CURLoRA: Stable LLM Continual Fine-Tuning and Catastrophic Forgetting Mitigation
Muhammad Fawi
2024-09-02
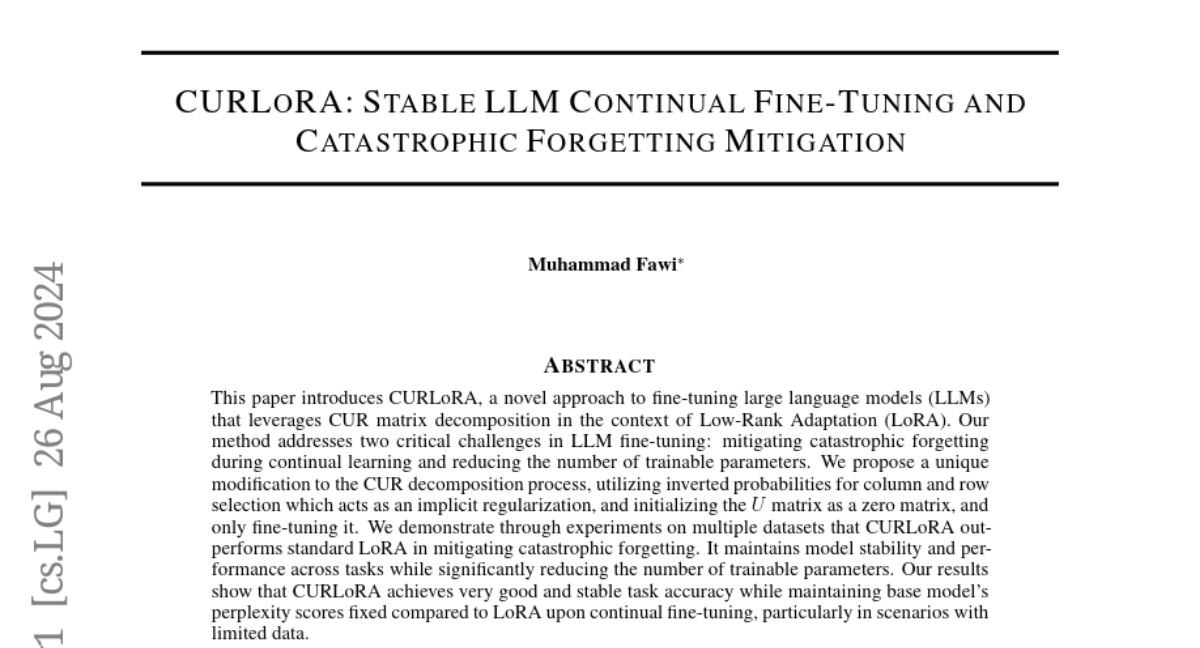
Summary
This paper introduces CURLoRA, a new method for fine-tuning large language models (LLMs) that helps prevent them from forgetting what they've learned while also reducing the number of parameters that need training.
What's the problem?
When large language models are continuously trained on new information, they often forget previously learned information, which is known as catastrophic forgetting. Additionally, traditional fine-tuning methods can require a lot of computational resources because they involve adjusting many parameters.
What's the solution?
CURLoRA uses a technique called CUR matrix decomposition in combination with Low-Rank Adaptation (LoRA) to address these issues. It modifies how the model selects and organizes data during training, which helps maintain stability and performance across different tasks. By focusing on only fine-tuning certain parts of the model (the U matrix) while keeping others fixed, CURLoRA reduces the number of parameters that need to be trained.
Why it matters?
This research is important because it improves the way we can train large language models without losing their previous knowledge. This can lead to more efficient models that are easier to use in various applications, making them more accessible for researchers and developers working on different tasks.
Abstract
This paper introduces CURLoRA, a novel approach to fine-tuning large language models (LLMs) that leverages CUR matrix decomposition in the context of Low-Rank Adaptation (LoRA). Our method addresses two critical challenges in LLM fine-tuning: mitigating catastrophic forgetting during continual learning and reducing the number of trainable parameters. We propose a unique modification to the CUR decomposition process, utilizing inverted probabilities for column and row selection which acts as an implicit regularization, and initializing the U matrix as a zero matrix, and only fine-tuning it. We demonstrate through experiments on multiple datasets that CURLoRA outperforms standard LoRA in mitigating catastrophic forgetting. It maintains model stability and performance across tasks while significantly reducing the number of trainable parameters. Our results show that CURLoRA achieves very good and stable task accuracy while maintaining base model's perplexity scores fixed compared to LoRA upon continual fine-tuning, particularly in scenarios with limited data.