Controllable Safety Alignment: Inference-Time Adaptation to Diverse Safety Requirements
Jingyu Zhang, Ahmed Elgohary, Ahmed Magooda, Daniel Khashabi, Benjamin Van Durme
2024-10-17
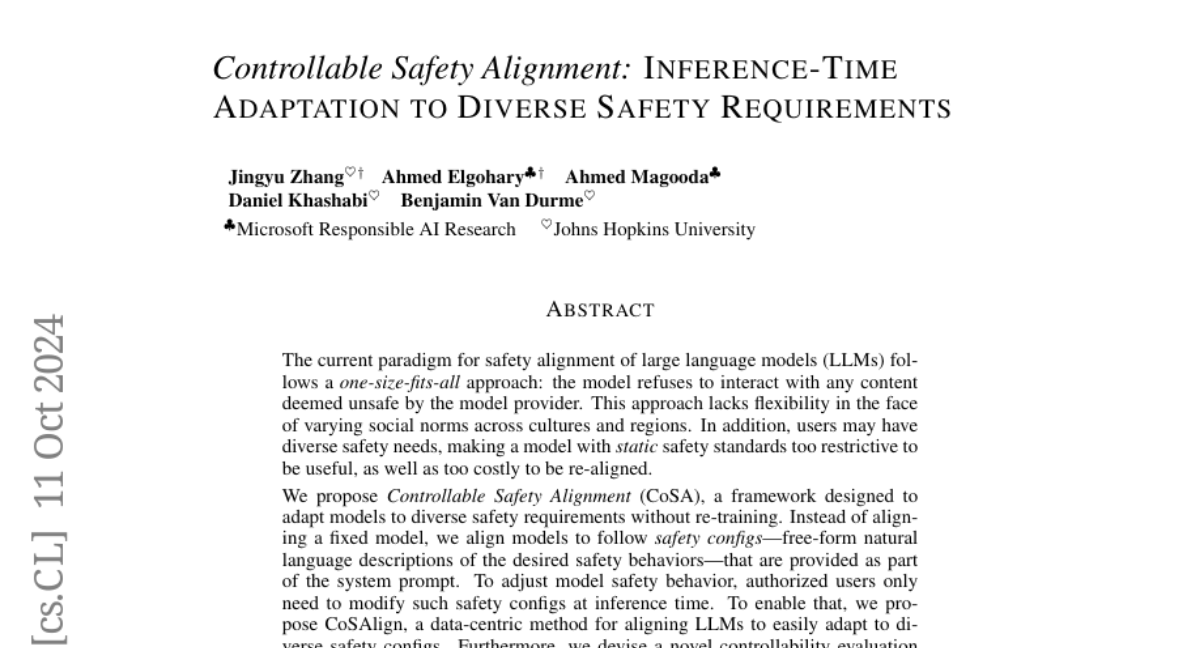
Summary
This paper presents Controllable Safety Alignment (CoSA), a new framework that allows large language models (LLMs) to adapt to different safety requirements without needing to be retrained.
What's the problem?
Current safety measures for LLMs use a one-size-fits-all approach, meaning they apply the same safety rules to all situations. This can be too strict because different cultures and users have varying safety needs. As a result, these models might not be useful in all contexts and can be costly to adjust when safety standards change.
What's the solution?
To solve this problem, the authors propose CoSA, which allows users to specify their own safety requirements using simple natural language descriptions called safety configs. Instead of retraining the entire model, users can adjust these configs at inference time (when the model is being used). The authors also introduce a method called CoSAlign to help the model adapt to these diverse safety needs and create a scoring system (CoSA-Score) to evaluate how well the model meets both helpfulness and safety criteria.
Why it matters?
This research is important because it makes LLMs more flexible and practical for real-world applications. By allowing models to adapt their safety behavior based on user needs, CoSA can improve user satisfaction and ensure that AI systems are safer and more aligned with diverse human values.
Abstract
The current paradigm for safety alignment of large language models (LLMs) follows a one-size-fits-all approach: the model refuses to interact with any content deemed unsafe by the model provider. This approach lacks flexibility in the face of varying social norms across cultures and regions. In addition, users may have diverse safety needs, making a model with static safety standards too restrictive to be useful, as well as too costly to be re-aligned. We propose Controllable Safety Alignment (CoSA), a framework designed to adapt models to diverse safety requirements without re-training. Instead of aligning a fixed model, we align models to follow safety configs -- free-form natural language descriptions of the desired safety behaviors -- that are provided as part of the system prompt. To adjust model safety behavior, authorized users only need to modify such safety configs at inference time. To enable that, we propose CoSAlign, a data-centric method for aligning LLMs to easily adapt to diverse safety configs. Furthermore, we devise a novel controllability evaluation protocol that considers both helpfulness and configured safety, summarizing them into CoSA-Score, and construct CoSApien, a human-authored benchmark that consists of real-world LLM use cases with diverse safety requirements and corresponding evaluation prompts. We show that CoSAlign leads to substantial gains of controllability over strong baselines including in-context alignment. Our framework encourages better representation and adaptation to pluralistic human values in LLMs, and thereby increasing their practicality.