CORAL: Benchmarking Multi-turn Conversational Retrieval-Augmentation Generation
Yiruo Cheng, Kelong Mao, Ziliang Zhao, Guanting Dong, Hongjin Qian, Yongkang Wu, Tetsuya Sakai, Ji-Rong Wen, Zhicheng Dou
2024-10-31
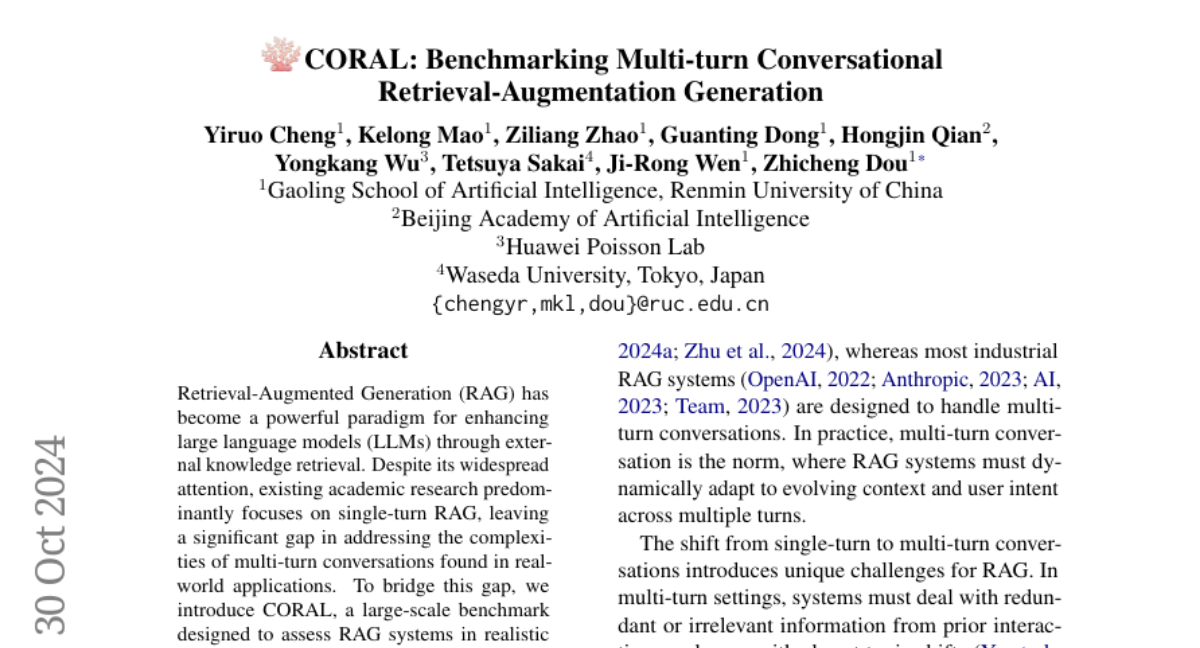
Summary
This paper presents CORAL, a new benchmark designed to evaluate how well Retrieval-Augmented Generation (RAG) systems perform in multi-turn conversations. It aims to improve the way AI systems handle complex dialogues by assessing their ability to retrieve information and generate responses over multiple exchanges.
What's the problem?
Most research on RAG has focused on single-turn interactions, which means that AI systems have not been thoroughly tested on the more complicated task of maintaining a conversation over several turns. This gap makes it difficult for these systems to perform well in real-world applications where conversations are dynamic and require understanding context from previous exchanges.
What's the solution?
CORAL addresses this issue by providing a large-scale benchmark that includes various information-seeking conversations derived from Wikipedia. It evaluates RAG systems on three main tasks: retrieving relevant passages, generating appropriate responses, and labeling citations correctly. The benchmark is designed to test how well these systems can handle open-domain topics, shifts in conversation, and the need for informative responses, thereby helping researchers improve their models.
Why it matters?
This research is important because it helps advance the development of AI systems that can engage in more natural and informative conversations. By creating a standardized way to evaluate multi-turn interactions, CORAL encourages improvements in AI technology that can lead to better applications in customer service, virtual assistants, and other areas where effective communication is crucial.
Abstract
Retrieval-Augmented Generation (RAG) has become a powerful paradigm for enhancing large language models (LLMs) through external knowledge retrieval. Despite its widespread attention, existing academic research predominantly focuses on single-turn RAG, leaving a significant gap in addressing the complexities of multi-turn conversations found in real-world applications. To bridge this gap, we introduce CORAL, a large-scale benchmark designed to assess RAG systems in realistic multi-turn conversational settings. CORAL includes diverse information-seeking conversations automatically derived from Wikipedia and tackles key challenges such as open-domain coverage, knowledge intensity, free-form responses, and topic shifts. It supports three core tasks of conversational RAG: passage retrieval, response generation, and citation labeling. We propose a unified framework to standardize various conversational RAG methods and conduct a comprehensive evaluation of these methods on CORAL, demonstrating substantial opportunities for improving existing approaches.