Decoding Reading Goals from Eye Movements
Omer Shubi, Cfir Avraham Hadar, Yevgeni Berzak
2024-10-31
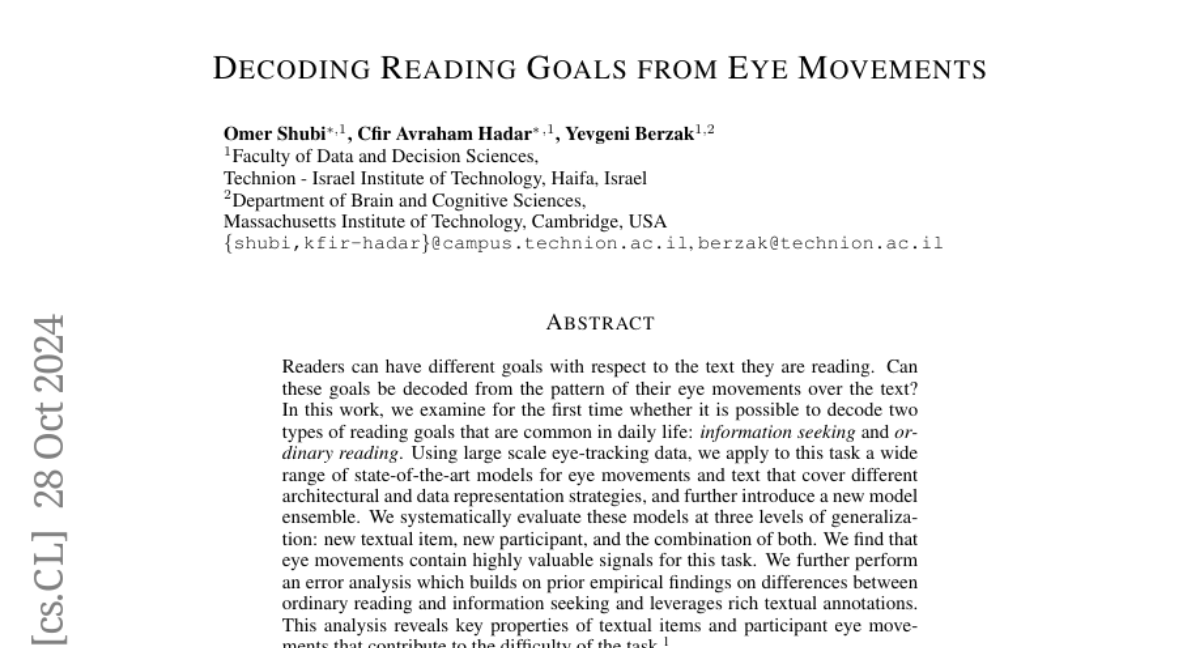
Summary
This paper investigates whether we can understand a reader's goals based on their eye movements while reading, specifically focusing on two common reading goals: seeking information and ordinary reading.
What's the problem?
Readers often have different goals when they read, and these goals can affect how they move their eyes over the text. However, it's unclear if we can accurately decode these goals just by looking at the patterns of eye movements. Previous studies haven't thoroughly explored how eye movements relate to different reading objectives, which makes it hard to understand how readers process information.
What's the solution?
The authors use a large dataset of eye-tracking data to analyze eye movements associated with two types of reading goals: information seeking and ordinary reading. They apply various advanced models to examine these movements and introduce a new model ensemble to improve accuracy. The study evaluates how well these models can generalize to new texts and different readers, finding that eye movements contain valuable signals that can help identify the reader's goal. They also conduct an error analysis to understand the challenges in decoding these goals based on eye movement patterns.
Why it matters?
This research is important because it helps us better understand how readers interact with text based on their goals. By decoding reading intentions from eye movements, we can improve educational tools and reading aids, making it easier for students and researchers to analyze reading strategies and enhance comprehension skills.
Abstract
Readers can have different goals with respect to the text they are reading. Can these goals be decoded from the pattern of their eye movements over the text? In this work, we examine for the first time whether it is possible to decode two types of reading goals that are common in daily life: information seeking and ordinary reading. Using large scale eye-tracking data, we apply to this task a wide range of state-of-the-art models for eye movements and text that cover different architectural and data representation strategies, and further introduce a new model ensemble. We systematically evaluate these models at three levels of generalization: new textual item, new participant, and the combination of both. We find that eye movements contain highly valuable signals for this task. We further perform an error analysis which builds on prior empirical findings on differences between ordinary reading and information seeking and leverages rich textual annotations. This analysis reveals key properties of textual items and participant eye movements that contribute to the difficulty of the task.