Contrastive Localized Language-Image Pre-Training
Hong-You Chen, Zhengfeng Lai, Haotian Zhang, Xinze Wang, Marcin Eichner, Keen You, Meng Cao, Bowen Zhang, Yinfei Yang, Zhe Gan
2024-10-04
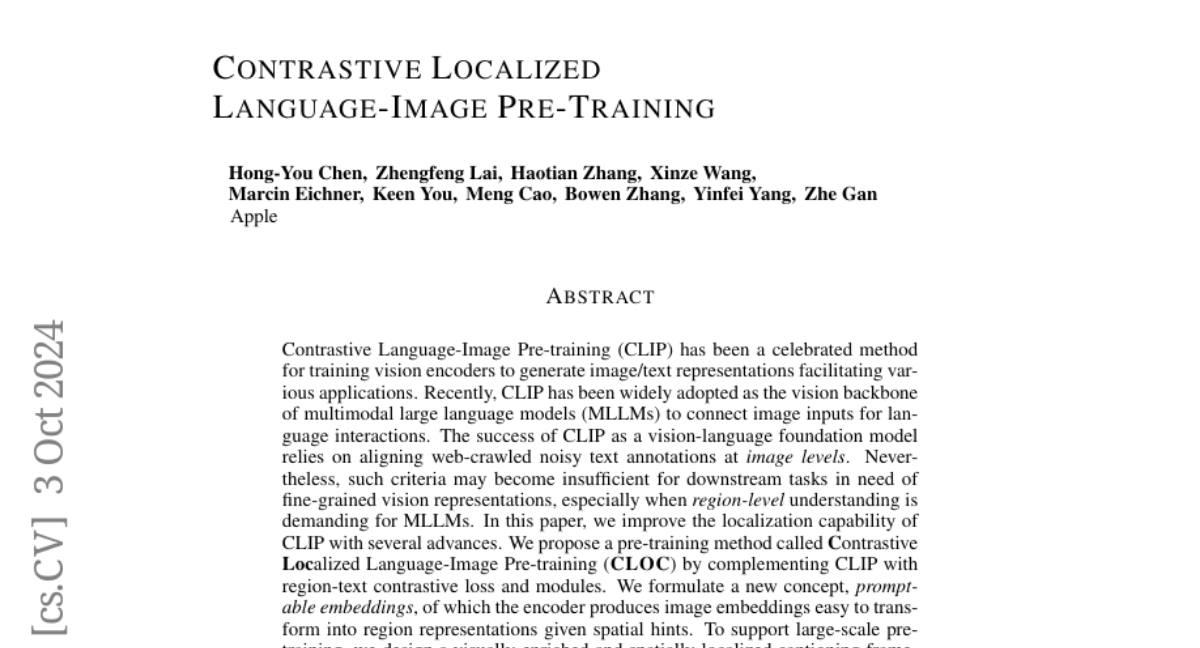
Summary
This paper introduces Contrastive Localized Language-Image Pre-Training (CLOC), a new method designed to enhance the ability of multimodal models to understand and generate detailed information from images and text.
What's the problem?
While existing models like CLIP have been successful in connecting images and text, they often struggle with tasks that require understanding specific parts of an image, such as identifying objects or regions. The current methods rely on general image-level annotations, which may not provide enough detail for more complex tasks that need fine-grained understanding.
What's the solution?
To improve this, the authors developed CLOC, which adds new techniques to CLIP that focus on region-level understanding. They introduced a training method that uses region-text contrastive loss, allowing the model to learn better relationships between specific areas of an image and corresponding text descriptions. Additionally, they created a system to generate a large-scale dataset with detailed region-text labels, enabling the model to learn from billions of annotated images. This approach helps the model produce high-quality representations for recognizing and retrieving specific parts of images.
Why it matters?
This research is important because it enhances the performance of multimodal models in tasks that require precise understanding of images and text. By enabling better region-level recognition, CLOC can improve applications in areas like image search engines, automated content creation, and assistive technologies that rely on accurately interpreting visual information.
Abstract
Contrastive Language-Image Pre-training (CLIP) has been a celebrated method for training vision encoders to generate image/text representations facilitating various applications. Recently, CLIP has been widely adopted as the vision backbone of multimodal large language models (MLLMs) to connect image inputs for language interactions. The success of CLIP as a vision-language foundation model relies on aligning web-crawled noisy text annotations at image levels. Nevertheless, such criteria may become insufficient for downstream tasks in need of fine-grained vision representations, especially when region-level understanding is demanding for MLLMs. In this paper, we improve the localization capability of CLIP with several advances. We propose a pre-training method called Contrastive Localized Language-Image Pre-training (CLOC) by complementing CLIP with region-text contrastive loss and modules. We formulate a new concept, promptable embeddings, of which the encoder produces image embeddings easy to transform into region representations given spatial hints. To support large-scale pre-training, we design a visually-enriched and spatially-localized captioning framework to effectively generate region-text pseudo-labels at scale. By scaling up to billions of annotated images, CLOC enables high-quality regional embeddings for image region recognition and retrieval tasks, and can be a drop-in replacement of CLIP to enhance MLLMs, especially on referring and grounding tasks.