Data Contamination Can Cross Language Barriers
Feng Yao, Yufan Zhuang, Zihao Sun, Sunan Xu, Animesh Kumar, Jingbo Shang
2024-06-24
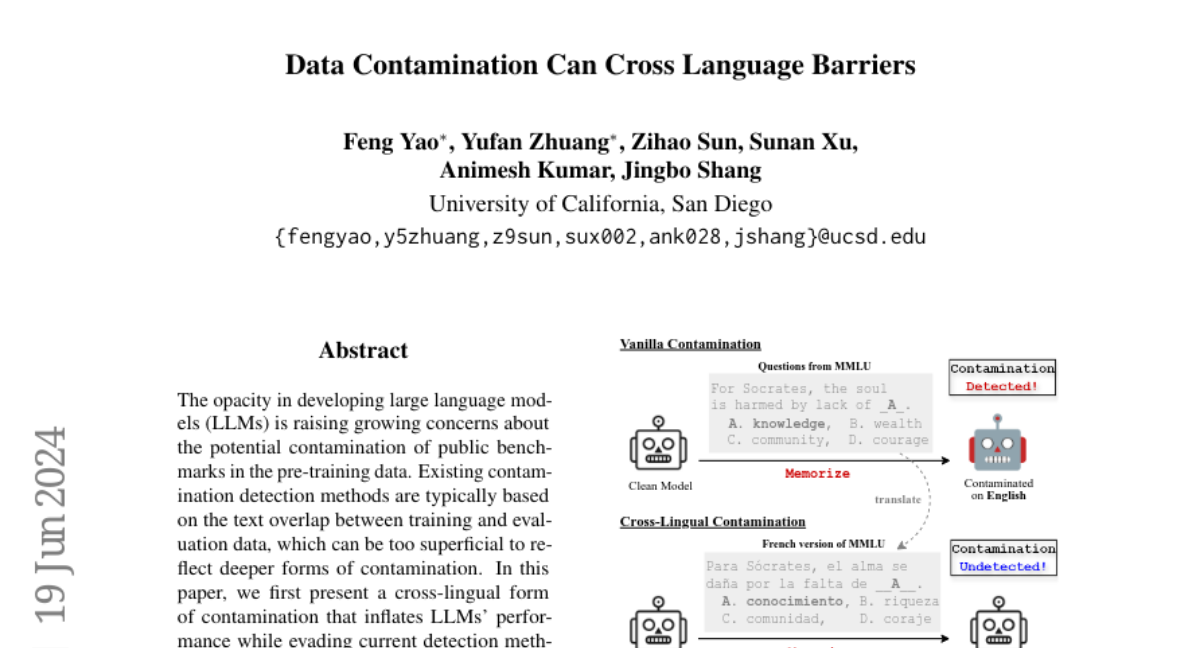
Summary
This paper explores how large language models (LLMs) can be affected by a type of data contamination that crosses language barriers, which makes them seem more accurate than they actually are. It highlights the limitations of current methods for detecting this problem and proposes new approaches to uncover it.
What's the problem?
As LLMs are trained on large datasets, there is a risk that these datasets may contain overlapping or contaminated information, which can lead to misleading performance results. Current detection methods often only look for simple overlaps in text between training and testing data, which may not catch deeper issues of contamination, especially when translations are involved.
What's the solution?
The authors introduce a new way to identify this cross-lingual contamination by testing how well LLMs perform when the original benchmark questions are altered. They replace incorrect answer choices with correct ones from different questions to see if the model can still perform well. Their experiments show that contaminated models struggle to adapt to these changes, revealing the hidden contamination that other methods fail to detect.
Why it matters?
Understanding and addressing cross-lingual contamination is crucial for improving the reliability of LLMs. By developing better detection methods, researchers can ensure that these models are truly effective and trustworthy, especially in multilingual contexts. This research could lead to advancements in how LLMs are trained and evaluated, enhancing their performance across different languages.
Abstract
The opacity in developing large language models (LLMs) is raising growing concerns about the potential contamination of public benchmarks in the pre-training data. Existing contamination detection methods are typically based on the text overlap between training and evaluation data, which can be too superficial to reflect deeper forms of contamination. In this paper, we first present a cross-lingual form of contamination that inflates LLMs' performance while evading current detection methods, deliberately injected by overfitting LLMs on the translated versions of benchmark test sets. Then, we propose generalization-based approaches to unmask such deeply concealed contamination. Specifically, we examine the LLM's performance change after modifying the original benchmark by replacing the false answer choices with correct ones from other questions. Contaminated models can hardly generalize to such easier situations, where the false choices can be not even wrong, as all choices are correct in their memorization. Experimental results demonstrate that cross-lingual contamination can easily fool existing detection methods, but not ours. In addition, we discuss the potential utilization of cross-lingual contamination in interpreting LLMs' working mechanisms and in post-training LLMs for enhanced multilingual capabilities. The code and dataset we use can be obtained from https://github.com/ShangDataLab/Deep-Contam.