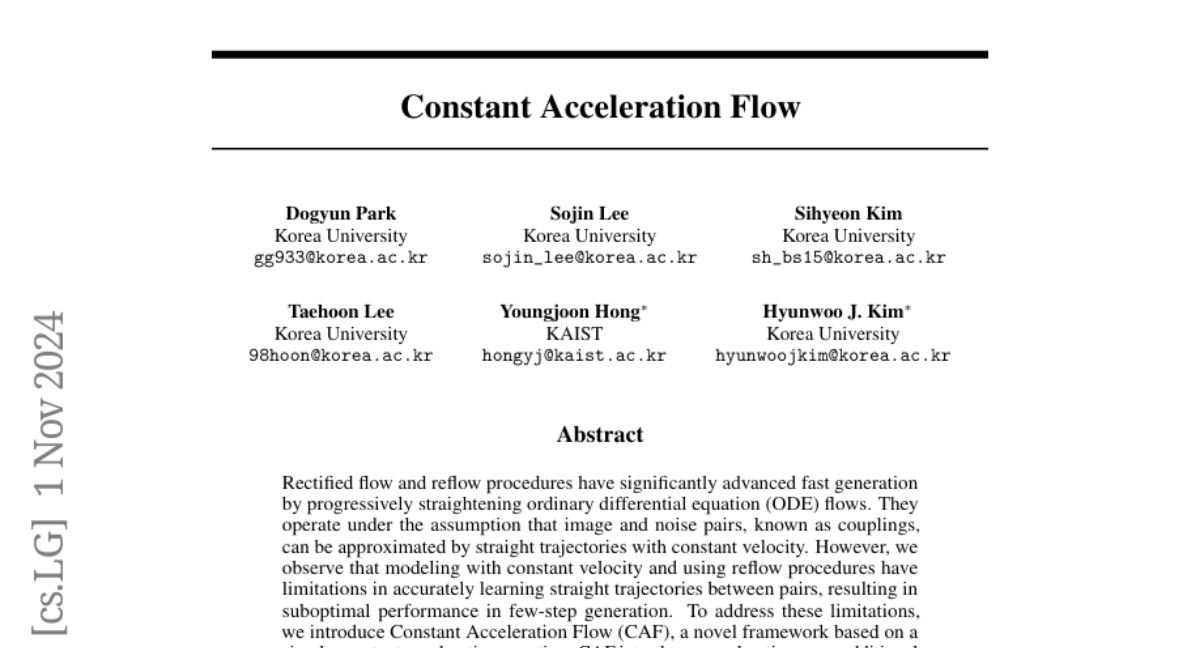
Summary
This paper introduces a new method called Constant Acceleration Flow (CAF) for generating images and other data more efficiently. It improves upon existing methods by allowing for changes in speed, making the generation process faster and more accurate.
What's the problem?
Current methods for generating images often rely on the assumption that changes happen at a constant speed, which can lead to inaccuracies, especially when trying to create images in just a few steps. This limitation can result in lower quality images when using these methods.
What's the solution?
The authors developed CAF, which incorporates acceleration as a variable that can change, rather than just keeping speed constant. This allows the model to better understand how to transition between different states when generating images. They also introduced techniques to improve how the model learns about initial speeds and how to adjust them during the generation process.
Why it matters?
This research is significant because it enhances the ability of generative models to create high-quality images quickly. By improving how these models work, CAF could have a wide range of applications in fields like computer graphics, video game design, and artificial intelligence, making it easier to produce realistic visuals.
Abstract
Rectified flow and reflow procedures have significantly advanced fast generation by progressively straightening ordinary differential equation (ODE) flows. They operate under the assumption that image and noise pairs, known as couplings, can be approximated by straight trajectories with constant velocity. However, we observe that modeling with constant velocity and using reflow procedures have limitations in accurately learning straight trajectories between pairs, resulting in suboptimal performance in few-step generation. To address these limitations, we introduce Constant Acceleration Flow (CAF), a novel framework based on a simple constant acceleration equation. CAF introduces acceleration as an additional learnable variable, allowing for more expressive and accurate estimation of the ODE flow. Moreover, we propose two techniques to further improve estimation accuracy: initial velocity conditioning for the acceleration model and a reflow process for the initial velocity. Our comprehensive studies on toy datasets, CIFAR-10, and ImageNet 64x64 demonstrate that CAF outperforms state-of-the-art baselines for one-step generation. We also show that CAF dramatically improves few-step coupling preservation and inversion over Rectified flow. Code is available at https://github.com/mlvlab/CAF{https://github.com/mlvlab/CAF}.