CRiM-GS: Continuous Rigid Motion-Aware Gaussian Splatting from Motion Blur Images
Junghe Lee, Donghyeong Kim, Dogyoon Lee, Suhwan Cho, Sangyoun Lee
2024-07-08
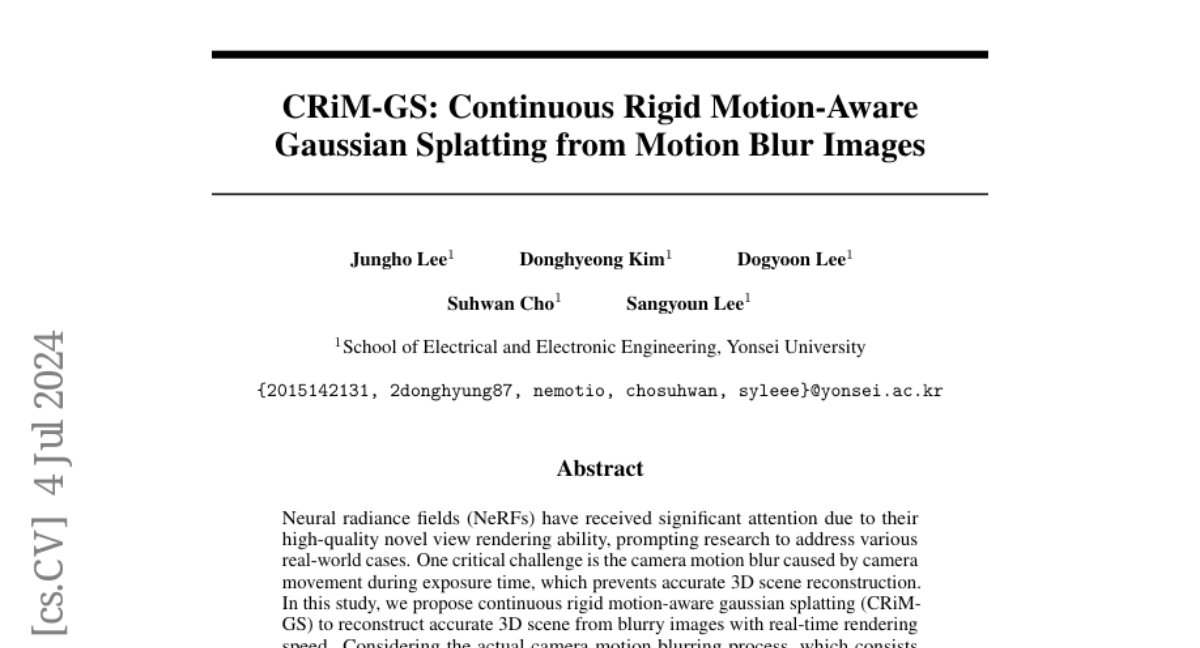
Summary
This paper talks about CRiM-GS, a new method for reconstructing accurate 3D scenes from images that are blurry due to camera motion. It uses advanced techniques to quickly create high-quality 3D images even when the camera has moved during the shot.
What's the problem?
The main problem is that when a camera moves while taking a picture, it can cause motion blur, making it difficult to accurately capture and reconstruct the 3D scene. This blur can lead to poor quality in the final images, which is a significant challenge in fields like photography and computer graphics where clear and precise images are essential.
What's the solution?
To solve this issue, the authors developed CRiM-GS, which stands for Continuous Rigid Motion-Aware Gaussian Splatting. This method predicts how the camera moved during the exposure using mathematical models called neural ordinary differential equations (ODEs). It takes into account the complex patterns of camera motion and uses rigid body transformations to maintain the shape and size of objects in the scene. Additionally, it introduces flexible transformations to better adapt to real-world situations. This allows for accurate modeling of how the camera moved, resulting in clearer 3D reconstructions from blurry images.
Why it matters?
This research is important because it enhances our ability to create high-quality 3D models from images that would otherwise be unusable due to motion blur. By improving how we reconstruct scenes from blurry images, CRiM-GS can benefit various applications such as virtual reality, video games, and film production, where clear and realistic visuals are crucial.
Abstract
Neural radiance fields (NeRFs) have received significant attention due to their high-quality novel view rendering ability, prompting research to address various real-world cases. One critical challenge is the camera motion blur caused by camera movement during exposure time, which prevents accurate 3D scene reconstruction. In this study, we propose continuous rigid motion-aware gaussian splatting (CRiM-GS) to reconstruct accurate 3D scene from blurry images with real-time rendering speed. Considering the actual camera motion blurring process, which consists of complex motion patterns, we predict the continuous movement of the camera based on neural ordinary differential equations (ODEs). Specifically, we leverage rigid body transformations to model the camera motion with proper regularization, preserving the shape and size of the object. Furthermore, we introduce a continuous deformable 3D transformation in the SE(3) field to adapt the rigid body transformation to real-world problems by ensuring a higher degree of freedom. By revisiting fundamental camera theory and employing advanced neural network training techniques, we achieve accurate modeling of continuous camera trajectories. We conduct extensive experiments, demonstrating state-of-the-art performance both quantitatively and qualitatively on benchmark datasets.