CS-Bench: A Comprehensive Benchmark for Large Language Models towards Computer Science Mastery
Xiaoshuai Song, Muxi Diao, Guanting Dong, Zhengyang Wang, Yujia Fu, Runqi Qiao, Zhexu Wang, Dayuan Fu, Huangxuan Wu, Bin Liang, Weihao Zeng, Yejie Wang, Zhuoma GongQue, Jianing Yu, Qiuna Tan, Weiran Xu
2024-06-14
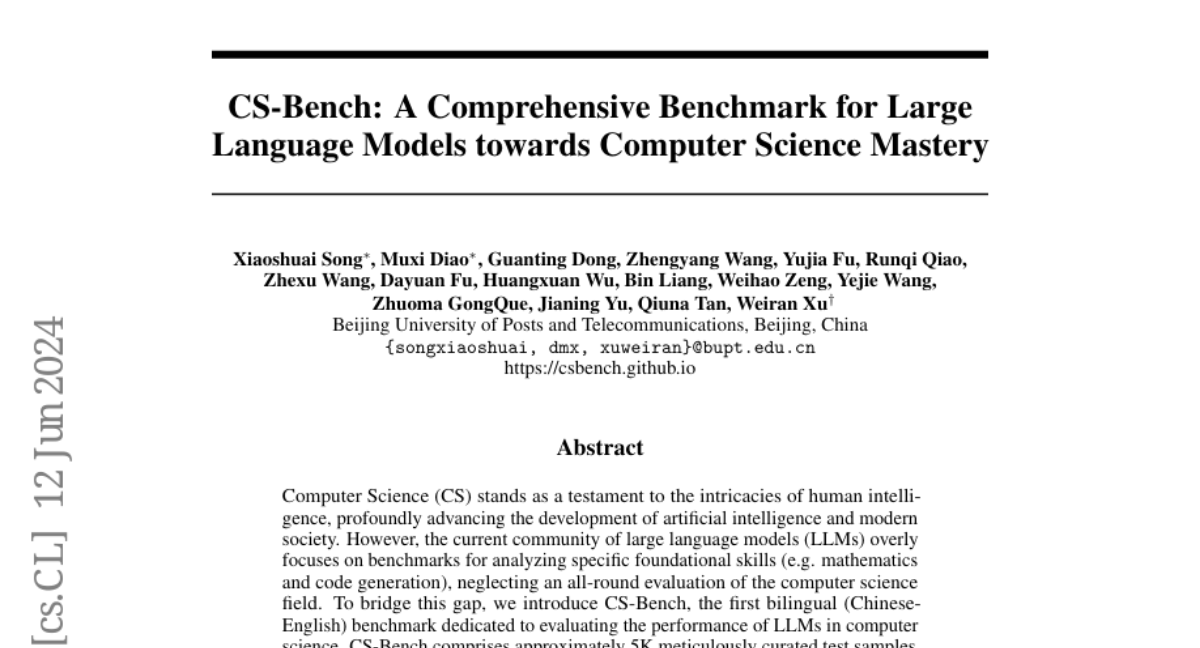
Summary
This paper introduces CS-Bench, a new benchmark designed to evaluate how well large language models (LLMs) understand and perform tasks in computer science. It is the first bilingual benchmark that assesses LLMs in both Chinese and English across various areas of computer science.
What's the problem?
Currently, most benchmarks for LLMs focus on specific skills, like mathematics or coding, without providing a comprehensive evaluation of their abilities in the broader field of computer science. This means that while LLMs may be good at certain tasks, we lack a clear understanding of how well they can apply their knowledge across different areas of computer science.
What's the solution?
CS-Bench addresses this issue by providing around 5,000 carefully selected test samples that cover 26 subfields within four main areas of computer science. The benchmark includes a variety of question types to assess both knowledge and reasoning abilities. The authors evaluated over 30 popular LLMs using CS-Bench and analyzed their performance to identify strengths and weaknesses. They found that there is a strong connection between how well LLMs perform in computer science and their abilities in mathematics and coding.
Why it matters?
This research is important because it helps improve our understanding of how LLMs can be applied in the field of computer science. By creating a comprehensive evaluation tool like CS-Bench, researchers can better assess and enhance the capabilities of these models, ultimately leading to more effective AI applications in technology and education. Additionally, making the data and evaluation code available encourages further research and development in this area.
Abstract
Computer Science (CS) stands as a testament to the intricacies of human intelligence, profoundly advancing the development of artificial intelligence and modern society. However, the current community of large language models (LLMs) overly focuses on benchmarks for analyzing specific foundational skills (e.g. mathematics and code generation), neglecting an all-round evaluation of the computer science field. To bridge this gap, we introduce CS-Bench, the first bilingual (Chinese-English) benchmark dedicated to evaluating the performance of LLMs in computer science. CS-Bench comprises approximately 5K meticulously curated test samples, covering 26 subfields across 4 key areas of computer science, encompassing various task forms and divisions of knowledge and reasoning. Utilizing CS-Bench, we conduct a comprehensive evaluation of over 30 mainstream LLMs, revealing the relationship between CS performance and model scales. We also quantitatively analyze the reasons for failures in existing LLMs and highlight directions for improvements, including knowledge supplementation and CS-specific reasoning. Further cross-capability experiments show a high correlation between LLMs' capabilities in computer science and their abilities in mathematics and coding. Moreover, expert LLMs specialized in mathematics and coding also demonstrate strong performances in several CS subfields. Looking ahead, we envision CS-Bench serving as a cornerstone for LLM applications in the CS field and paving new avenues in assessing LLMs' diverse reasoning capabilities. The CS-Bench data and evaluation code are available at https://github.com/csbench/csbench.