Dallah: A Dialect-Aware Multimodal Large Language Model for Arabic
Fakhraddin Alwajih, Gagan Bhatia, Muhammad Abdul-Mageed
2024-07-26
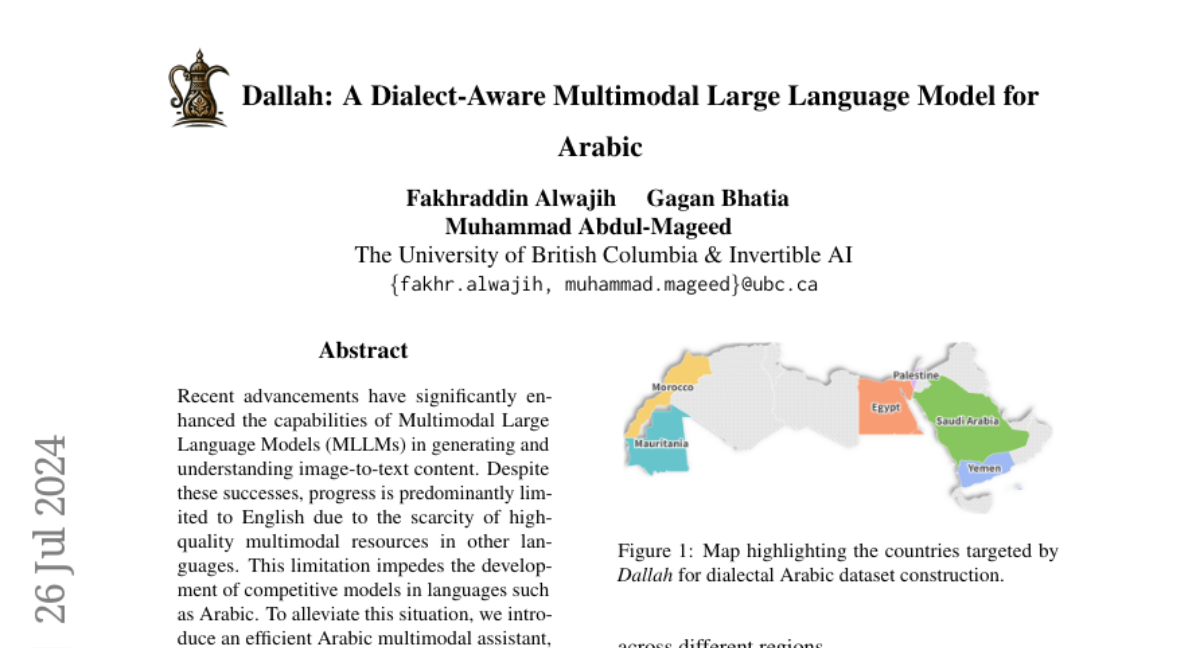
Summary
This paper introduces Dallah, a new multimodal language model designed specifically for the Arabic language. It can understand and generate content in various Arabic dialects, making it more effective for users who speak different forms of Arabic.
What's the problem?
Most language models have been primarily developed for English and struggle with other languages, especially those with many dialects like Arabic. This lack of resources means that models often don't perform well when trying to understand or generate content in Arabic, which has many regional variations that are important for effective communication.
What's the solution?
Dallah addresses this problem by being trained on a diverse dataset that includes both text and images from multiple Arabic dialects. It uses an advanced model based on LLaMA-2 and has been fine-tuned to handle complex interactions involving both text and visuals. Dallah performs well in tests that assess its understanding of Modern Standard Arabic (the formal version) as well as various dialects, showcasing its ability to interact naturally with users across different regions.
Why it matters?
This research is important because it enhances the capabilities of AI in understanding and generating Arabic content, which can improve communication and accessibility for Arabic speakers. By being dialect-aware, Dallah can help preserve the richness of the Arabic language and make technology more inclusive for diverse users.
Abstract
Recent advancements have significantly enhanced the capabilities of Multimodal Large Language Models (MLLMs) in generating and understanding image-to-text content. Despite these successes, progress is predominantly limited to English due to the scarcity of high quality multimodal resources in other languages. This limitation impedes the development of competitive models in languages such as Arabic. To alleviate this situation, we introduce an efficient Arabic multimodal assistant, dubbed Dallah, that utilizes an advanced language model based on LLaMA-2 to facilitate multimodal interactions. Dallah demonstrates state-of-the-art performance in Arabic MLLMs. Through fine-tuning six Arabic dialects, Dallah showcases its capability to handle complex dialectal interactions incorporating both textual and visual elements. The model excels in two benchmark tests: one evaluating its performance on Modern Standard Arabic (MSA) and another specifically designed to assess dialectal responses. Beyond its robust performance in multimodal interaction tasks, Dallah has the potential to pave the way for further development of dialect-aware Arabic MLLMs.