Degradation-Guided One-Step Image Super-Resolution with Diffusion Priors
Aiping Zhang, Zongsheng Yue, Renjing Pei, Wenqi Ren, Xiaochun Cao
2024-09-26
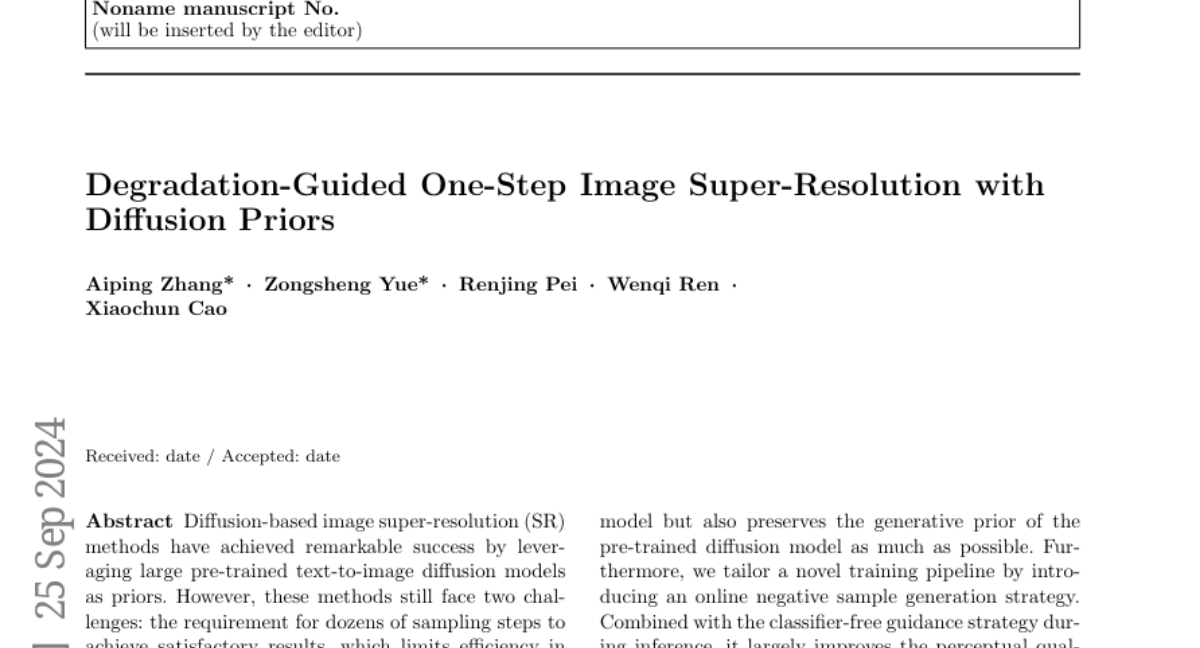
Summary
This paper introduces a new method for enhancing image quality called Degradation-Guided One-Step Image Super-Resolution. It aims to improve the process of turning low-resolution images into high-resolution ones by using advanced techniques that make it faster and more efficient.
What's the problem?
Traditional methods for improving image resolution often require many steps to achieve good results, which can be slow and inefficient. Additionally, these methods usually ignore important information about how the original low-resolution images were degraded, which is crucial for accurately restoring them. This leads to challenges in producing high-quality images quickly.
What's the solution?
The researchers developed a new one-step super-resolution model that uses a technique called Low-Rank Adaptation (LoRA) to adjust the model based on pre-estimated degradation information from low-resolution images. This allows the model to generate high-quality images in a single step instead of requiring multiple sampling steps. They also introduced a novel training method that helps improve the quality of the generated images by using negative samples during training, which enhances the overall perceptual quality of the results.
Why it matters?
This research is important because it significantly improves the efficiency of image super-resolution techniques, making it faster to produce high-quality images from low-resolution sources. This advancement can have practical applications in various fields, such as photography, video production, and medical imaging, where clear and detailed images are essential.
Abstract
Diffusion-based image super-resolution (SR) methods have achieved remarkable success by leveraging large pre-trained text-to-image diffusion models as priors. However, these methods still face two challenges: the requirement for dozens of sampling steps to achieve satisfactory results, which limits efficiency in real scenarios, and the neglect of degradation models, which are critical auxiliary information in solving the SR problem. In this work, we introduced a novel one-step SR model, which significantly addresses the efficiency issue of diffusion-based SR methods. Unlike existing fine-tuning strategies, we designed a degradation-guided Low-Rank Adaptation (LoRA) module specifically for SR, which corrects the model parameters based on the pre-estimated degradation information from low-resolution images. This module not only facilitates a powerful data-dependent or degradation-dependent SR model but also preserves the generative prior of the pre-trained diffusion model as much as possible. Furthermore, we tailor a novel training pipeline by introducing an online negative sample generation strategy. Combined with the classifier-free guidance strategy during inference, it largely improves the perceptual quality of the super-resolution results. Extensive experiments have demonstrated the superior efficiency and effectiveness of the proposed model compared to recent state-of-the-art methods.