DC3DO: Diffusion Classifier for 3D Objects
Nursena Koprucu, Meher Shashwat Nigam, Shicheng Xu, Biruk Abere, Gabriele Dominici, Andrew Rodriguez, Sharvaree Vadgam, Berfin Inal, Alberto Tono
2024-08-14
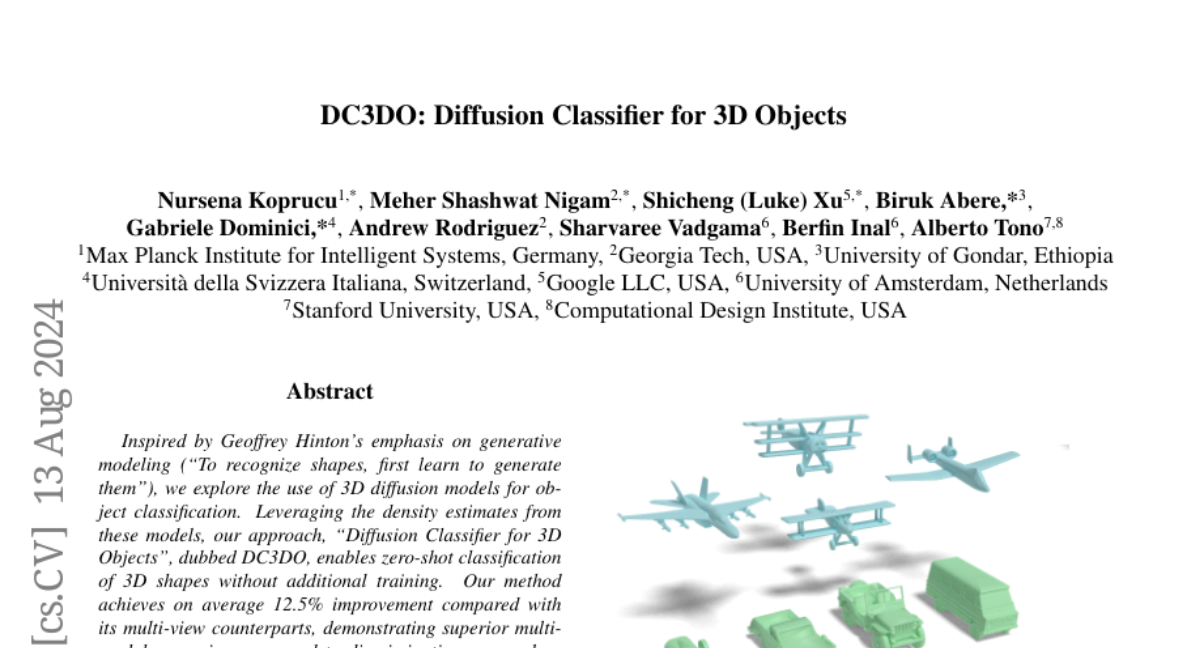
Summary
This paper discusses DC3DO, a new method for classifying 3D objects using diffusion models, allowing the model to recognize shapes without needing extra training.
What's the problem?
Classifying 3D objects can be difficult because traditional methods often require a lot of examples for each type of object to learn from. This means that if a model hasn't seen a specific shape before, it might struggle to identify it correctly.
What's the solution?
The authors developed DC3DO (Diffusion Classifier for 3D Objects), which uses a technique called diffusion modeling to learn how to generate and classify 3D shapes. This approach allows the model to classify new shapes it has never seen before, known as zero-shot classification. The model was trained on a large dataset called ShapeNet, focusing on identifying objects like chairs and cars. The results showed that DC3DO outperformed other existing methods by an average of 12.5% in accuracy.
Why it matters?
This research is important because it demonstrates how generative models can be applied to 3D object classification, making it easier and more efficient to identify various shapes without needing extensive training data. This could have significant implications for fields like robotics, computer graphics, and virtual reality, where understanding and recognizing objects in three dimensions is crucial.
Abstract
Inspired by Geoffrey Hinton emphasis on generative modeling, To recognize shapes, first learn to generate them, we explore the use of 3D diffusion models for object classification. Leveraging the density estimates from these models, our approach, the Diffusion Classifier for 3D Objects (DC3DO), enables zero-shot classification of 3D shapes without additional training. On average, our method achieves a 12.5 percent improvement compared to its multiview counterparts, demonstrating superior multimodal reasoning over discriminative approaches. DC3DO employs a class-conditional diffusion model trained on ShapeNet, and we run inferences on point clouds of chairs and cars. This work highlights the potential of generative models in 3D object classification.