CrossViewDiff: A Cross-View Diffusion Model for Satellite-to-Street View Synthesis
Weijia Li, Jun He, Junyan Ye, Huaping Zhong, Zhimeng Zheng, Zilong Huang, Dahua Lin, Conghui He
2024-09-02
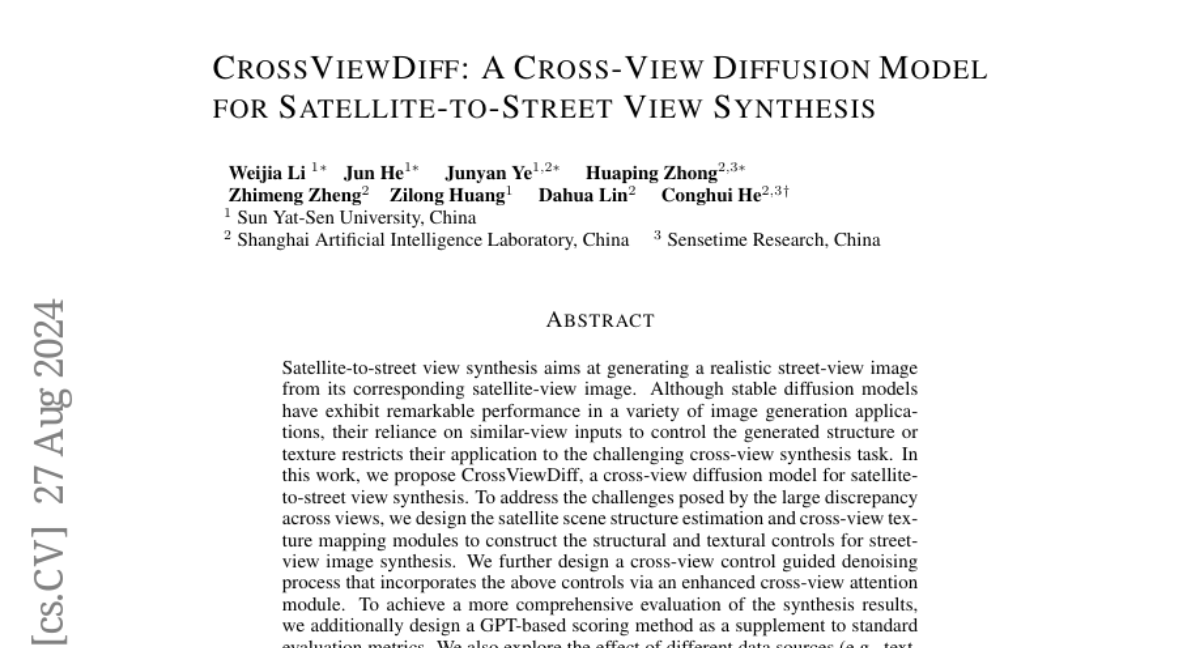
Summary
This paper talks about CrossViewDiff, a new model that generates realistic street-view images from satellite images by overcoming challenges related to different perspectives.
What's the problem?
Creating street-view images from satellite images is difficult because the two views are very different. Most existing models work well only when the input images are similar, which limits their effectiveness in this cross-view synthesis task.
What's the solution?
CrossViewDiff addresses this issue by using two main components: one that estimates the structure of the satellite scene and another that maps textures from the satellite view to the street view. It also incorporates a special attention mechanism to improve how these components work together. To evaluate its performance, the authors created a new scoring method based on GPT, in addition to standard metrics, to ensure a thorough assessment of the generated images.
Why it matters?
This research is important because it improves our ability to generate high-quality street-view images, which can be useful for various applications like urban planning, navigation systems, and virtual reality. By successfully bridging the gap between satellite and street views, CrossViewDiff enhances how we visualize and understand urban environments.
Abstract
Satellite-to-street view synthesis aims at generating a realistic street-view image from its corresponding satellite-view image. Although stable diffusion models have exhibit remarkable performance in a variety of image generation applications, their reliance on similar-view inputs to control the generated structure or texture restricts their application to the challenging cross-view synthesis task. In this work, we propose CrossViewDiff, a cross-view diffusion model for satellite-to-street view synthesis. To address the challenges posed by the large discrepancy across views, we design the satellite scene structure estimation and cross-view texture mapping modules to construct the structural and textural controls for street-view image synthesis. We further design a cross-view control guided denoising process that incorporates the above controls via an enhanced cross-view attention module. To achieve a more comprehensive evaluation of the synthesis results, we additionally design a GPT-based scoring method as a supplement to standard evaluation metrics. We also explore the effect of different data sources (e.g., text, maps, building heights, and multi-temporal satellite imagery) on this task. Results on three public cross-view datasets show that CrossViewDiff outperforms current state-of-the-art on both standard and GPT-based evaluation metrics, generating high-quality street-view panoramas with more realistic structures and textures across rural, suburban, and urban scenes. The code and models of this work will be released at https://opendatalab.github.io/CrossViewDiff/.