DeeR-VLA: Dynamic Inference of Multimodal Large Language Models for Efficient Robot Execution
Yang Yue, Yulin Wang, Bingyi Kang, Yizeng Han, Shenzhi Wang, Shiji Song, Jiashi Feng, Gao Huang
2024-11-06
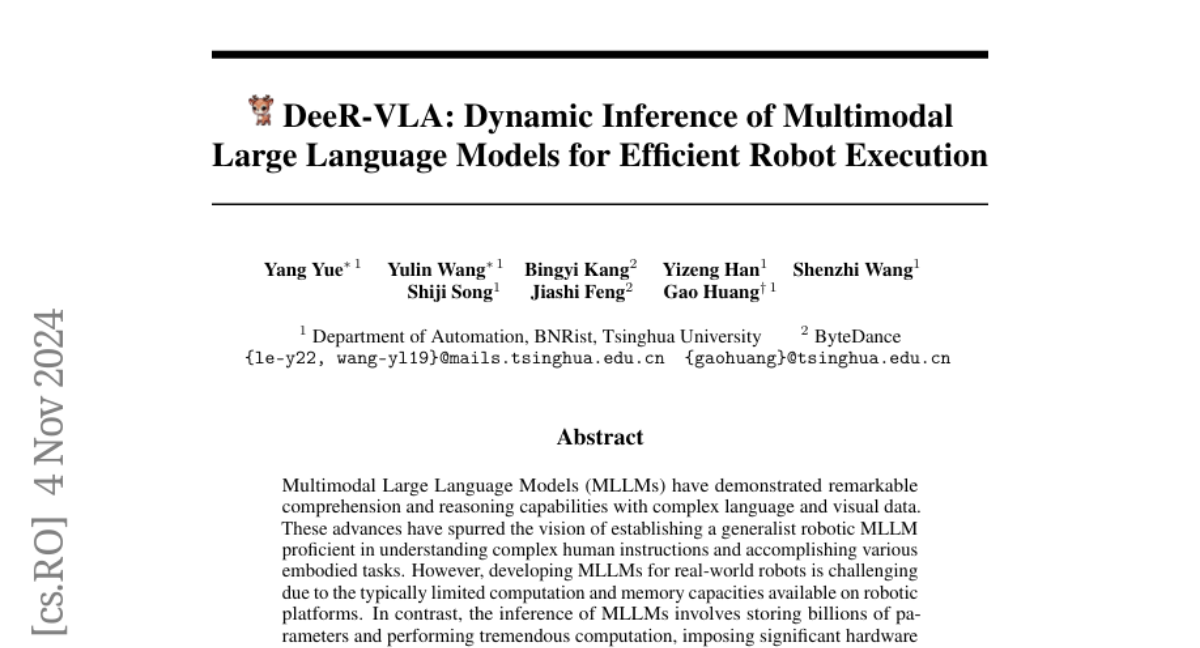
Summary
This paper introduces DeeR-VLA, a new system that helps robots use large language models more efficiently by adjusting how much of the model they use based on the task at hand.
What's the problem?
Robots that use large language models (LLMs) often struggle because these models require a lot of computing power and memory, which can be limited in real-world robotic systems. This makes it difficult for robots to perform tasks quickly and effectively.
What's the solution?
The authors developed a method called Dynamic Early-Exit Framework (DeeR) that allows robots to only use the necessary parts of the LLM based on the complexity of the task. This means that if a task is simple, the robot can stop processing once it has enough information, saving time and resources. They also created algorithms to determine when to stop processing to keep costs low while still performing well.
Why it matters?
This research is important because it makes robotic systems smarter and more efficient. By reducing the computational load, robots can work faster and use less energy, making them more practical for everyday tasks. This could lead to better applications in areas like manufacturing, healthcare, and service industries.
Abstract
MLLMs have demonstrated remarkable comprehension and reasoning capabilities with complex language and visual data. These advances have spurred the vision of establishing a generalist robotic MLLM proficient in understanding complex human instructions and accomplishing various embodied tasks. However, developing MLLMs for real-world robots is challenging due to the typically limited computation and memory capacities available on robotic platforms. In contrast, the inference of MLLMs involves storing billions of parameters and performing tremendous computation, imposing significant hardware demands. In our paper, we propose a Dynamic Early-Exit Framework for Robotic Vision-Language-Action Model (DeeR-VLA, or simply DeeR) that automatically adjusts the size of the activated MLLM based on each situation at hand. The approach leverages a multi-exit architecture in MLLMs, which allows the model to terminate processing once a proper size of the model has been activated for a specific situation, thus avoiding further redundant computation. Additionally, we develop novel algorithms that establish early-termination criteria for DeeR, conditioned on predefined demands such as average computational cost (i.e., power consumption), as well as peak computational consumption (i.e., latency) and GPU memory usage. These enhancements ensure that DeeR operates efficiently under varying resource constraints while maintaining competitive performance. On the CALVIN robot manipulation benchmark, DeeR demonstrates significant reductions in computational costs of LLM by 5.2-6.5x and GPU memory of LLM by 2-6x without compromising performance. Code and checkpoints are available at https://github.com/yueyang130/DeeR-VLA.