DeepSeek-Prover-V1.5: Harnessing Proof Assistant Feedback for Reinforcement Learning and Monte-Carlo Tree Search
Huajian Xin, Z. Z. Ren, Junxiao Song, Zhihong Shao, Wanjia Zhao, Haocheng Wang, Bo Liu, Liyue Zhang, Xuan Lu, Qiushi Du, Wenjun Gao, Qihao Zhu, Dejian Yang, Zhibin Gou, Z. F. Wu, Fuli Luo, Chong Ruan
2024-08-16
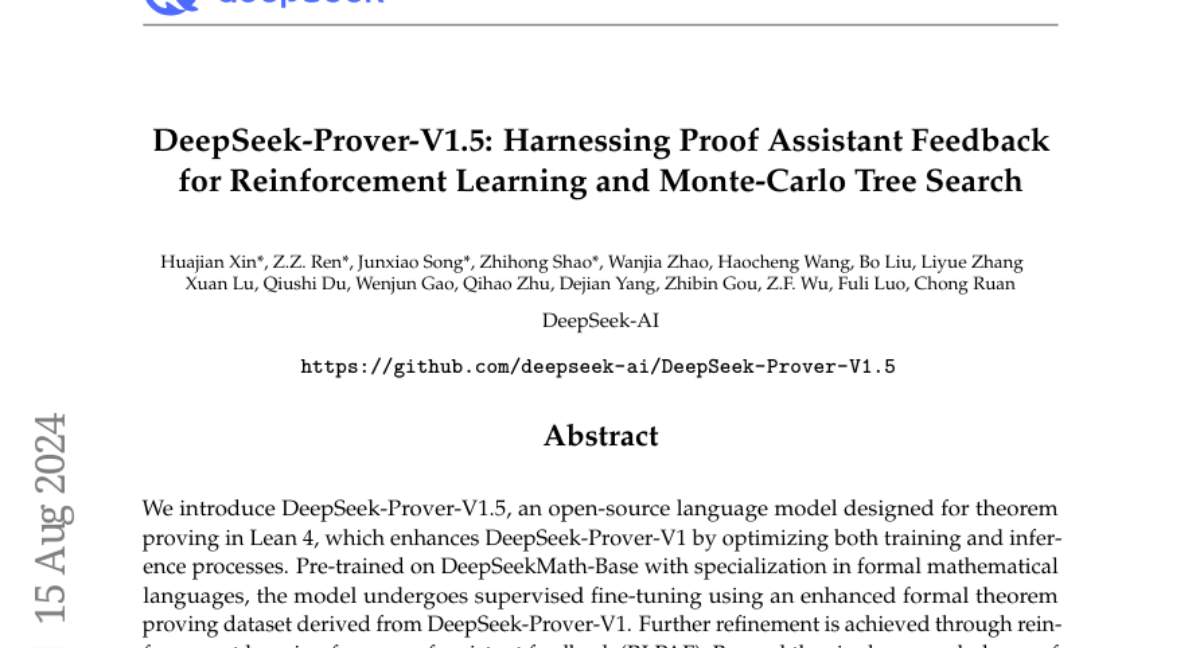
Summary
This paper introduces DeepSeek-Prover-V1.5, an advanced open-source language model designed to help prove mathematical theorems more effectively using feedback from proof assistants.
What's the problem?
The challenge with theorem proving is that existing models often struggle with efficiency and accuracy. They typically rely on large amounts of data and can be slow in generating proofs, which limits their usefulness in practical applications.
What's the solution?
DeepSeek-Prover-V1.5 improves upon its predecessor by optimizing the training process and using a special technique called reinforcement learning from proof assistant feedback (RLPAF). This model not only generates proofs more efficiently but also incorporates a new approach called RMaxTS, which enhances the way it explores different proof paths. As a result, it achieves better performance on benchmark tests for both high school and undergraduate levels.
Why it matters?
This research is important because it advances the field of automated theorem proving, making it easier and faster to verify mathematical statements. By improving these models, it can aid students and researchers in mathematics, potentially leading to new discoveries and a deeper understanding of complex concepts.
Abstract
We introduce DeepSeek-Prover-V1.5, an open-source language model designed for theorem proving in Lean 4, which enhances DeepSeek-Prover-V1 by optimizing both training and inference processes. Pre-trained on DeepSeekMath-Base with specialization in formal mathematical languages, the model undergoes supervised fine-tuning using an enhanced formal theorem proving dataset derived from DeepSeek-Prover-V1. Further refinement is achieved through reinforcement learning from proof assistant feedback (RLPAF). Beyond the single-pass whole-proof generation approach of DeepSeek-Prover-V1, we propose RMaxTS, a variant of Monte-Carlo tree search that employs an intrinsic-reward-driven exploration strategy to generate diverse proof paths. DeepSeek-Prover-V1.5 demonstrates significant improvements over DeepSeek-Prover-V1, achieving new state-of-the-art results on the test set of the high school level miniF2F benchmark (63.5%) and the undergraduate level ProofNet benchmark (25.3%).