Data Scaling Laws in Imitation Learning for Robotic Manipulation
Fanqi Lin, Yingdong Hu, Pingyue Sheng, Chuan Wen, Jiacheng You, Yang Gao
2024-10-25
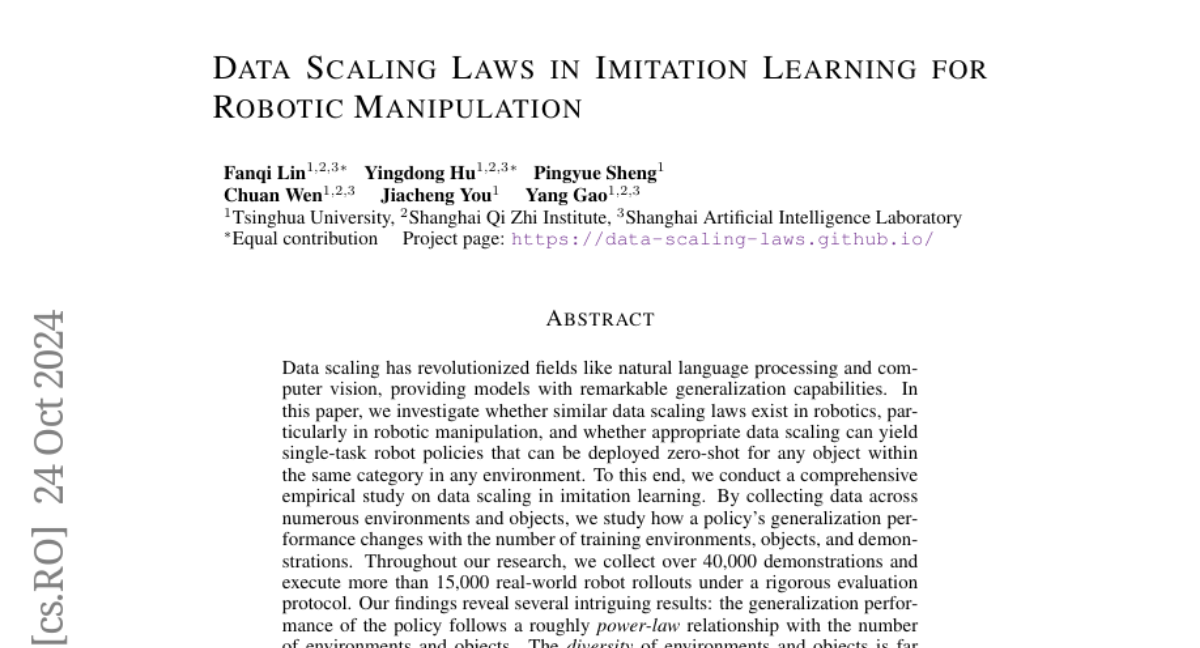
Summary
This paper investigates how data scaling laws can improve imitation learning in robotic manipulation, focusing on how the amount and diversity of training data affect a robot's ability to perform tasks.
What's the problem?
In fields like natural language processing and computer vision, researchers have found that having more data generally leads to better model performance. However, it's unclear if the same rules apply to robotics, especially for tasks where robots need to learn from examples, like pouring water or arranging objects. Existing methods may not effectively consider how different types of training environments and objects impact a robot's ability to generalize its skills.
What's the solution?
The authors conducted extensive experiments by collecting over 40,000 demonstrations of robots performing tasks in various environments and with different objects. They discovered that the diversity of these environments and objects is more important than just having a large number of demonstrations. Once a certain number of demonstrations is reached, adding more does not significantly improve performance. Based on these insights, they developed a strategy for efficiently gathering data that allows robots to achieve high success rates in new situations with previously unseen objects.
Why it matters?
This research is important because it provides valuable insights into how robots can learn more effectively from fewer but more diverse examples. By understanding the relationship between data diversity and performance, researchers can improve robotic systems, making them more adaptable and efficient in real-world applications.
Abstract
Data scaling has revolutionized fields like natural language processing and computer vision, providing models with remarkable generalization capabilities. In this paper, we investigate whether similar data scaling laws exist in robotics, particularly in robotic manipulation, and whether appropriate data scaling can yield single-task robot policies that can be deployed zero-shot for any object within the same category in any environment. To this end, we conduct a comprehensive empirical study on data scaling in imitation learning. By collecting data across numerous environments and objects, we study how a policy's generalization performance changes with the number of training environments, objects, and demonstrations. Throughout our research, we collect over 40,000 demonstrations and execute more than 15,000 real-world robot rollouts under a rigorous evaluation protocol. Our findings reveal several intriguing results: the generalization performance of the policy follows a roughly power-law relationship with the number of environments and objects. The diversity of environments and objects is far more important than the absolute number of demonstrations; once the number of demonstrations per environment or object reaches a certain threshold, additional demonstrations have minimal effect. Based on these insights, we propose an efficient data collection strategy. With four data collectors working for one afternoon, we collect sufficient data to enable the policies for two tasks to achieve approximately 90% success rates in novel environments with unseen objects.