Crowdsource, Crawl, or Generate? Creating SEA-VL, a Multicultural Vision-Language Dataset for Southeast Asia
Samuel Cahyawijaya, Holy Lovenia, Joel Ruben Antony Moniz, Tack Hwa Wong, Mohammad Rifqi Farhansyah, Thant Thiri Maung, Frederikus Hudi, David Anugraha, Muhammad Ravi Shulthan Habibi, Muhammad Reza Qorib, Amit Agarwal, Joseph Marvin Imperial, Hitesh Laxmichand Patel, Vicky Feliren, Bahrul Ilmi Nasution, Manuel Antonio Rufino, Genta Indra Winata, Rian Adam Rajagede, Carlos Rafael Catalan, Mohamed Fazli Imam, Priyaranjan Pattnayak, Salsabila Zahirah Pranida
2025-03-12
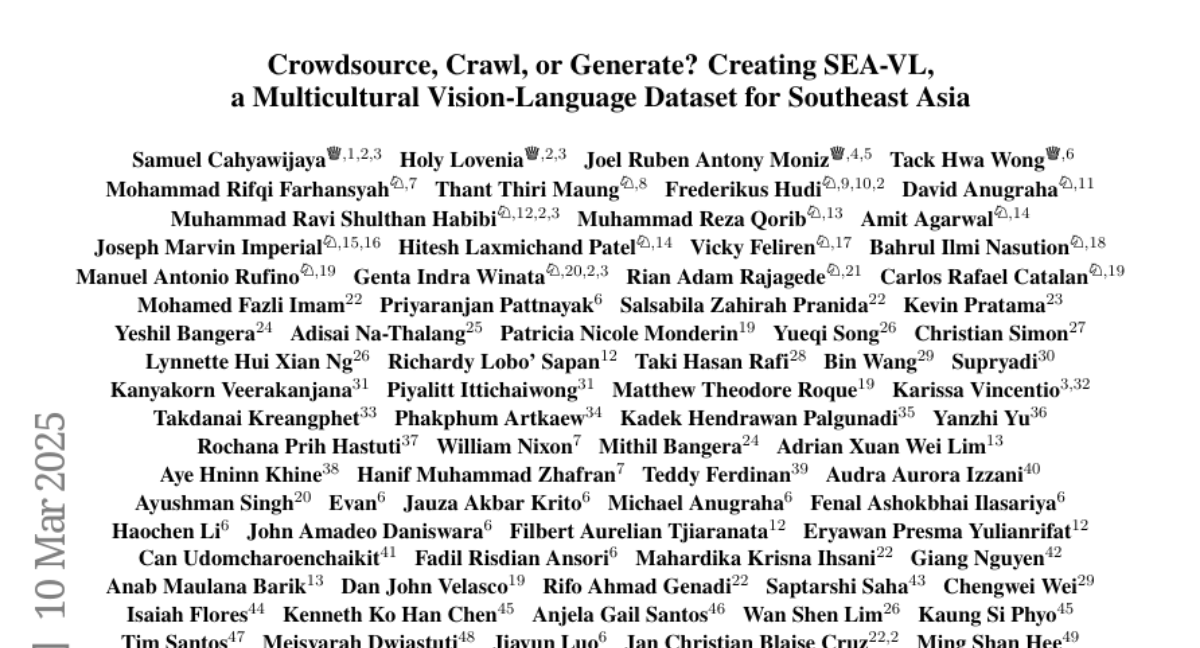
Summary
This paper talks about SEA-VL, a project that collects millions of pictures and descriptions from Southeast Asia to help AI understand the region’s cultures better, since most AI tools don’t know enough about them.
What's the problem?
AI models struggle with Southeast Asian languages and cultures because they’re trained mostly on Western data, leading to mistakes like missing local traditions or misidentifying objects.
What's the solution?
SEA-VL gathers real photos from locals, crawls the web for relevant images, and tries AI-generated pictures, but found real photos work best. They built a huge dataset with 1.28 million images to train better AI.
Why it matters?
This helps create fairer AI tools for things like translation or image search in Southeast Asia, so they respect local cultures instead of favoring Western ones.
Abstract
Southeast Asia (SEA) is a region of extraordinary linguistic and cultural diversity, yet it remains significantly underrepresented in vision-language (VL) research. This often results in artificial intelligence (AI) models that fail to capture SEA cultural nuances. To fill this gap, we present SEA-VL, an open-source initiative dedicated to developing high-quality, culturally relevant data for SEA languages. By involving contributors from SEA countries, SEA-VL aims to ensure better cultural relevance and diversity, fostering greater inclusivity of underrepresented languages in VL research. Beyond crowdsourcing, our initiative goes one step further in the exploration of the automatic collection of culturally relevant images through crawling and image generation. First, we find that image crawling achieves approximately ~85% cultural relevance while being more cost- and time-efficient than crowdsourcing. Second, despite the substantial progress in generative vision models, synthetic images remain unreliable in accurately reflecting SEA cultures. The generated images often fail to reflect the nuanced traditions and cultural contexts of the region. Collectively, we gather 1.28M SEA culturally-relevant images, more than 50 times larger than other existing datasets. Through SEA-VL, we aim to bridge the representation gap in SEA, fostering the development of more inclusive AI systems that authentically represent diverse cultures across SEA.