Data Contamination Report from the 2024 CONDA Shared Task
Oscar Sainz, Iker García-Ferrero, Alon Jacovi, Jon Ander Campos, Yanai Elazar, Eneko Agirre, Yoav Goldberg, Wei-Lin Chen, Jenny Chim, Leshem Choshen, Luca D'Amico-Wong, Melissa Dell, Run-Ze Fan, Shahriar Golchin, Yucheng Li, Pengfei Liu, Bhavish Pahwa, Ameya Prabhu, Suryansh Sharma, Emily Silcock, Kateryna Solonko, David Stap
2024-08-01
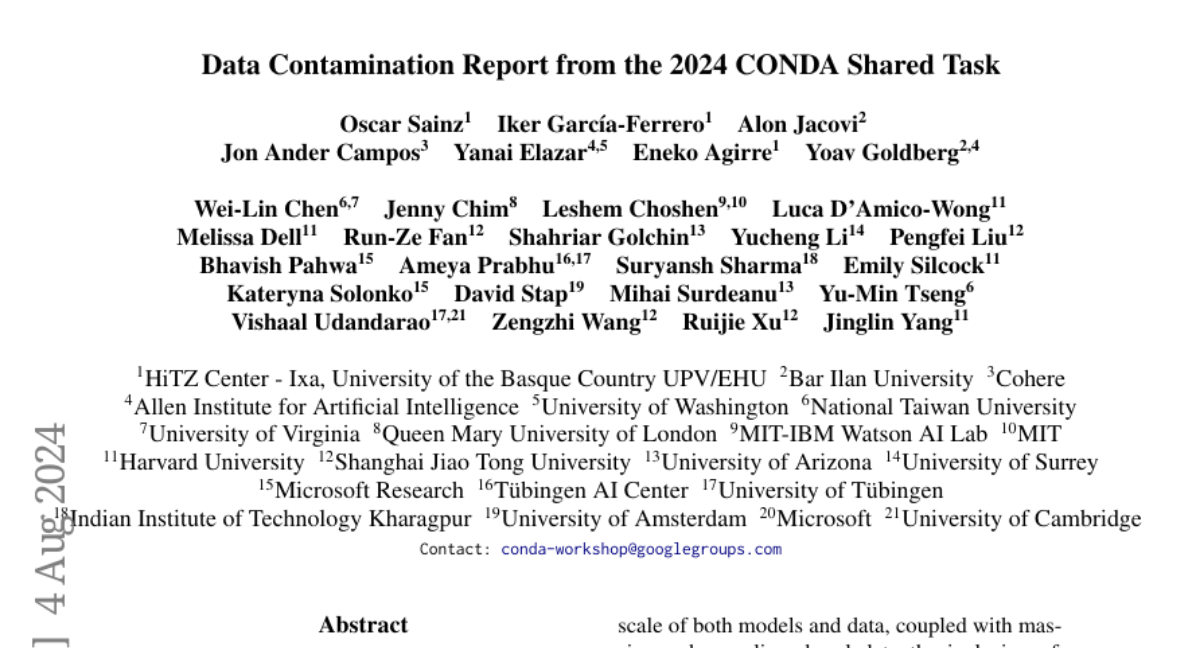
Summary
This paper discusses the findings from the 2024 CONDA workshop on data contamination, which focuses on how including evaluation data in training sets can lead to inaccurate results in natural language processing models.
What's the problem?
Data contamination occurs when the data used to evaluate AI models accidentally includes information that was also used to train those models. This can make it seem like the models perform better than they actually do, as they may have 'seen' the evaluation data before. This is a significant issue because it can mislead researchers and users about the true capabilities of these models.
What's the solution?
To address this problem, the CONDA workshop organized a shared task where researchers could report instances of data contamination. The paper compiles evidence from 566 reported cases across 91 different sources, helping to create a centralized database that tracks contamination incidents. This allows the research community to better understand the extent of the problem and avoid using contaminated datasets in future evaluations.
Why it matters?
This research is crucial because it helps improve the integrity and reliability of AI models. By highlighting and documenting instances of data contamination, researchers can work towards creating cleaner datasets and more accurate evaluations. This ultimately leads to better AI systems that can be trusted to perform well in real-world applications.
Abstract
The 1st Workshop on Data Contamination (CONDA 2024) focuses on all relevant aspects of data contamination in natural language processing, where data contamination is understood as situations where evaluation data is included in pre-training corpora used to train large scale models, compromising evaluation results. The workshop fostered a shared task to collect evidence on data contamination in current available datasets and models. The goal of the shared task and associated database is to assist the community in understanding the extent of the problem and to assist researchers in avoiding reporting evaluation results on known contaminated resources. The shared task provides a structured, centralized public database for the collection of contamination evidence, open to contributions from the community via GitHub pool requests. This first compilation paper is based on 566 reported entries over 91 contaminated sources from a total of 23 contributors. The details of the individual contamination events are available in the platform. The platform continues to be online, open to contributions from the community.