CosmoCLIP: Generalizing Large Vision-Language Models for Astronomical Imaging
Raza Imam, Mohammed Talha Alam, Umaima Rahman, Mohsen Guizani, Fakhri Karray
2024-07-11
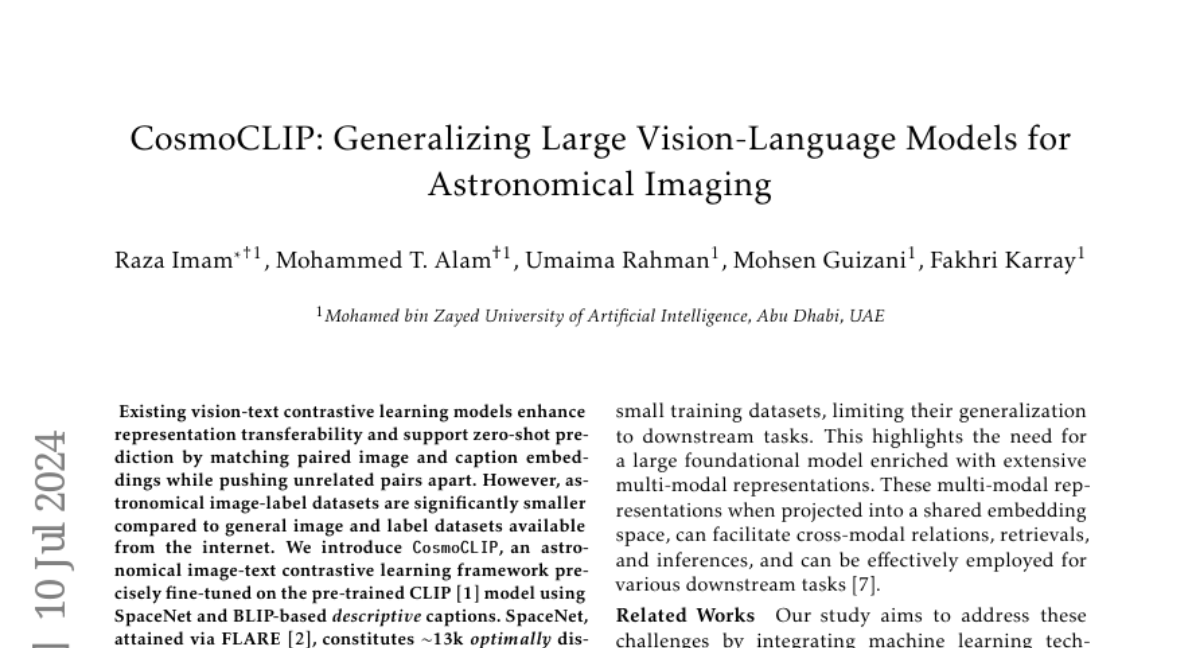
Summary
This paper presents CosmoCLIP, a new framework designed to improve how large vision-language models work with astronomical images. It focuses on enhancing the model's ability to understand and classify these images using text descriptions.
What's the problem?
Most existing models that connect images and text perform well but struggle with astronomical data because there are fewer labeled images available compared to general datasets. This lack of data makes it hard for models to learn effectively and accurately classify astronomical images.
What's the solution?
CosmoCLIP addresses this issue by fine-tuning the pre-trained CLIP model specifically for astronomical images and their captions. It uses a dataset called SpaceNet, which contains about 13,000 well-distributed astronomical images, along with detailed descriptions generated by a tool called BLIP. By training the model to recognize and match these image-text pairs, CosmoCLIP improves its ability to generalize and perform well on both familiar and unfamiliar tasks related to astronomical imaging.
Why it matters?
This research is significant because it enhances the capabilities of AI in the field of astronomy, allowing for better classification and retrieval of astronomical images. By improving how models understand and analyze these images, CosmoCLIP can help scientists make more accurate observations and discoveries in space research.
Abstract
Existing vision-text contrastive learning models enhance representation transferability and support zero-shot prediction by matching paired image and caption embeddings while pushing unrelated pairs apart. However, astronomical image-label datasets are significantly smaller compared to general image and label datasets available from the internet. We introduce CosmoCLIP, an astronomical image-text contrastive learning framework precisely fine-tuned on the pre-trained CLIP model using SpaceNet and BLIP-based captions. SpaceNet, attained via FLARE, constitutes ~13k optimally distributed images, while BLIP acts as a rich knowledge extractor. The rich semantics derived from this SpaceNet and BLIP descriptions, when learned contrastively, enable CosmoCLIP to achieve superior generalization across various in-domain and out-of-domain tasks. Our results demonstrate that CosmoCLIP is a straightforward yet powerful framework, significantly outperforming CLIP in zero-shot classification and image-text retrieval tasks.